Can depth-adaptive BERT perform better on binary classification tasks
arXiv (Cornell University)(2021)
摘要
In light of the success of transferring language models into NLP tasks, we ask whether the full BERT model is always the best and does it exist a simple but effective method to find the winning ticket in state-of-the-art deep neural networks without complex calculations. We construct a series of BERT-based models with different size and compare their predictions on 8 binary classification tasks. The results show there truly exist smaller sub-networks performing better than the full model. Then we present a further study and propose a simple method to shrink BERT appropriately before fine-tuning. Some extended experiments indicate that our method could save time and storage overhead extraordinarily with little even no accuracy loss.
更多查看译文
关键词
classification,depth-adaptive
AI 理解论文
溯源树
样例
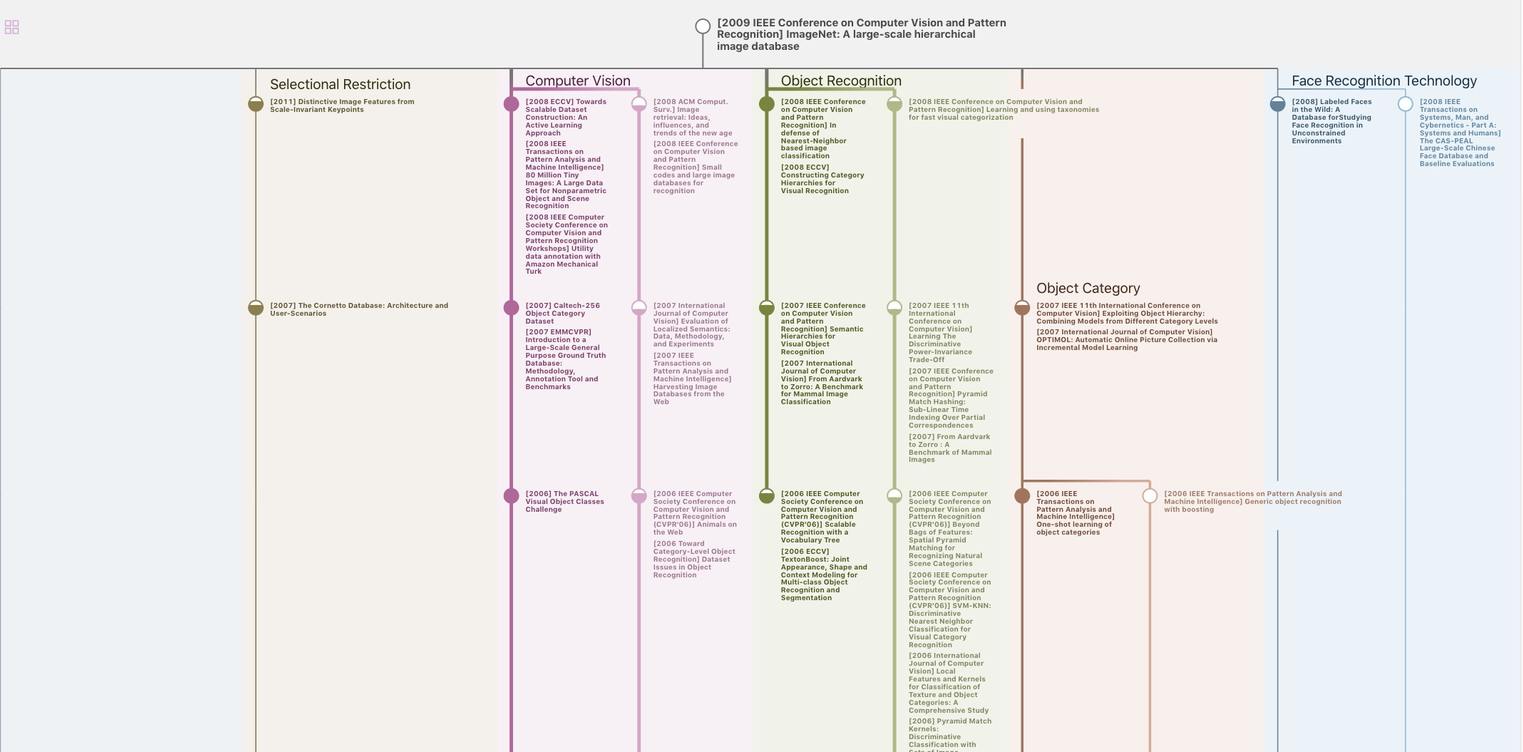
生成溯源树,研究论文发展脉络
Chat Paper
正在生成论文摘要