A Backpropagation Approach for Distributed Resource Allocation
HAL (Le Centre pour la Communication Scientifique Directe)(2021)
摘要
Network resource allocation through Network Utility Maximization (NUM) is one of the fundamental problems in the realm of networked systems. In the NUM framework, the network comprises a set of nodes each of which is associated with a utility function, and the goal is to distribute resources across nodes so as to maximize the sum of the nodes utilities. In this paper, we propose a novel backpropagation approach for distributed resource allocation. e internal ow of resources among nodes is governed by the network dynamics, assumed to be captured through a directed acyclic graph (DAG). Control is exercised as an external injection of limited resources at some nodes, where the goal is to determine the optimal amount of resources to be injected at nodes, under the NUM framework. To that aim, we present a novel forward-backward algorithm, inspired by neural network training, wherein ows of resources are transferred during the forward step, and gradients are backpropagated at the backward step. Based on such gradients, the controls are adjusted, considering two variations of the algorithm under synchronous and asynchronous se ings. e proposed algorithms are distributed, in the sense that information in transferred only between neighboring nodes in the network. In addition, they are suitable for continued operation, so that the optimum resource allocation is tracked as conditions gradually change. We formally establish convergence of the proposed algorithms, and numerically compare the speed of convergence under the asynchronous se ing against its synchronous counterpart. Together, our results advance the state-of-the-art in the realm of NUM under nonlinear constraints, indicating how to leverage a backpropagation approach for that matter.
更多查看译文
关键词
distributed resource allocation,resource allocation,backpropagation approach
AI 理解论文
溯源树
样例
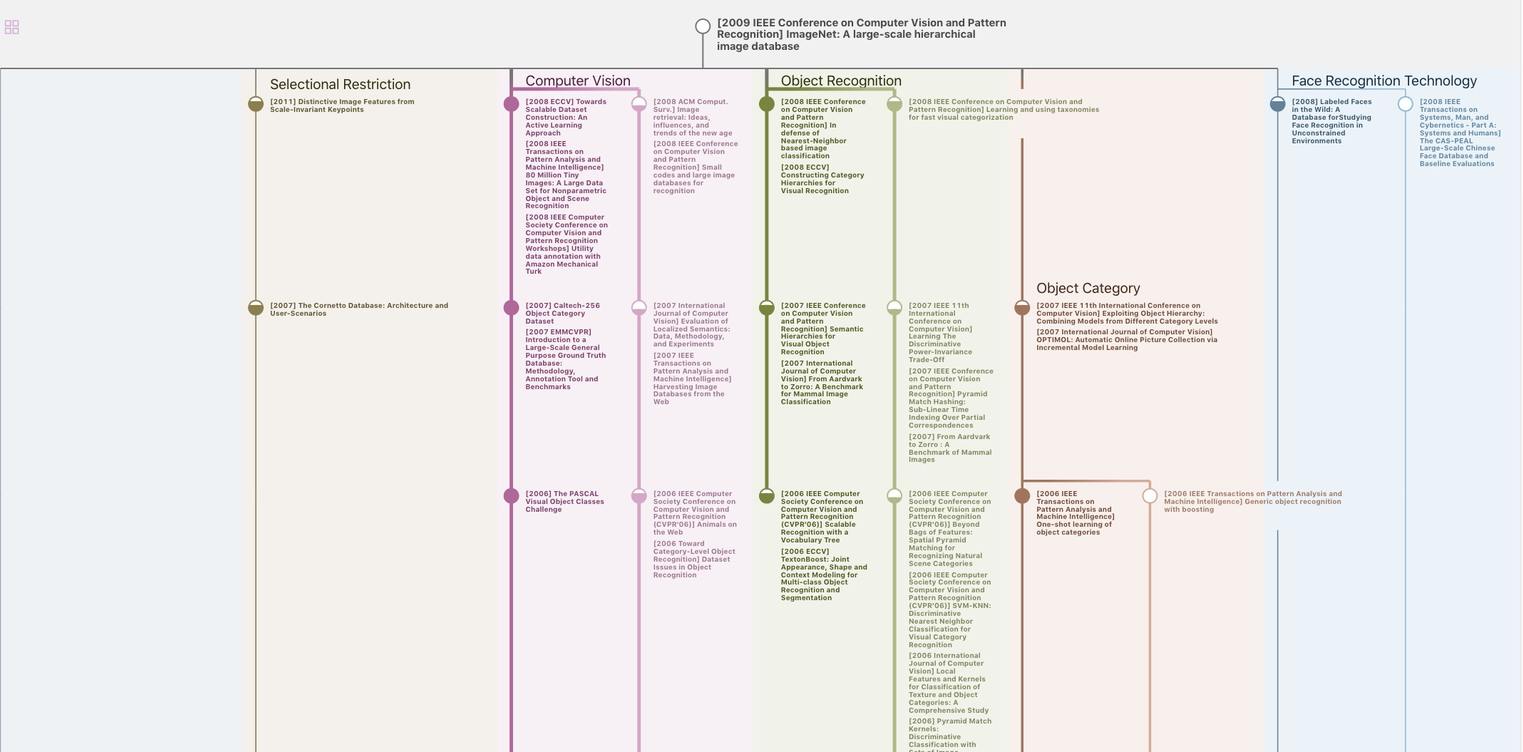
生成溯源树,研究论文发展脉络
Chat Paper
正在生成论文摘要