Rank-1 CNN for mental workload classification from EEG
HAL (Le Centre pour la Communication Scientifique Directe)(2021)
Abstract
Brain-computer interfaces (BCIs) can be separated into two main types: active and passive BCIs (Clerc et al. 2016). A BCI can be qualified of passive when the system uses signals involuntarily generated by the user. More specifically, this type of BCI is often used with the aim to assess the mental workload of users performing various task with different levels of mental demand, especially with electroencephalography (EEG) (Wang et al. 2015, Appriou et al. 2018, Shalchy et al. 2020). In most cases those systems are built with a classifier that classifies brain signals into different categories. This relies on having collected labelled data beforehand. However, those systems are often developed in laboratory settings, where both the train and test set have known labels. The Grand Challenge: Passive BCI Hackathon organised for the Neuroergonomics 2021 conference enables to challenge researchers with a real-life scenario of a passive BCI: classifying data from unseen sessions, with labels concealed for them, preventing any kind of fine tuning on the test set. The dataset provided for this challenge (Hinss et al. 2021) was composed of EEG recordings of 15 participants performing in 3 distinct sessions the Multi-Attribute Task Battery-II (MATB-II) developed by the NASA. Each session is decomposed in blocks of different difficulties: easy, medium and difficult. The data provided consists in epochs of 2 seconds (with a sampling frequency of 250 Hz) from those blocks for a total 447 epochs for each session and each participant. Difficulty labels were provided only for the 2 first sessions.
MoreTranslated text
Key words
mental workload classification,eeg
AI Read Science
Must-Reading Tree
Example
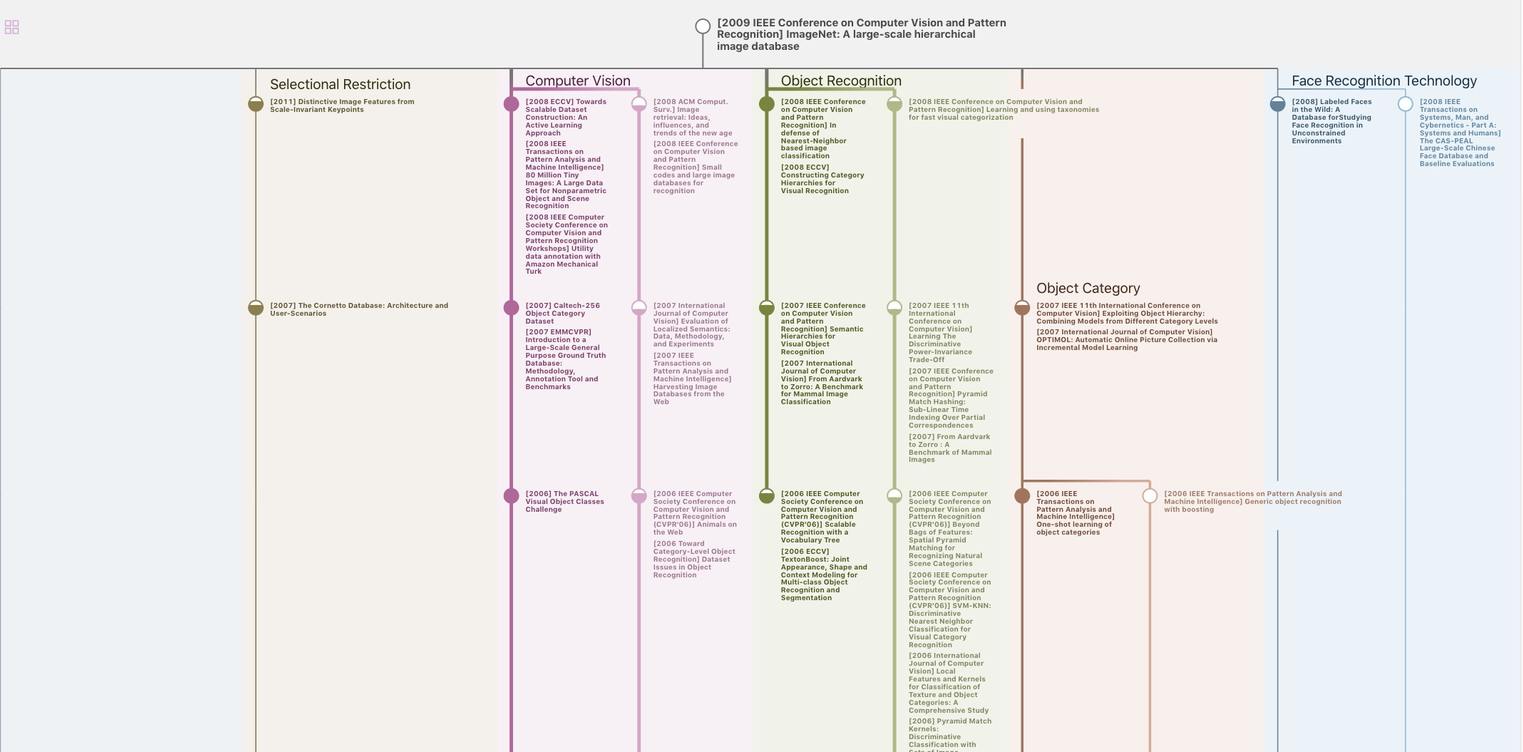
Generate MRT to find the research sequence of this paper
Chat Paper
Summary is being generated by the instructions you defined