Motif-based phosphoproteome clustering improves modeling and interpretation
bioRxiv (Cold Spring Harbor Laboratory)(2021)
Abstract
Abstract Cell signaling is orchestrated in part through a network of protein kinases and phosphatases. Dysregulation of kinase signaling is widespread in diseases such as cancer and is readily targetable through inhibitors of kinase enzymatic activity. Mass spectrometry-based analysis of kinase signaling can provide a global view of kinase signaling regulation but making sense of these data is complicated by its stochastic coverage of the proteome, measurement of substrates rather than kinase signaling itself, and the scale of the data collected. Here, we implement a dual data and motif clustering strategy (DDMC) that simultaneously clusters substrate peptides into similarly regulated groups based on their variation within an experiment and their sequence profile. We show that this can help to identify putative upstream kinases and supply more robust clustering. We apply this clustering to large-scale clinical proteomic profiling of lung cancer and identify conserved proteomic signatures of tumorigenicity, genetic mutations, and tumor immune infiltration. We propose that DDMC provides a general and flexible clustering strategy for the analysis of phosphoproteomic data. One-sentence Summary DDMC is a general and flexible strategy for phosphoproteomic analysis by clustering phosphopeptides using both their phosphorylation abundance and sequence motifs.
MoreTranslated text
Key words
phosphoproteome clustering,motif-based
AI Read Science
Must-Reading Tree
Example
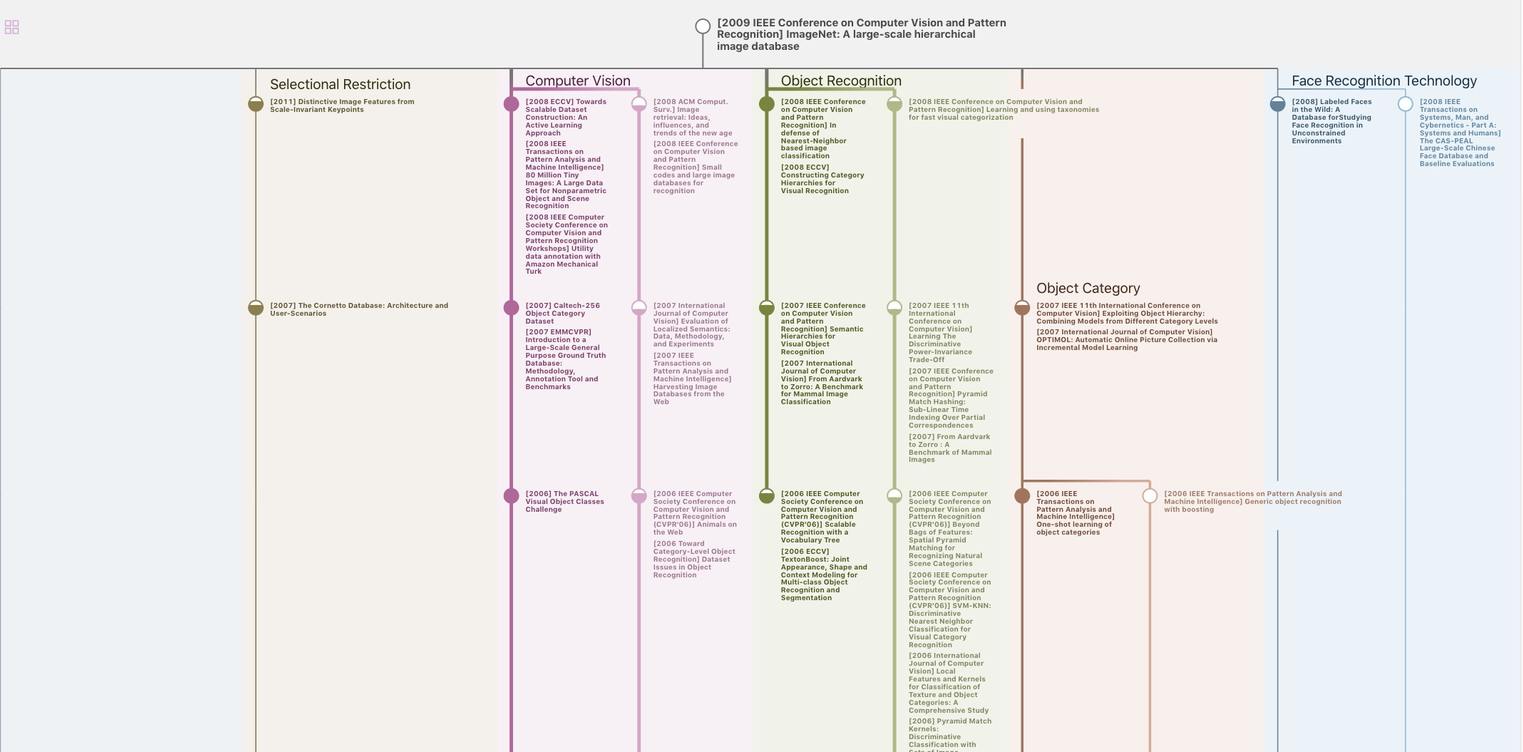
Generate MRT to find the research sequence of this paper
Chat Paper
Summary is being generated by the instructions you defined