Realization of an ion trap quantum classifier
arXiv (Cornell University)(2021)
摘要
We report the realization of a versatile classifier based on the quantum mechanics of a single atom. The problem of classification has been extensively studied by the classical machine learning community, with plenty of proposed algorithms that have been refined over time. Quantum computation must necessarily develop quantum classifiers and benchmark them against their classical counterparts. It is not obvious how to make use of our increasing ability to precisely control and evolve a quantum state to solve this kind of problems, while there is only a limited number of strong theorems backing the quantum algorithms for classification. Here we show that both of these limitations can be successfully addressed by the implementation of a recently proposed data re-uploading algorithm in an ion trap based quantum processing unit. The quantum classifier is trained in two steps: first, the quantum circuit is fed with an optimal set of variational parameters found by classical simulation; then, the variational circuit is optimized by inspecting the parameter landscape with only the quantum processing unit. This second step provides a partial cancellation of the systematic errors inherent to the quantum device. The accuracy of our quantum supervised classifier is benchmarked on a variety of datasets, that imply, finding the separation of classes associated to regions in a plane in both binary and multi-class problems, as well as in higher-dimensional feature spaces. Our experiments show that a single-ion quantum classifier circuit made out of $k$ gates is as powerful as a neural network with one intermediate hidden layer of $k$ neurons.
更多查看译文
关键词
quantum,realization
AI 理解论文
溯源树
样例
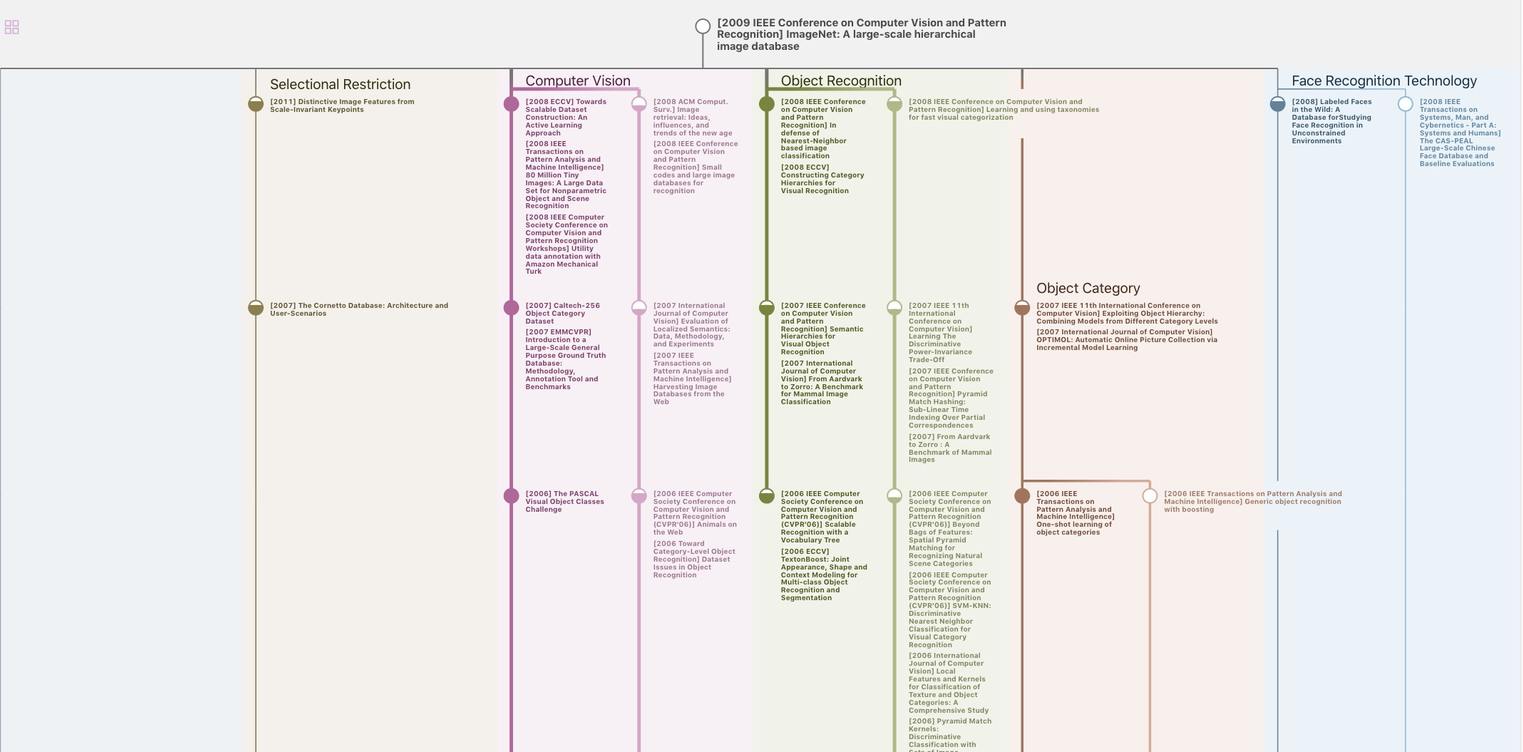
生成溯源树,研究论文发展脉络
Chat Paper
正在生成论文摘要