Structure-Preserving Learning Using Gaussian Processes and Variational Integrators
arXiv (Cornell University)(2021)
摘要
Gaussian process regression is often applied for learning unknown systems and specifying the uncertainty of the learned model. When using Gaussian process regression to learn unknown systems, a commonly considered approach consists of learning the residual dynamics after applying some standard discretization, which might however not be appropriate for the system at hand. Variational integrators are a less common yet promising approach to discretization, as they retain physical properties of the underlying system, such as energy conservation or satisfaction of explicit constraints. In this work, we propose the combination of a variational integrator for the nominal dynamics of a mechanical system and learning residual dynamics with Gaussian process regression. We extend our approach to systems with known kinematic constraints and provide formal bounds on the prediction uncertainty. The simulative evaluation of the proposed method shows desirable energy conservation properties in accordance with the theoretical results and demonstrates the capability of treating constrained dynamical systems.
更多查看译文
关键词
gaussian processes,variational
AI 理解论文
溯源树
样例
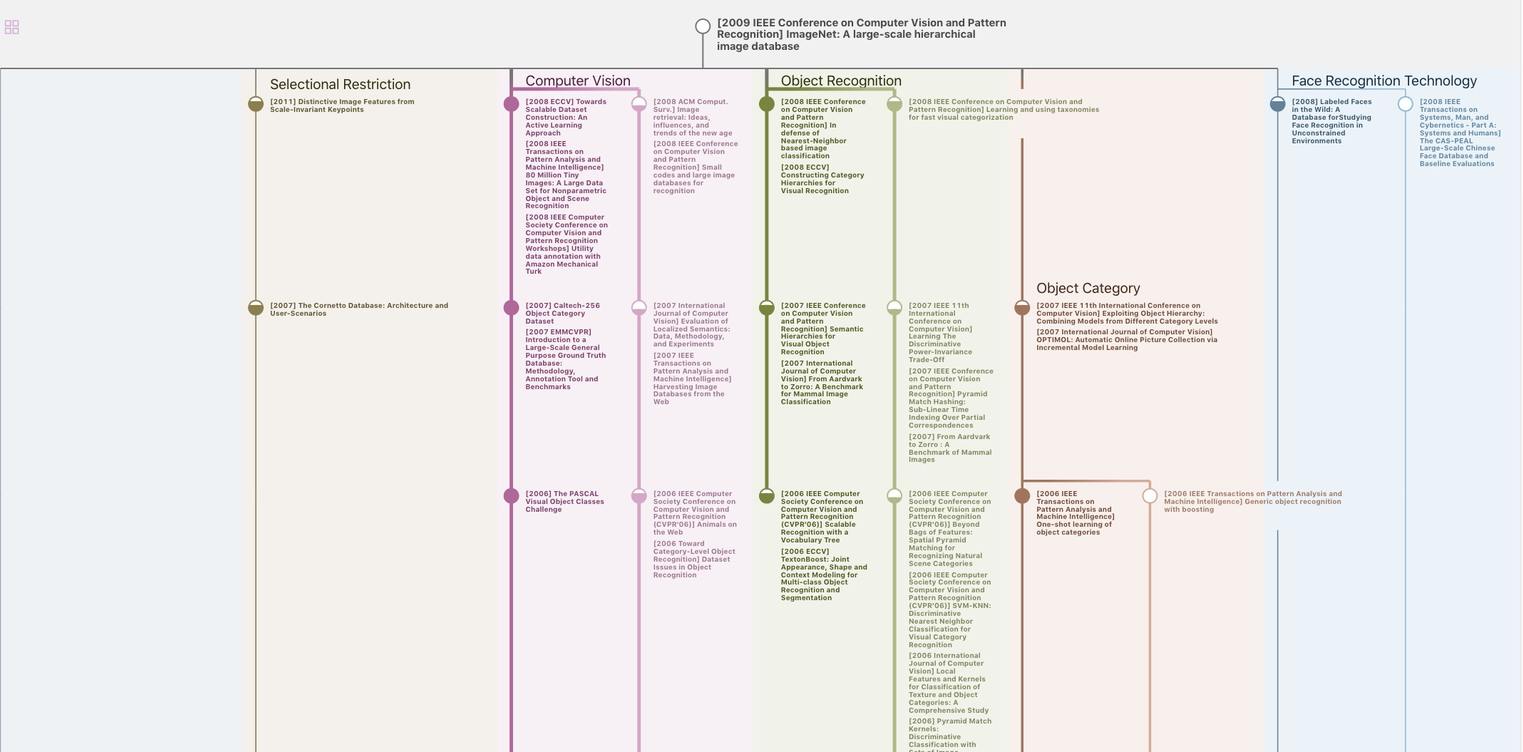
生成溯源树,研究论文发展脉络
Chat Paper
正在生成论文摘要