Integrating Unsupervised Data Generation into Self-Supervised Neural Machine Translation for Low-Resource Languages
arXiv (Cornell University)(2021)
摘要
For most language combinations, parallel data is either scarce or simply unavailable. To address this, unsupervised machine translation (UMT) exploits large amounts of monolingual data by using synthetic data generation techniques such as back-translation and noising, while self-supervised NMT (SSNMT) identifies parallel sentences in smaller comparable data and trains on them. To date, the inclusion of UMT data generation techniques in SSNMT has not been investigated. We show that including UMT techniques into SSNMT significantly outperforms SSNMT and UMT on all tested language pairs, with improvements of up to +4.3 BLEU, +50.8 BLEU, +51.5 over SSNMT, statistical UMT and hybrid UMT, respectively, on Afrikaans to English. We further show that the combination of multilingual denoising autoencoding, SSNMT with backtranslation and bilingual finetuning enables us to learn machine translation even for distant language pairs for which only small amounts of monolingual data are available, e.g. yielding BLEU scores of 11.6 (English to Swahili).
更多查看译文
关键词
neural machine translation,unsupervised data generation,languages,self-supervised,low-resource
AI 理解论文
溯源树
样例
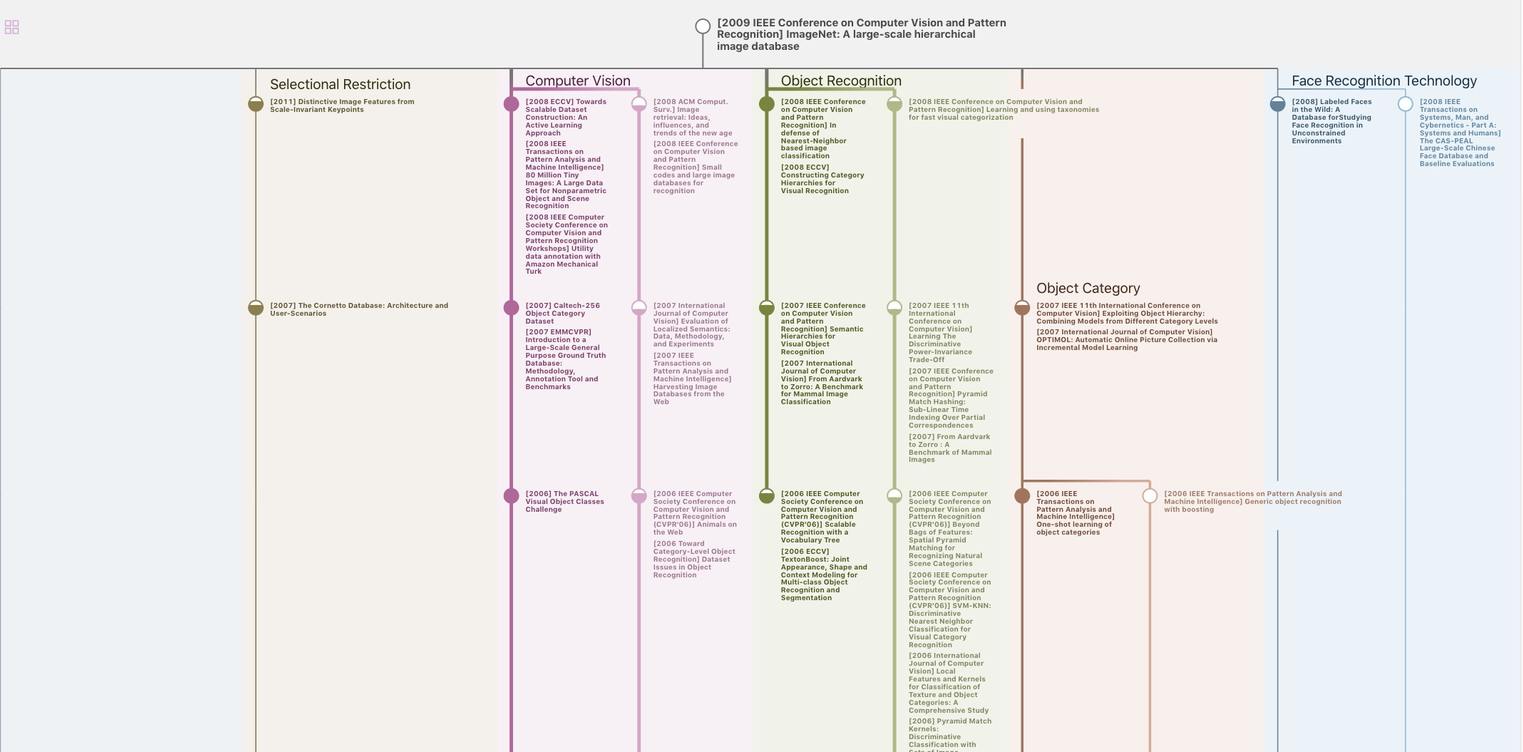
生成溯源树,研究论文发展脉络
Chat Paper
正在生成论文摘要