Selection of the best hybrid spectral similarity measure for characterising marine oil spills from multi-platform hyperspectral datasets
INTERNATIONAL JOURNAL OF COMPUTATIONAL SCIENCE AND ENGINEERING(2023)
摘要
Marine oil pollution causes major economic crises in major industrial sectors like fishing, shipping and tourism. It affects marine life and human even decades after spillage necessitating very quick detection and remediation. Generally, oils are exceedingly difficult to identify from high-resolution images as oil slicks and sea water possess identical spectral characteristics. Therefore a cohesive and synergistic classification method called hybrid spectral similarity measures (HSSM) that discerns the data-rich constituents of hyperspectral images (HSI) is recommended in this paper to classify oil spills. Oil spill HSI procured from spaceborne [earth observation (EO-1) Hyperion] and airborne [airborne visible/infrared imaging spectrometer (AVIRIS)] platforms are employed to discriminate different marine spectral classes. The statistical parameters like overall accuracy (OA), Kappa, ROC/PR curve, AUC/PRAUC, weighted Youden index (Jw), F1score and Noise analysis have identified spectral information divergence-chi square distance (SID-CHI) as the best HSSM promulgating its multi-class, multi-sensor, and multi-platform oil spill classification capability.
更多查看译文
关键词
hybrid spectral similarity measure,HSSM,hyperspectral image,HSI,ROC curve,weighted Youden index,F1 score,optimal cut-off value,OCV,signal to noise ratio,SNR,Hyperion,AVIRIS,oil spill
AI 理解论文
溯源树
样例
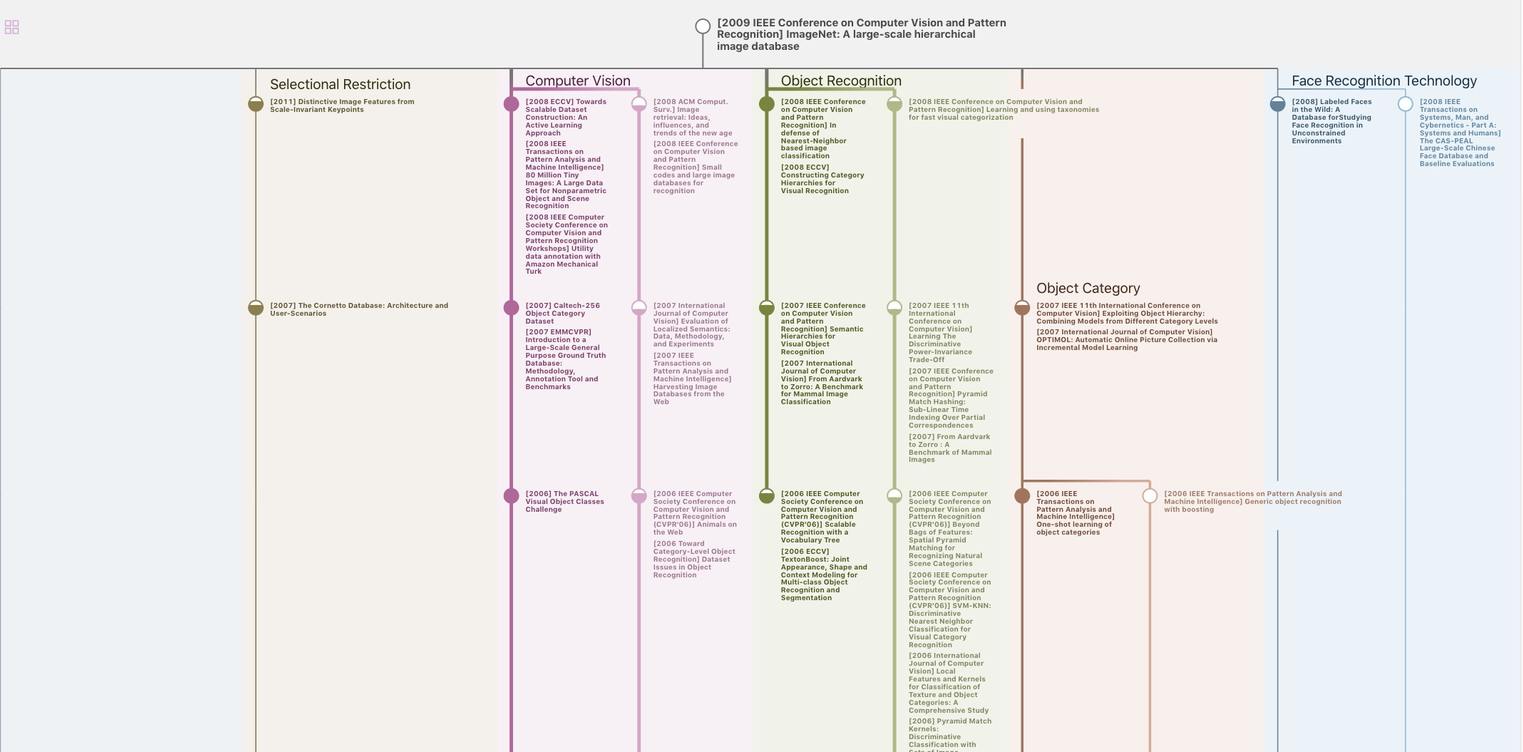
生成溯源树,研究论文发展脉络
Chat Paper
正在生成论文摘要