Non-iterative Methods in Inhomogeneous Background Inverse Scattering Imaging Problem Assisted by Swin Transformer Network
arxiv(2023)
摘要
A deep learning-assisted inversion method is proposed to solve the
inhomogeneous background imaging problem. Three non-iterative methods, namely
the distorted-Born (DB) major current coefficients method, the DB modified Born
approximation method, and the DB connection method, are introduced to address
the inhomogeneous background inverse scattering problem. These methods retain
the multiple scattering information by utilizing the major current obtained
through singular value decomposition of the Green's function and the scattered
field, without resourcing to optimization techniques. As a result, the proposed
methods offer improved reconstruction resolution and accuracy for unknown
objects embedded in inhomogeneous backgrounds, surpassing the backpropagation
scheme (BPS) and Born approximation (BA) method that disregard the multiple
scattering effect. To further enhance the resolution and accuracy of the
reconstruction, a Shifted-Window (Swin) transformer network is employed for
capturing super-resolution information in the images. The attention mechanism
incorporated in the shifted window facilitates global interactions between
objects, thereby enhancing the performance of the inhomogeneous background
imaging algorithm while reducing computational complexity. Moreover, an
adaptive training method is proposed to enhance the generalization ability of
the network. The effectiveness of the proposed methods is demonstrated through
both synthetic data and experimental data. Notably, super-resolution imaging is
achieved with quasi real-time speed, indicating promising application potential
for the proposed algorithms.
更多查看译文
AI 理解论文
溯源树
样例
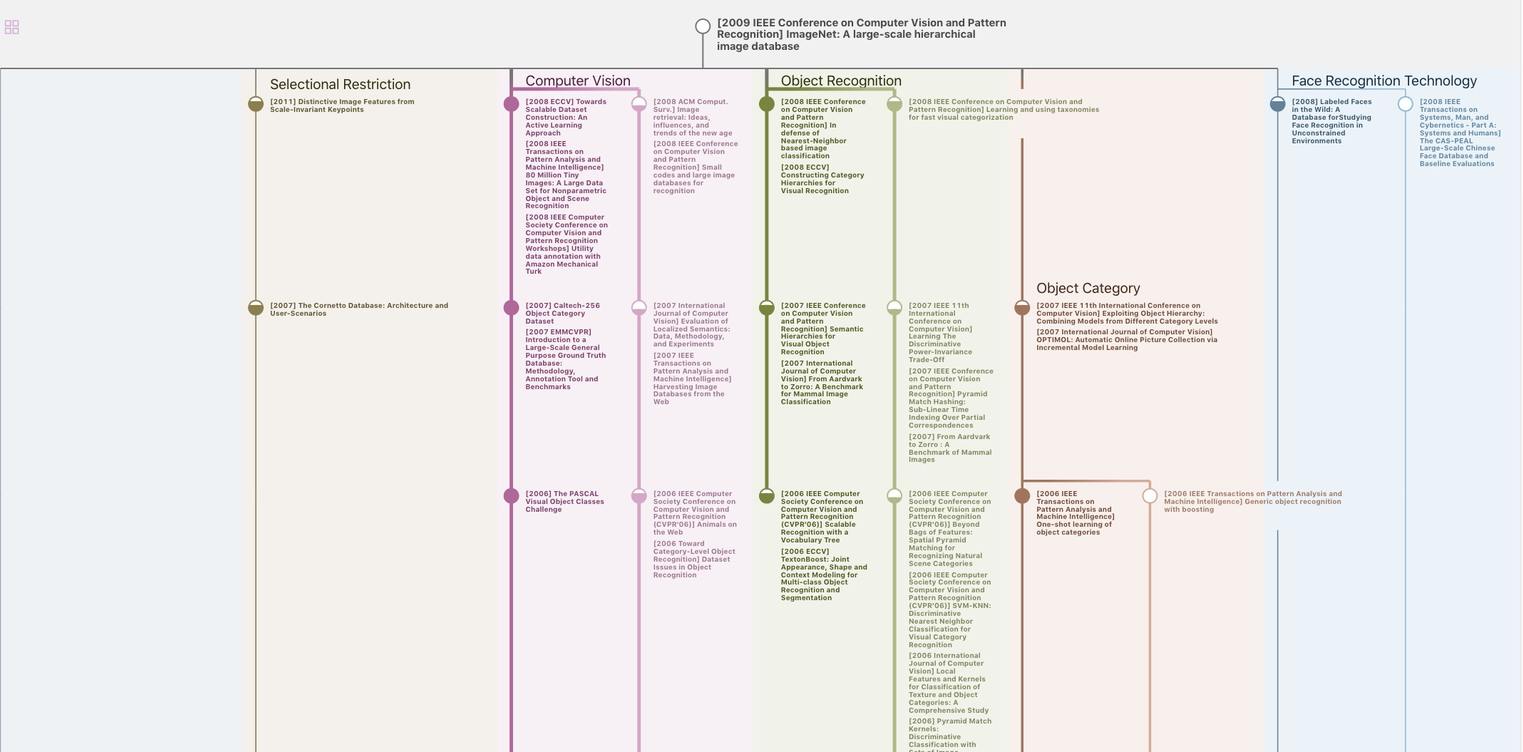
生成溯源树,研究论文发展脉络
Chat Paper
正在生成论文摘要