Personalized Federated Learning: A Unified Framework and Universal Optimization Techniques
arXiv (Cornell University)(2021)
摘要
We investigate the optimization aspects of personalized Federated Learning (FL). We propose general optimizers that can be applied to numerous existing personalized FL objectives, specifically a tailored variant of Local SGD and variants of accelerated coordinate descent/accelerated SVRCD. By examining a general personalized objective capable of recovering many existing personalized FL objectives as special cases, we develop a comprehensive optimization theory applicable to a wide range of strongly convex personalized FL models in the literature. We showcase the practicality and/or optimality of our methods in terms of communication and local computation. Remarkably, our general optimization solvers and theory can recover the best-known communication and computation guarantees for addressing specific personalized FL objectives. Consequently, our proposed methods can serve as universal optimizers, rendering the design of task-specific optimizers unnecessary in many instances.
更多查看译文
关键词
federated learning,unified framework,optimization
AI 理解论文
溯源树
样例
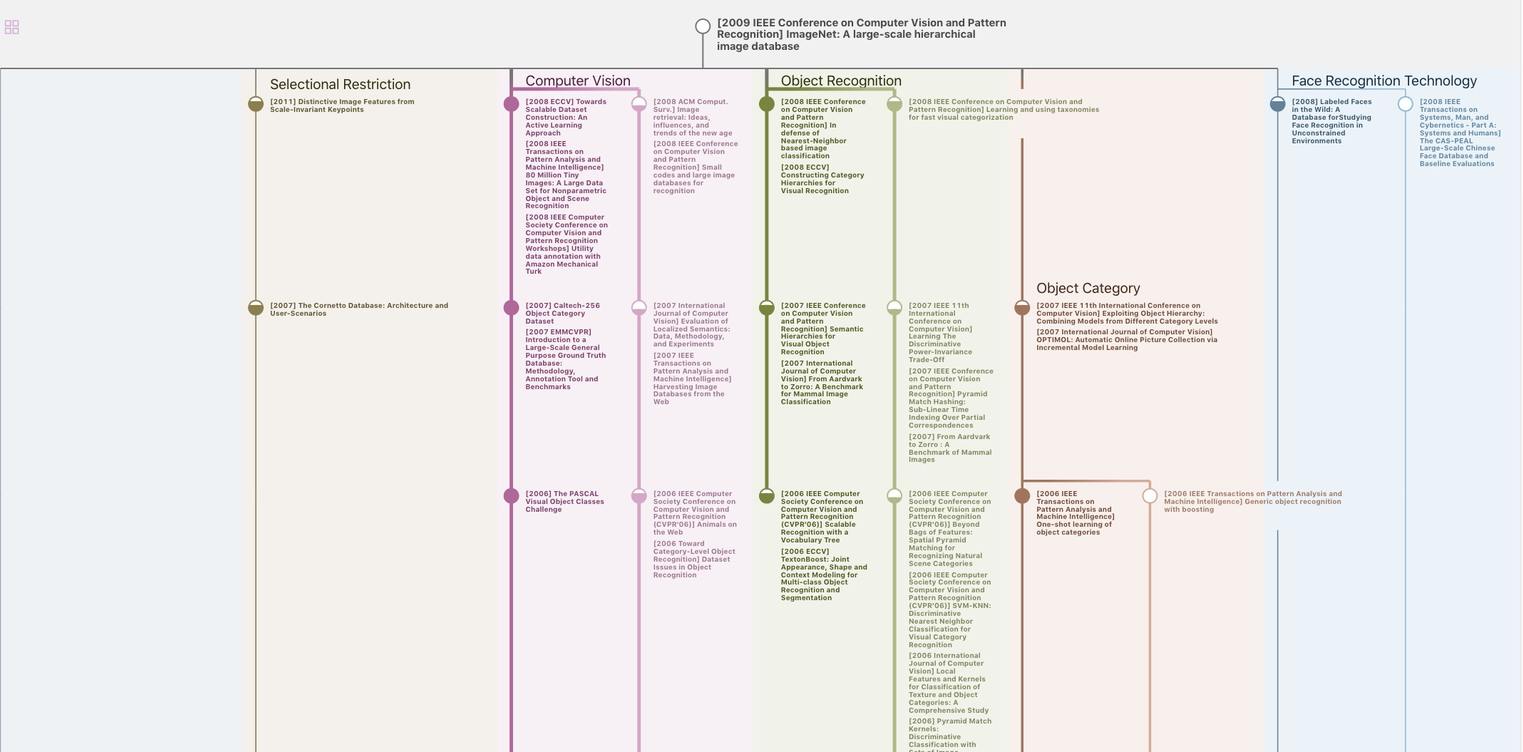
生成溯源树,研究论文发展脉络
Chat Paper
正在生成论文摘要