Towards glass-box CNNs.
arXiv (Cornell University)(2021)
摘要
Convolution neural networks (CNNs) are brain-inspired architectures popular for their ability to train and relearn visually complex tasks. It is incremental and scalable; however, CNN is mostly treated as black-box and involves multiple trial & error runs. We observe that CNN constructs powerful internal representations that help achieve state-of-the-art performance. Here we propose three layer glass-box (analytical) CNN for two-class image classifcation problems. First is a representation layer that encompasses both the class information (group invariant) and symmetric transformations (group equivariant) of input images. It is then passed through dimension reduction layer (PCA). Finally the compact yet complete representation is provided to a classifer. Analytical machine learning classifers and multilayer perceptrons are used to assess sensitivity. Proposed glass-box CNN is compared with equivariance of AlexNet (CNN) internal representation for better understanding and dissemination of results. In future, we would like to construct glass-box CNN for multiclass visually complex tasks.
更多查看译文
关键词
glass-box
AI 理解论文
溯源树
样例
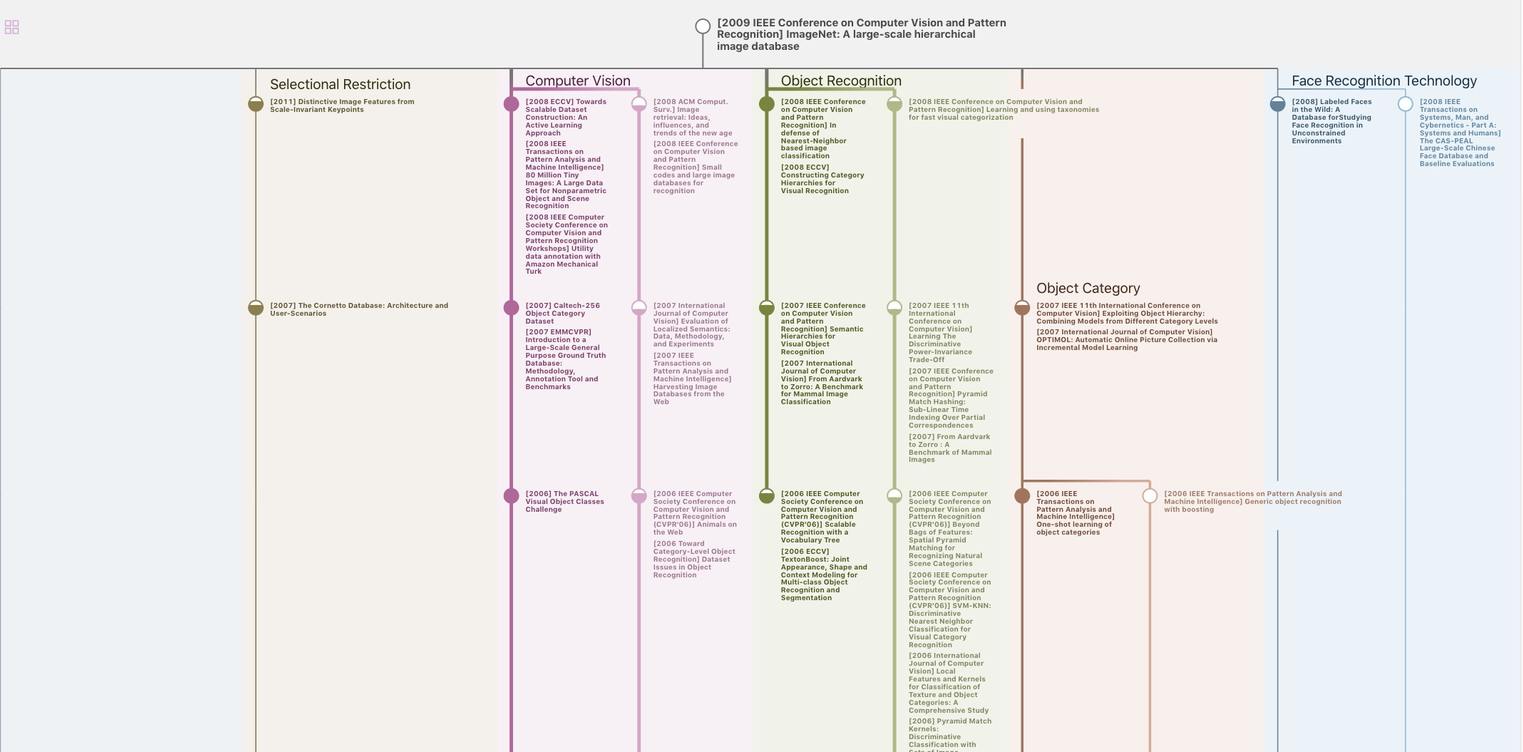
生成溯源树,研究论文发展脉络
Chat Paper
正在生成论文摘要