iMLCA: Machine Learning-powered Iterative Combinatorial Auctions with Interval Bidding
arXiv (Cornell University)(2020)
摘要
Preference elicitation is a major challenge in large combinatorial auctions because the bundle space grows exponentially in the number of items. Recent work has used machine learning (ML) algorithms to identify a small set of bundles to query from each bidder. However, a shortcoming of this prior work is that bidders must submit exact values for the queried bundles, which can be quite costly. To address this, we propose iMLCA, a new ML-powered iterative combinatorial auction with interval bidding (i.e., where bidders submit upper and lower bounds instead of exact values). To steer the auction towards an efficient allocation, we introduce a price-based activity rule, asking bidders to tighten bounds on relevant bundles only. In our experiments, iMLCA achieves the same allocative efficiency as the prior ML-based auction that uses exact bidding. Moreover, it outperforms the well-known combinatorial clock auction in a realistically-sized domain.
更多查看译文
关键词
iterative combinatorial auctions,learning-powered
AI 理解论文
溯源树
样例
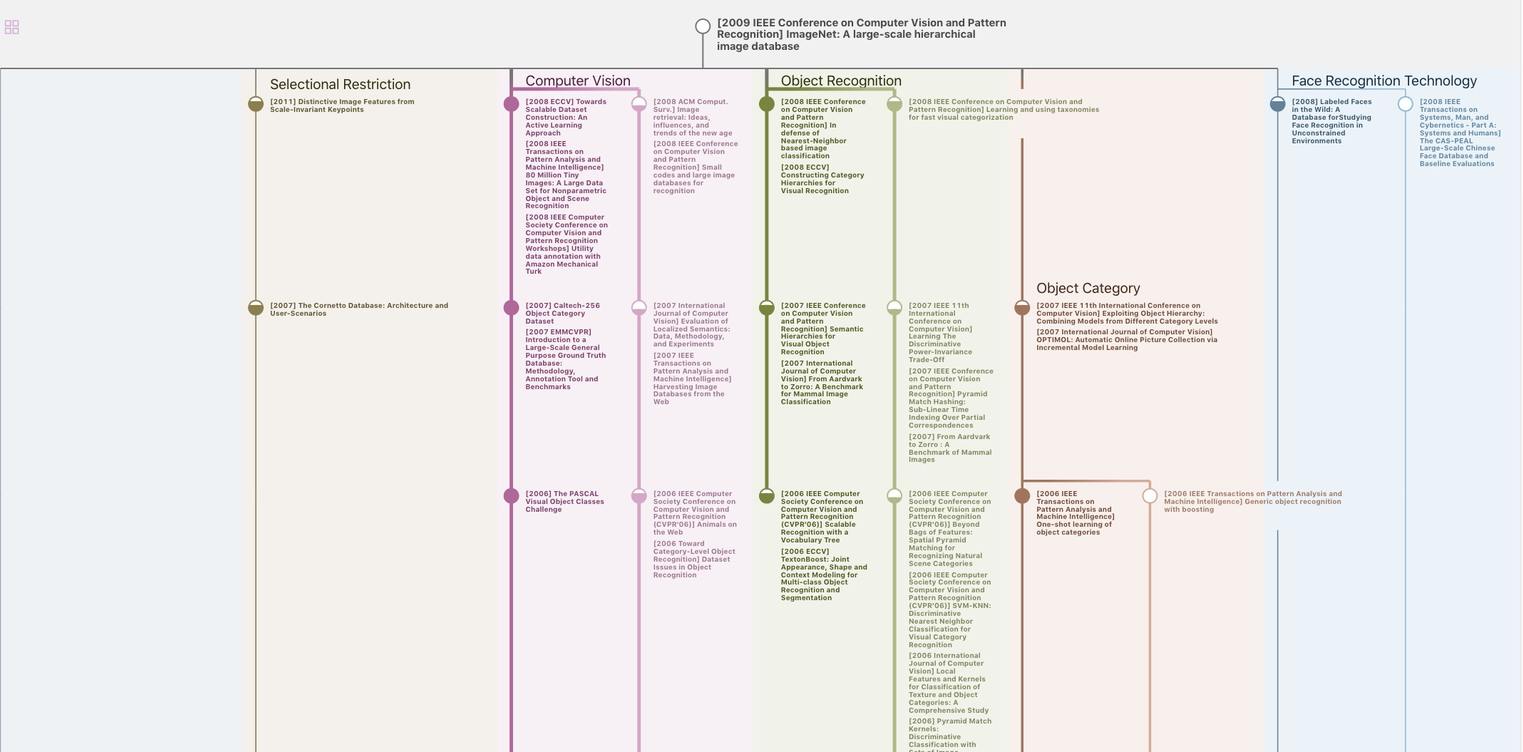
生成溯源树,研究论文发展脉络
Chat Paper
正在生成论文摘要