Convergence of Multi-Scale Reinforcement Q-Learning Algorithms for Mean Field Game and Control Problems
arxiv(2023)
摘要
We establish the convergence of the unified two-timescale Reinforcement
Learning (RL) algorithm presented in a previous work by Angiuli et al. This
algorithm provides solutions to Mean Field Game (MFG) or Mean Field Control
(MFC) problems depending on the ratio of two learning rates, one for the value
function and the other for the mean field term. Our proof of convergence
highlights the fact that in the case of MFC several mean field distributions
need to be updated and for this reason we present two separate algorithms, one
for MFG and one for MFC. We focus on a setting with finite state and action
spaces, discrete time and infinite horizon. The proofs of convergence rely on a
generalization of the two-timescale approach of Borkar. The accuracy of
approximation to the true solutions depends on the smoothing of the policies.
We provide a numerical example illustrating the convergence.
更多查看译文
AI 理解论文
溯源树
样例
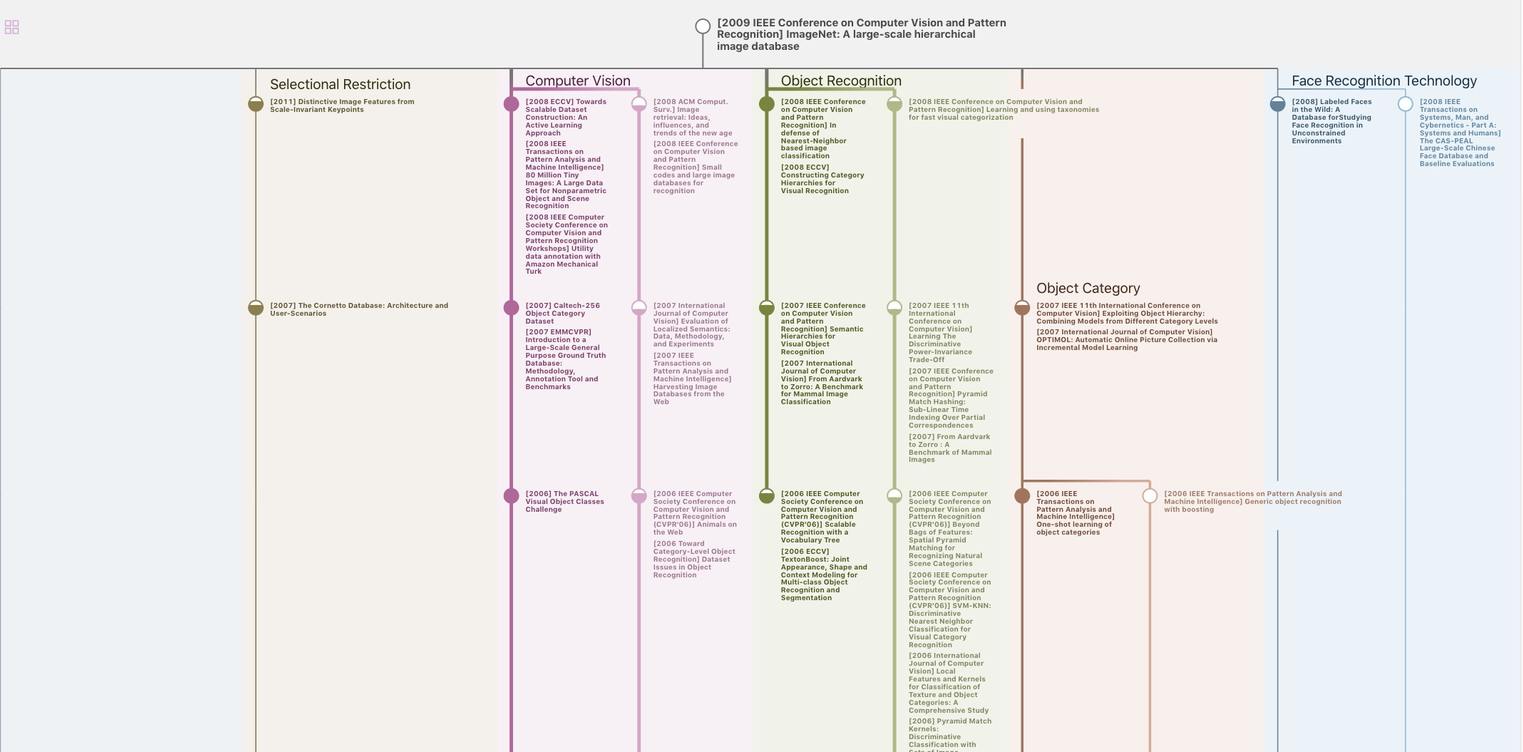
生成溯源树,研究论文发展脉络
Chat Paper
正在生成论文摘要