Layer-wise Pruning of Transformer Attention Heads for Efficient Language Modeling
arXiv (Cornell University)(2021)
摘要
While Transformer-based models have shown impressive language modeling performance, the large computation cost is often prohibitive for practical use. Attention head pruning, which removes unnecessary attention heads in the multihead attention, is a promising technique to solve this problem. However, it does not evenly reduce the overall load because the heavy feedforward module is not affected by head pruning. In this paper, we apply layer-wise attention head pruning on All-attention Transformer so that the entire computation and the number of parameters can be reduced proportionally to the number of pruned heads. While the architecture has the potential to fully utilize head pruning, we propose three training methods that are especially helpful to minimize performance degradation and stabilize the pruning process. Our pruned model shows consistently lower perplexity within a comparable parameter size than Transformer-XL on WikiText-103 language modeling benchmark.
更多查看译文
关键词
transformer attention heads,language,layer-wise
AI 理解论文
溯源树
样例
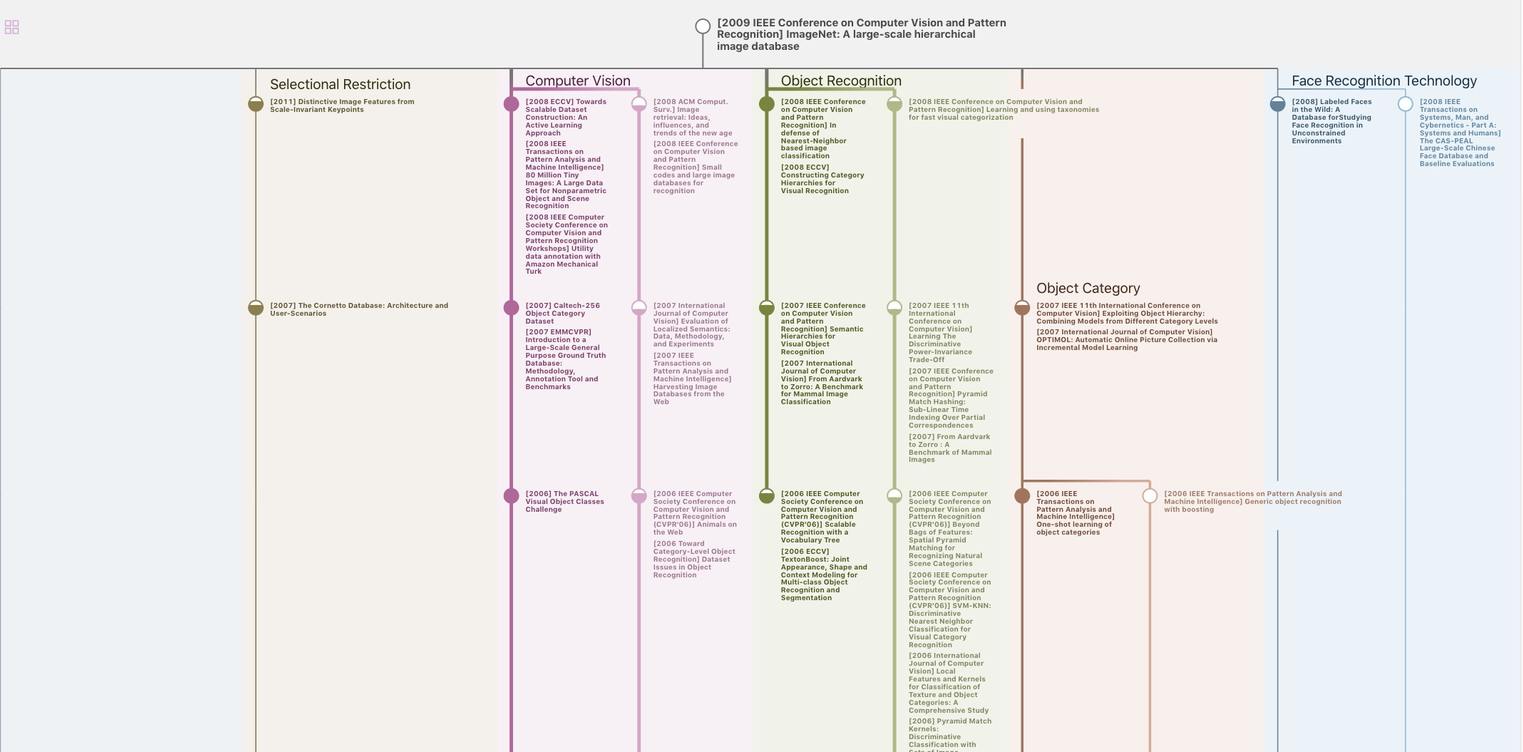
生成溯源树,研究论文发展脉络
Chat Paper
正在生成论文摘要