Seeing biodiversity: perspectives in machine learning for wildlife conservation
arXiv (Cornell University)(2021)
摘要
Data acquisition in animal ecology is rapidly accelerating due to inexpensive and accessible sensors such as smartphones, drones, satellites, audio recorders and bio-logging devices. These new technologies and the data they generate hold great potential for large-scale environmental monitoring and understanding, but are limited by current data processing approaches which are inefficient in how they ingest, digest, and distill data into relevant information. We argue that machine learning, and especially deep learning approaches, can meet this analytic challenge to enhance our understanding, monitoring capacity, and conservation of wildlife species. Incorporating machine learning into ecological workflows could improve inputs for population and behavior models and eventually lead to integrated hybrid modeling tools, with ecological models acting as constraints for machine learning models and the latter providing data-supported insights. In essence, by combining new machine learning approaches with ecological domain knowledge, animal ecologists can capitalize on the abundance of data generated by modern sensor technologies in order to reliably estimate population abundances, study animal behavior and mitigate human/wildlife conflicts. To succeed, this approach will require close collaboration and cross-disciplinary education between the computer science and animal ecology communities in order to ensure the quality of machine learning approaches and train a new generation of data scientists in ecology and conservation.
更多查看译文
关键词
wildlife conservation,biodiversity,machine learning,perspectives
AI 理解论文
溯源树
样例
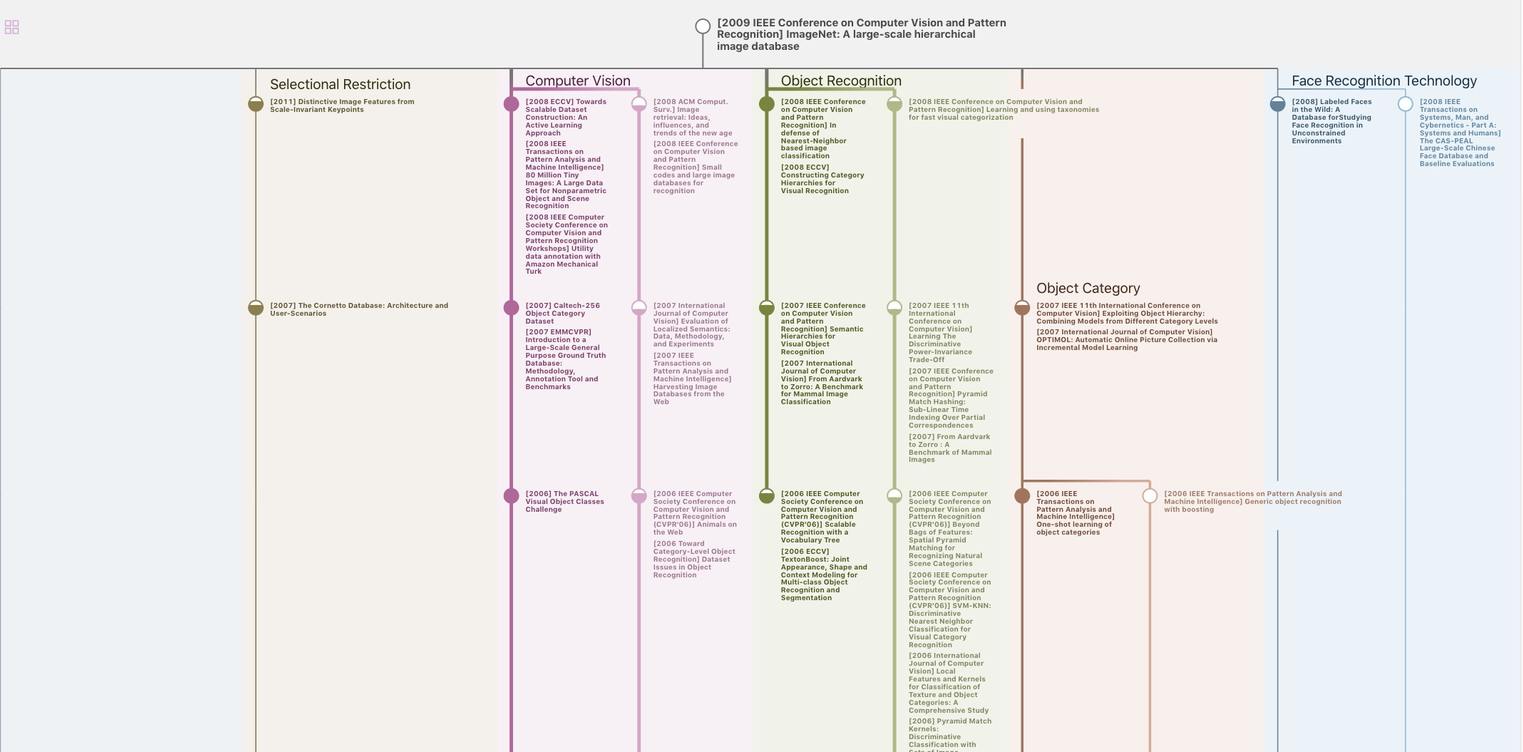
生成溯源树,研究论文发展脉络
Chat Paper
正在生成论文摘要