PowerGraph: Using neural networks and principal components to determine multivariate statistical power trade-offs
arXiv (Cornell University)(2021)
摘要
Statistical power estimation for studies with multiple model parameters is inherently a multivariate problem. Power for individual parameters of interest cannot be reliably estimated univariately since correlation and variance explained relative to one parameter will impact the power for another parameter, all usual univariate considerations being equal. Explicit solutions in such cases, especially for models with many parameters, are either impractical or impossible to solve, leaving researchers to the prevailing method of simulating power. However, the point estimates for a vector of model parameters are uncertain, and the impact of inaccuracy is unknown. In such cases, sensitivity analysis is recommended such that multiple combinations of possible observable parameter vectors are simulated to understand power trade-offs. A limitation to this approach is that it is computationally expensive to generate sufficient sensitivity combinations to accurately map the power trade-off function in increasingly high-dimensional spaces for the models that social scientists estimate. This paper explores the efficient estimation and graphing of statistical power for a study over varying model parameter combinations. We propose a simple and generalizable machine learning inspired solution to cut the computational cost to less than 10% of the brute force method while providing F1 scores above 90%. We further motivate the impact of transfer learning in learning power manifolds across varying distributions.
更多查看译文
关键词
principal components,powergraph,networks,multivariate,trade-offs
AI 理解论文
溯源树
样例
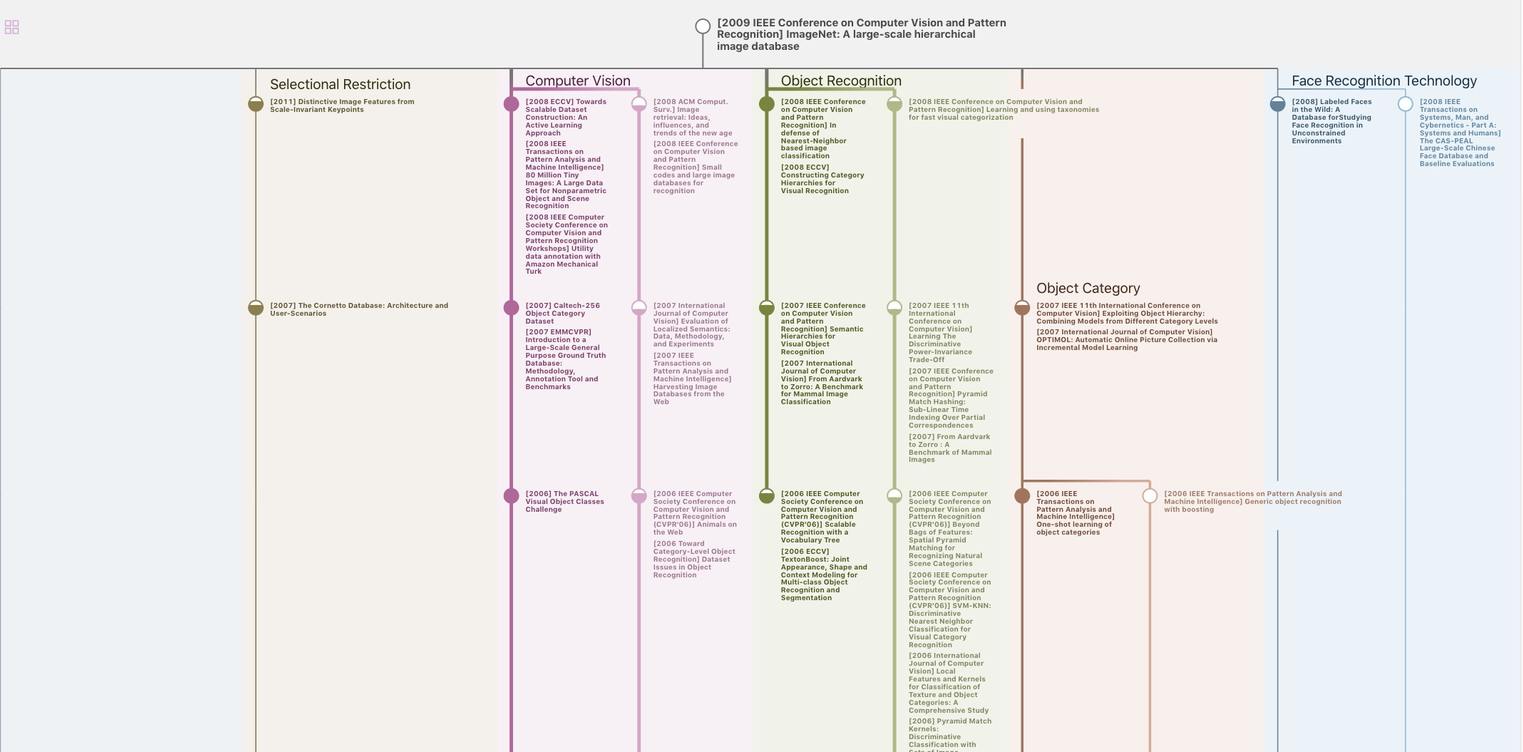
生成溯源树,研究论文发展脉络
Chat Paper
正在生成论文摘要