Many-Configuration Markov-Chain Monte Carlo
arXiv (Cornell University)(2021)
摘要
We propose a minimal generalization of the celebrated Markov-Chain Monte Carlo algorithm which allows for an arbitrary number of configurations to be visited at every Monte Carlo step. This is advantageous when a parallel computing machine is available, or when many biased configurations can be evaluated at little additional computational cost. As an example of the former case, we report a significant reduction of the thermalization time for the paradigmatic Sherrington-Kirkpatrick spin-glass model. For the latter case, we show that, by leveraging on the exponential number of biased configurations automatically computed by Diagrammatic Monte Carlo, we can speed up computations in the Fermi-Hubbard model by two orders of magnitude.
更多查看译文
关键词
many-configuration,markov-chain
AI 理解论文
溯源树
样例
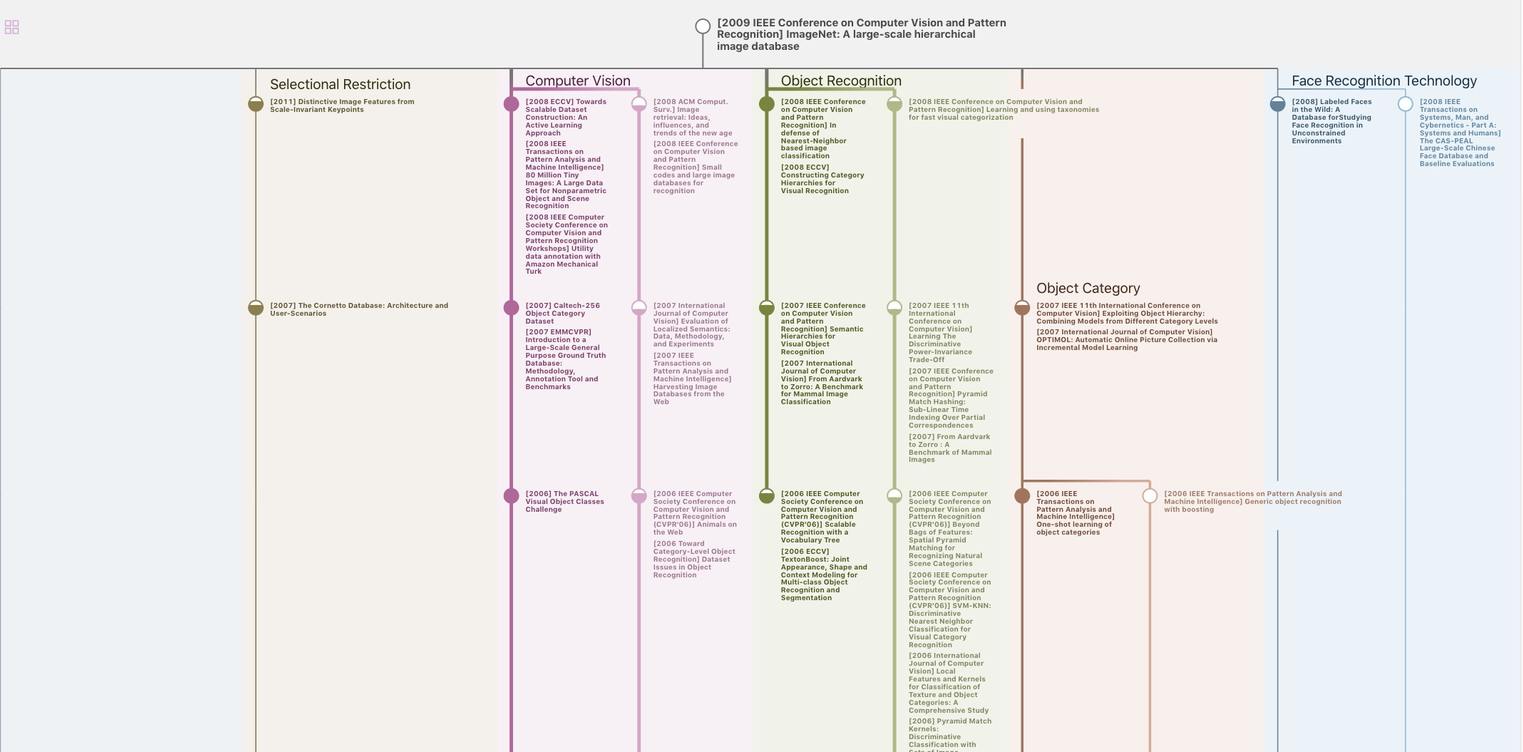
生成溯源树,研究论文发展脉络
Chat Paper
正在生成论文摘要