Federated Causality Learning with Explainable Adaptive Optimization
AAAI 2024(2024)
摘要
Discovering the causality from observational data is a crucial task in various scientific domains. With increasing awareness of privacy, data are not allowed to be exposed, and it is very hard to learn causal graphs from dispersed data, since these data may have different distributions. In this paper, we propose a federated causal discovery strategy (FedCausal) to learn the unified global causal graph from decentralized heterogeneous data. We design a global optimization formula to naturally aggregate the causal graphs from client data and constrain the acyclicity of the global graph without exposing local data. Unlike other federated causal learning algorithms, FedCausal unifies the local and global optimizations into a complete directed acyclic graph (DAG) learning process with a flexible optimization objective. We prove that this optimization objective has a high interpretability and can adaptively handle homogeneous and heterogeneous data. Experimental results on synthetic and real datasets show that FedCausal can effectively deal with non-independently and identically distributed (non-iid) data and has a superior performance.
更多查看译文
关键词
ML: Causal Learning,CSO: Constraint Optimization,DMKM: Graph Mining, Social Network Analysis & Community,ML: Distributed Machine Learning & Federated Learning
AI 理解论文
溯源树
样例
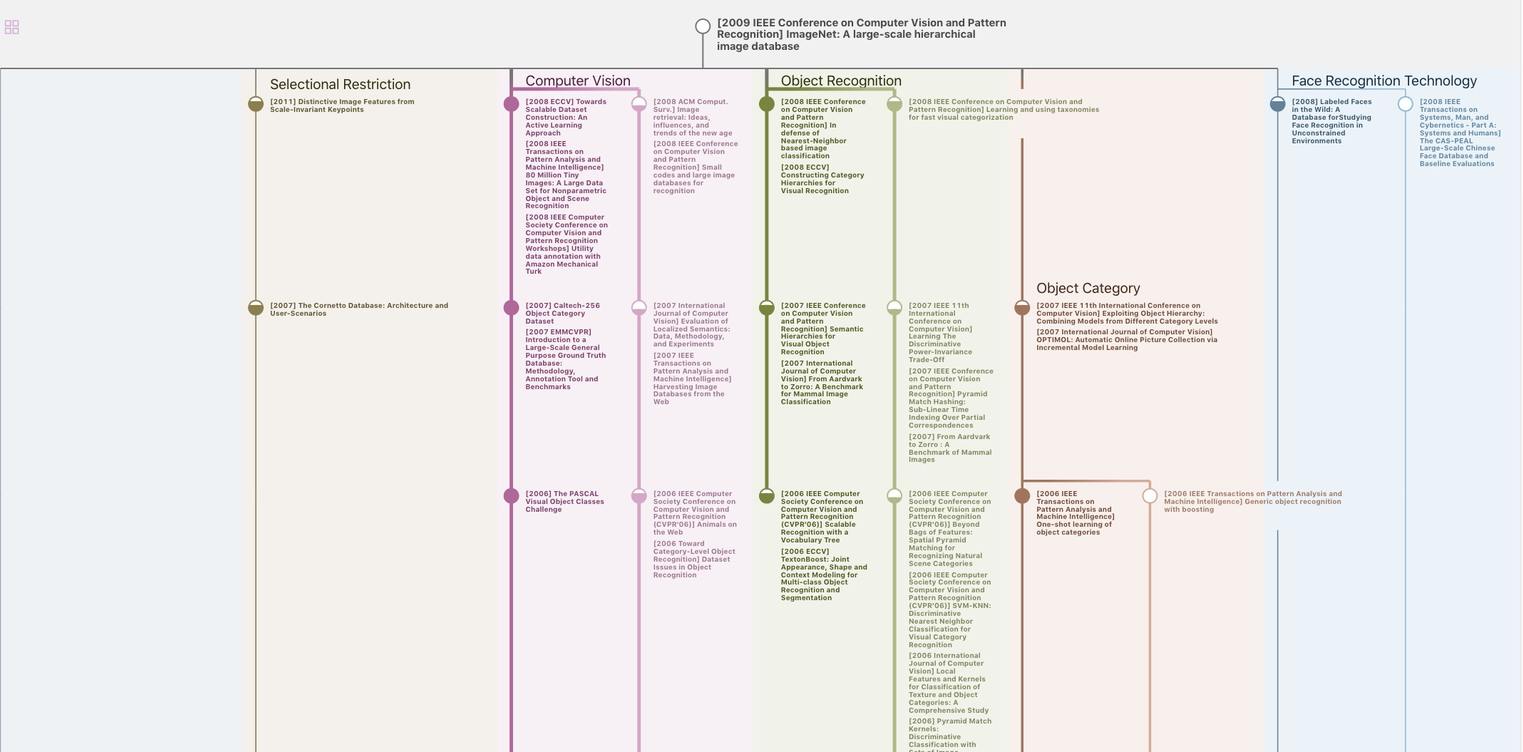
生成溯源树,研究论文发展脉络
Chat Paper
正在生成论文摘要