USPNet: unbiased organism-agnostic signal peptide predictor with deep protein language model
bioRxiv (Cold Spring Harbor Laboratory)(2021)
摘要
Abstract Signal peptide is a short peptide located in the N-terminus of proteins. It plays an important role in targeting and transferring transmembrane proteins and secreted proteins to correct positions. Compared with traditional experimental methods to identify and discover signal peptides, the computational methods are faster and more efficient, which are more practical for the analysis of thousands or even millions of protein sequences in reality, especially for the metagenomic data. Therefore, computational tools are recently proposed to classify signal peptides and predict cleavage site positions, but most of them disregard the extreme data imbalance problem in these tasks. In addition, almost all these methods rely on additional group information of proteins to boost their performances, which, however, may not always be available. To deal with these issues, in this paper, we present Unbiased Organism-agnostic Signal Peptide Network (USPNet), a signal peptide prediction and cleavage site prediction model based on deep protein language model. We propose to use label distribution-aware margin (LDAM) loss and evolutionary scale modeling (ESM) embedding to handle data imbalance and object-dependence problems. Extensive experimental results demonstrate that the proposed method significantly outperforms all the previous methods on the classification performance. Additional study on the simulated metagenomic data further indicates that our model is a more universal and robust tool without dependency on additional group information of proteins, with the Matthews correlation coefficient improved by up to 17.5%. The proposed method will be potentially useful to discover new signal peptides from the abundant metagenomic data.
更多查看译文
关键词
peptide,protein,deep,organism-agnostic
AI 理解论文
溯源树
样例
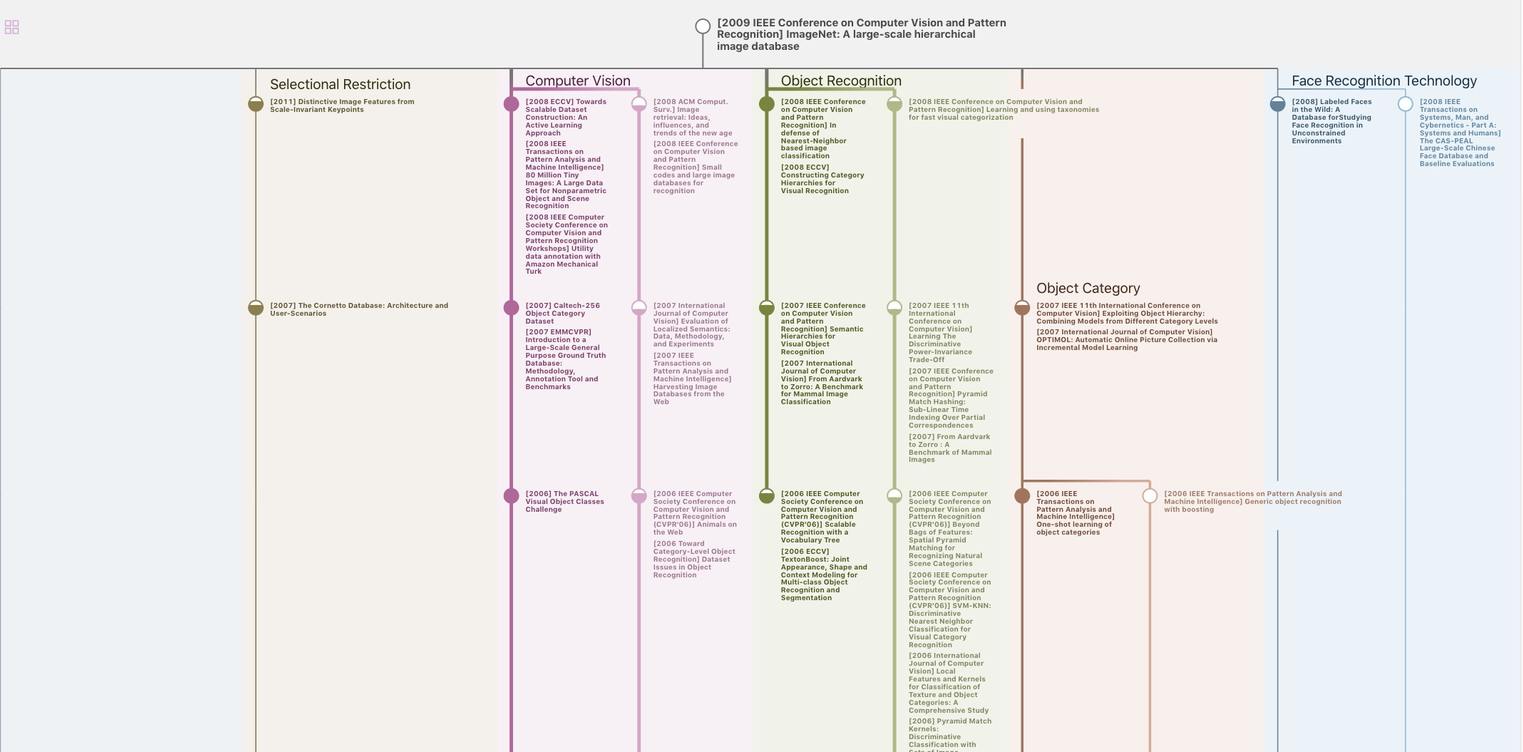
生成溯源树,研究论文发展脉络
Chat Paper
正在生成论文摘要