Targeted Attack on Deep RL-based Autonomous Driving with Learned Visual Patterns
arXiv (Cornell University)(2021)
摘要
Recent studies demonstrated the vulnerability of control policies learned through deep reinforcement learning against adversarial attacks, raising concerns about the application of such models to risk-sensitive tasks such as autonomous driving. Threat models for these demonstrations are limited to (1) targeted attacks through real-time manipulation of the agent's observation, and (2) untargeted attacks through manipulation of the physical environment. The former assumes full access to the agent's states/observations at all times, while the latter has no control over attack outcomes. This paper investigates the feasibility of targeted attacks through visually learned patterns placed on physical objects in the environment, a threat model that combines the practicality and effectiveness of the existing ones. Through analysis, we demonstrate that a pre-trained policy can be hijacked within a time window, e.g., performing an unintended self-parking, when an adversarial object is present. To enable the attack, we adopt an assumption that the dynamics of both the environment and the agent can be learned by the attacker. Lastly, we empirically show the effectiveness of the proposed attack on different driving scenarios, perform a location robustness test, and study the tradeoff between the attack strength and its effectiveness. Code is available at https://github.com/ASU-APG/Targeted-Physical-Adversarial-Attacks-on-AD
更多查看译文
关键词
learned visual
AI 理解论文
溯源树
样例
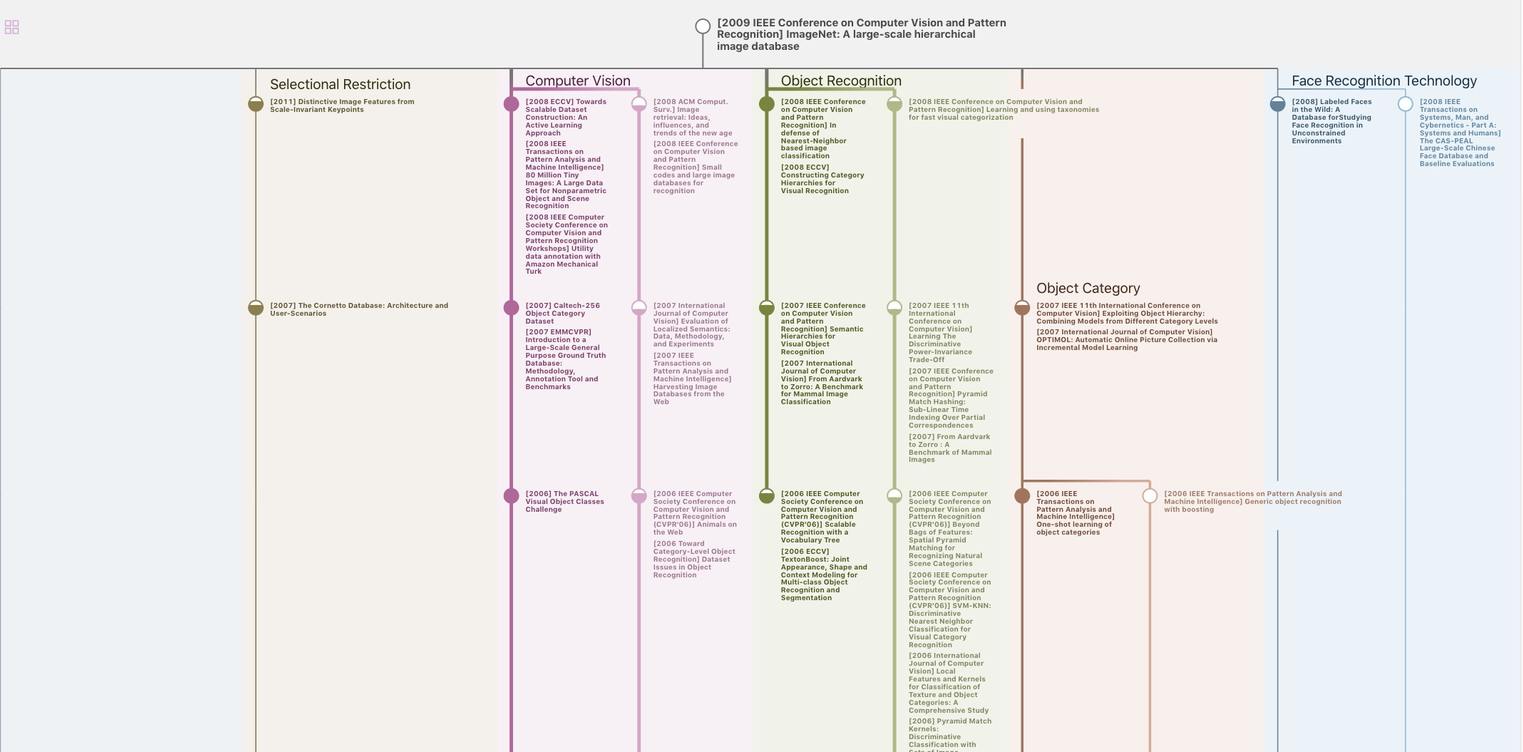
生成溯源树,研究论文发展脉络
Chat Paper
正在生成论文摘要