DEBOSH: Deep Bayesian Shape Optimization
arXiv (Cornell University)(2021)
摘要
Graph Neural Networks (GNNs) can predict the performance of an industrial design quickly and accurately and be used to optimize its shape effectively. However, to fully explore the shape space, one must often consider shapes deviating significantly from the training set. For these, GNN predictions become unreliable, something that is often ignored. For optimization techniques relying on Gaussian Processes, Bayesian Optimization (BO) addresses this issue by exploiting their ability to assess their own accuracy. Unfortunately, this is harder to do when using neural networks because standard approaches to estimating their uncertainty can entail high computational loads and reduced model accuracy. Hence, we propose a novel uncertainty-based method tailored to shape optimization. It enables effective BO and increases the quality of the resulting shapes beyond that of state-of-the-art approaches.
更多查看译文
关键词
shape,optimization,debosh
AI 理解论文
溯源树
样例
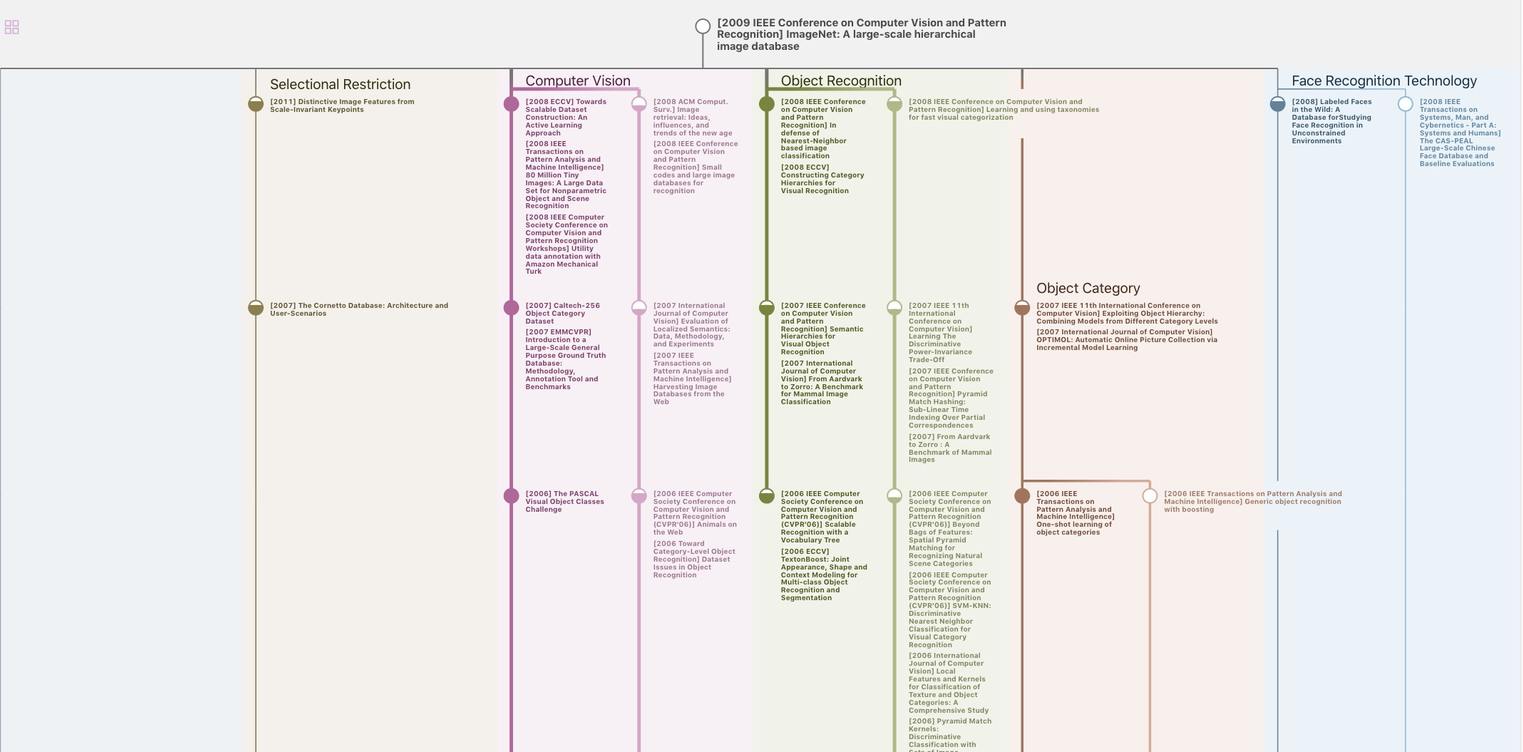
生成溯源树,研究论文发展脉络
Chat Paper
正在生成论文摘要