Predicting Emerging Themes in Rapidly Expanding COVID-19 Literature with Dynamic Word Embedding Networks and Machine Learning
medRxiv (Cold Spring Harbor Laboratory)(2021)
摘要
Abstract Evidence from peer-reviewed literature is the cornerstone for designing responses to global threats such as COVID-19. The collection of knowledge and interpretation in publications needs to be distilled into evidence by leveraging natural language in ways beyond standard meta-analysis. Several studies have focused on mining evidence from text using natural language processing, and have focused on a handful of diseases. Here we show that new knowledge can be captured, tracked and predicted using the evolution of unsupervised word embeddings and machine learning. Our approach to decipher the flow of latent knowledge in time-varying networks of word-vectors captured thromboembolic complications as an emerging theme in more than 77,000 peer-reviewed publications and more than 11,000 WHO vetted preprints on COVID-19. Furthermore, machine learning based prediction of emerging links in the networks reveals autoimmune diseases, multisystem inflammatory syndrome and neurological complications as a dominant research theme in COVID-19 publications starting March 2021.
更多查看译文
关键词
dynamic word,emerging themes,literature,machine learning
AI 理解论文
溯源树
样例
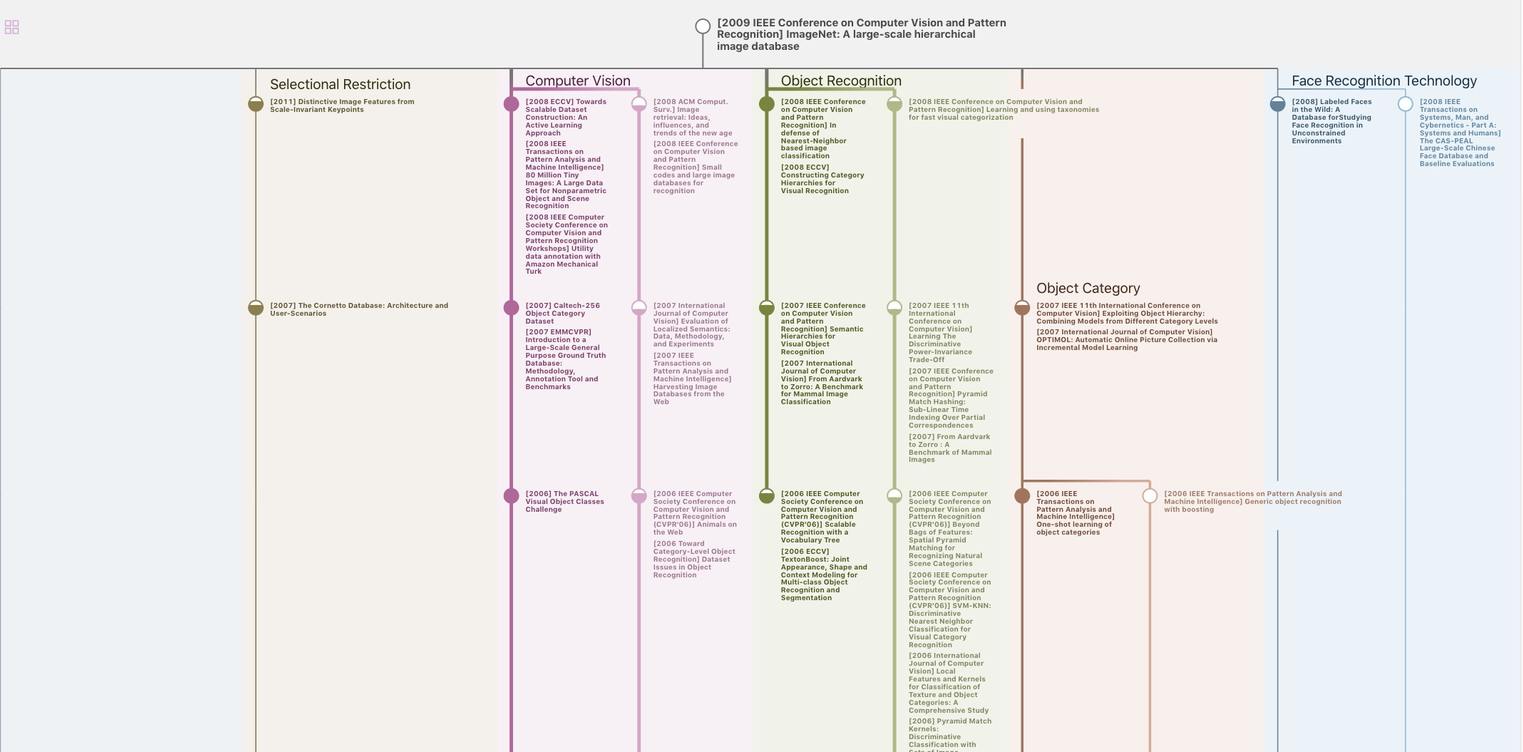
生成溯源树,研究论文发展脉络
Chat Paper
正在生成论文摘要