On Symmetric Rectilinear Matrix Partitioning.
arXiv (Cornell University)(2020)
摘要
Even distribution of irregular workload to processing units is crucial for efficient parallelization in many applications. In this work, we are concerned with a spatial partitioning called rectilinear partitioning (also known as generalized block distribution) of sparse matrices. More specifically, in this work, we address the problem of symmetric rectilinear partitioning of a square matrix. By symmetric, we mean the rows and columns of the matrix are identically partitioned yielding a tiling where the diagonal tiles (blocks) will be squares. We first show that the optimal solution to this problem is NP-hard, and we propose four heuristics to solve two different variants of this problem. We present a thorough analysis of the computational complexities of those proposed heuristics. To make the proposed techniques more applicable in real life application scenarios, we further reduce their computational complexities by utilizing effective sparsification strategies together with an efficient sparse prefix-sum data structure. We experimentally show the proposed algorithms are efficient and effective on more than six hundred test matrices. With sparsification, our methods take less than 3 seconds in the Twitter graph on a modern 24 core system and output a solution whose load imbalance is no worse than 1%.
更多查看译文
关键词
symmetric rectilinear matrix partitioning
AI 理解论文
溯源树
样例
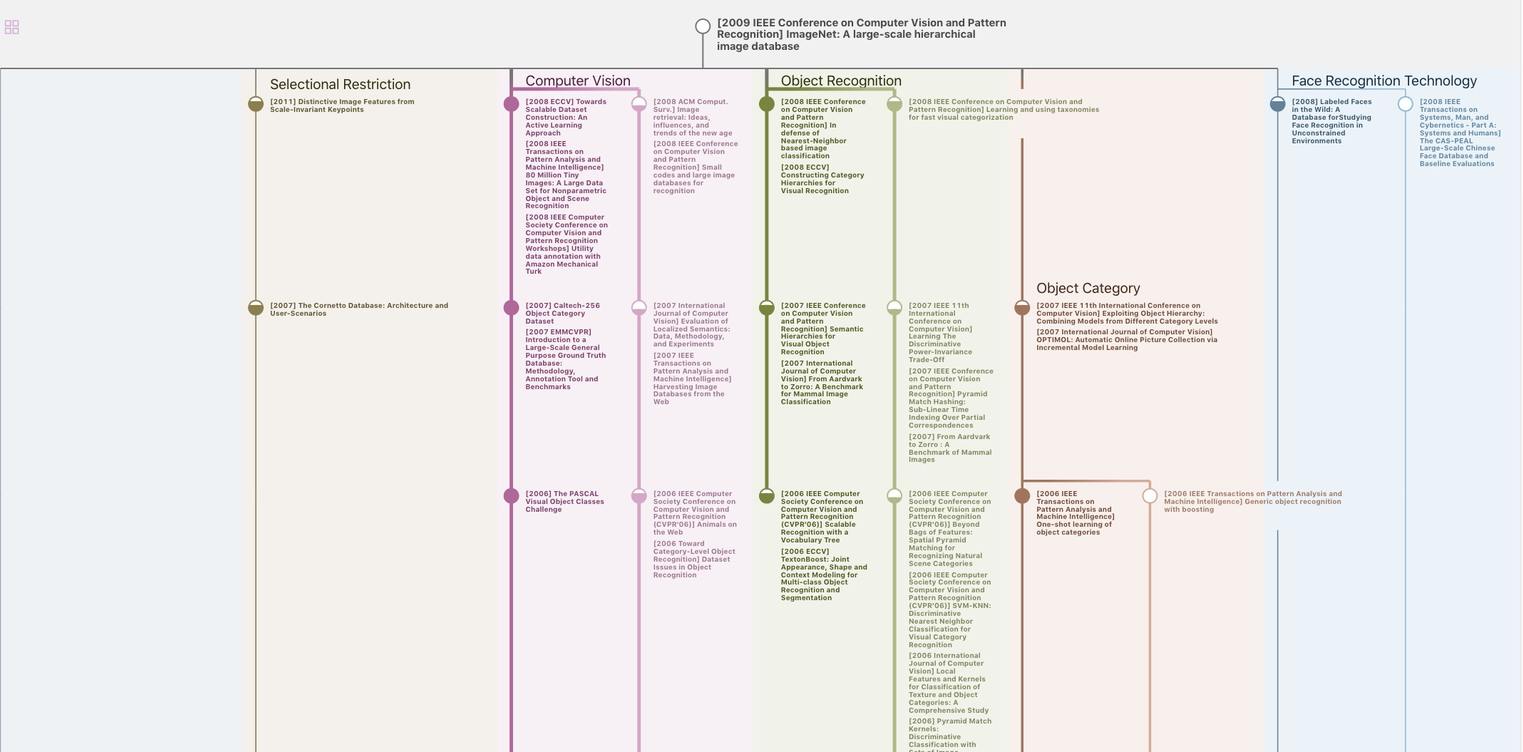
生成溯源树,研究论文发展脉络
Chat Paper
正在生成论文摘要