Sliced Multi-Marginal Optimal Transport.
arXiv (Cornell University)(2021)
摘要
We study multi-marginal optimal transport, a generalization of optimal transport that allows us to define discrepancies between multiple measures. It provides a framework to solve multi-task learning problems and to perform barycentric averaging. However, multi-marginal distances between multiple measures are typically challenging to compute because they require estimating a transport plan with $N^P$ variables. In this paper, we address this issue in the following way: 1) we efficiently solve the one-dimensional multi-marginal Monge-Wasserstein problem for a classical cost function in closed form, and 2) we propose a higher-dimensional multi-marginal discrepancy via slicing and study its generalized metric properties. We show that computing the sliced multi-marginal discrepancy is massively scalable for a large number of probability measures with support as large as $10^7$ samples. Our approach can be applied to solving problems such as barycentric averaging, multi-task density estimation and multi-task reinforcement learning.
更多查看译文
关键词
transport,optimal,multi-marginal
AI 理解论文
溯源树
样例
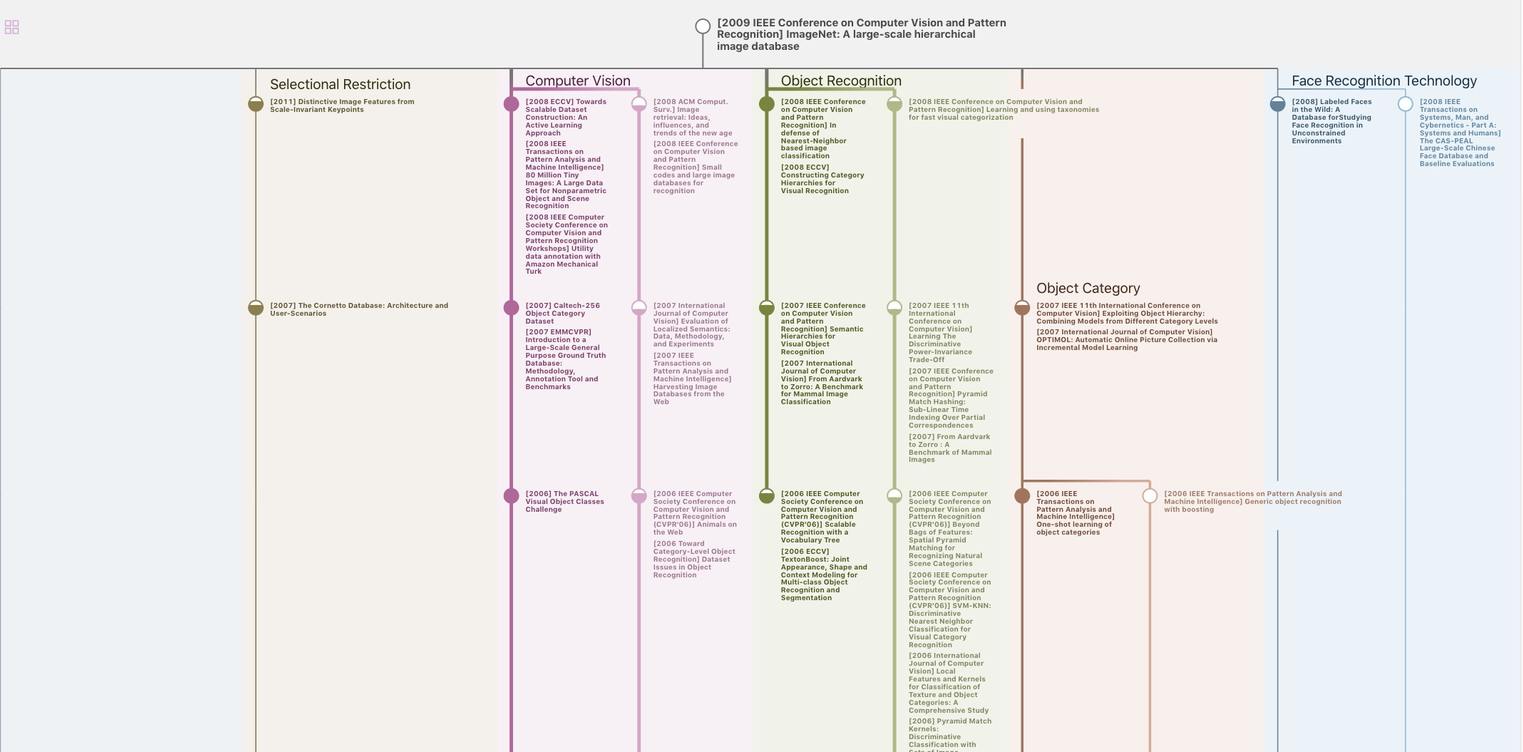
生成溯源树,研究论文发展脉络
Chat Paper
正在生成论文摘要