A novel perspective on the meaning of nestedness with conceptual and methodological solutions
bioRxiv (Cold Spring Harbor Laboratory)(2021)
摘要
ABSTRACT Nestedness is a common pattern in metacommunities and interaction networks, whose causes are still subject to investigation. Nestedness inference is challenging because, beyond calculating an index, we need to compare observed and expected values using null models. There are many different null models and the choice between them strongly affects test outcomes. Furthermore, there is no theoretical basis to guide this choice. Here, we propose a different look at the meaning of nestedness that improves our understanding of its causes and directly links null models to hypotheses. The nestedness of a matrix is the combination of marginal sum inequality and high overlap. The higher the overlap, the more predictable the cell values by marginal sums. Therefore, the only way a process can increase nestedness is by promoting marginal sum inequalities, without concomitantly introducing preferences. We propose a combination of null models to test for different topological hypotheses. In these null models, interactions are randomized following a probability matrix. The probability matrix of the equiprobable model excludes marginal sum inequalities and consequently all nestedness-generating mechanisms, providing a distribution of expected values for nestedness significance tests. The probability matrix of the proportional model, by conserving marginal sums and maximizing overlap, conserves nestedness-generating mechanisms and excludes nestedness-disrupting mechanisms, thereby yielding the expected nestedness values for fully nested matrices. We advance a new protocol to test for nestedness in binary and weighted matrices, in which those two null models represent different topological hypotheses. Our protocol helps to tell apart cases in which nestedness is different from expected by chance and cases in which nestedness represents the best topological archetype. We also evaluate the efficiency of applying this protocol using some common nestedness indices. Analyses based on weighted data proved to be highly efficient and informative, while binary analyses induced severe distortions. Finally, we empirically illustrate our approach using a plant-pollinator network. Through a shift of perspective, our approach reconciliates contradictions in null model analysis and provides a guideline for nestedness interpretation.
更多查看译文
关键词
nestedness,methodological solutions,meaning,novel perspective
AI 理解论文
溯源树
样例
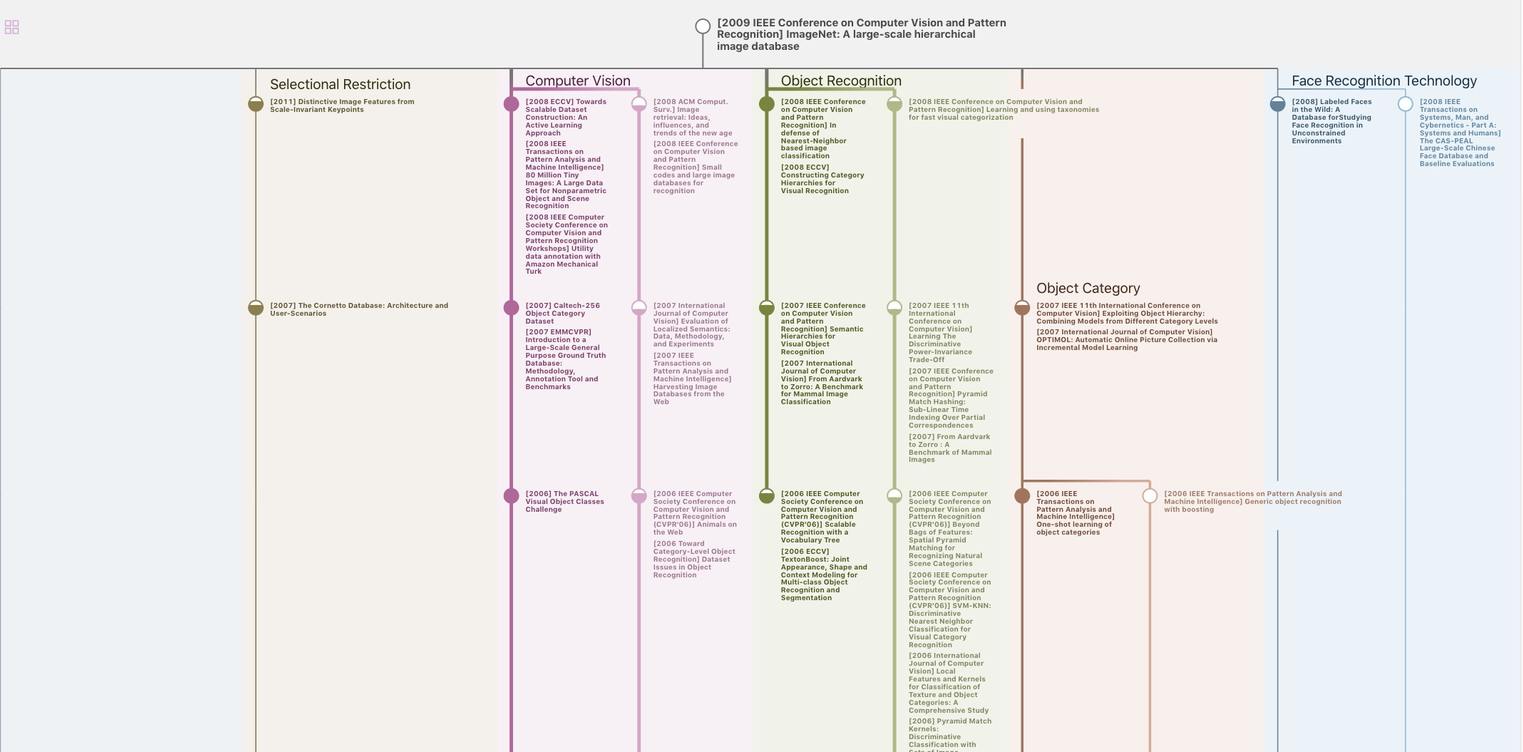
生成溯源树,研究论文发展脉络
Chat Paper
正在生成论文摘要