Differentially Private Methods for Managing Model Uncertainty in Linear Regression Models
JOURNAL OF MACHINE LEARNING RESEARCH(2024)
Abstract
In this work, we propose differentially private methods for hypothesis testing, model averaging, and model selection for normal linear models. We propose Bayesian methods based on mixtures of g-priors and non-Bayesian methods based on likelihood-ratio statistics and information criteria. The procedures are asymptotically consistent and straightforward to implement with existing software. We focus on practical issues such as adjusting critical values so that hypothesis tests have adequate type I error rates and quantifying the uncertainty introduced by the privacy-ensuring mechanisms.
MoreTranslated text
Key words
Confidential Data,Regression,Bayesian Methods,Information Criteria
AI Read Science
Must-Reading Tree
Example
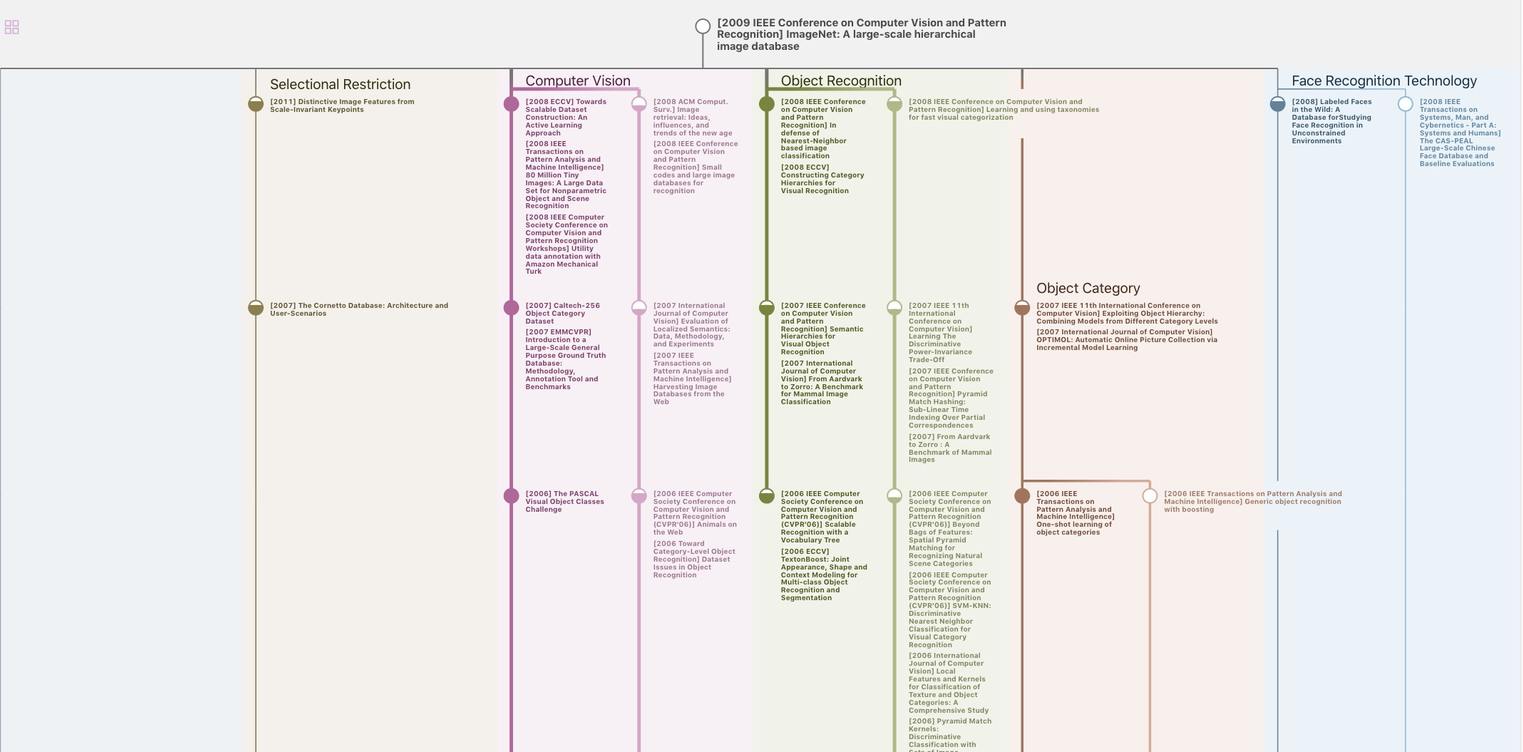
Generate MRT to find the research sequence of this paper
Chat Paper
Summary is being generated by the instructions you defined