QA2Explanation: Generating and Evaluating Explanations for Question Answering Systems over Knowledge Graph
arXiv (Cornell University)(2020)
摘要
In the era of Big Knowledge Graphs, Question Answering (QA) systems have reached a milestone in their performance and feasibility. However, their applicability, particularly in specific domains such as the biomedical domain, has not gained wide acceptance due to their "black box" nature, which hinders transparency, fairness, and accountability of QA systems. Therefore, users are unable to understand how and why particular questions have been answered, whereas some others fail. To address this challenge, in this paper, we develop an automatic approach for generating explanations during various stages of a pipeline-based QA system. Our approach is a supervised and automatic approach which considers three classes (i.e., success, no answer, and wrong answer) for annotating the output of involved QA components. Upon our prediction, a template explanation is chosen and integrated into the output of the corresponding component. To measure the effectiveness of the approach, we conducted a user survey as to how non-expert users perceive our generated explanations. The results of our study show a significant increase in the four dimensions of the human factor from the Human-computer interaction community.
更多查看译文
关键词
word segmentation experiments,linguist,languages,low-resource
AI 理解论文
溯源树
样例
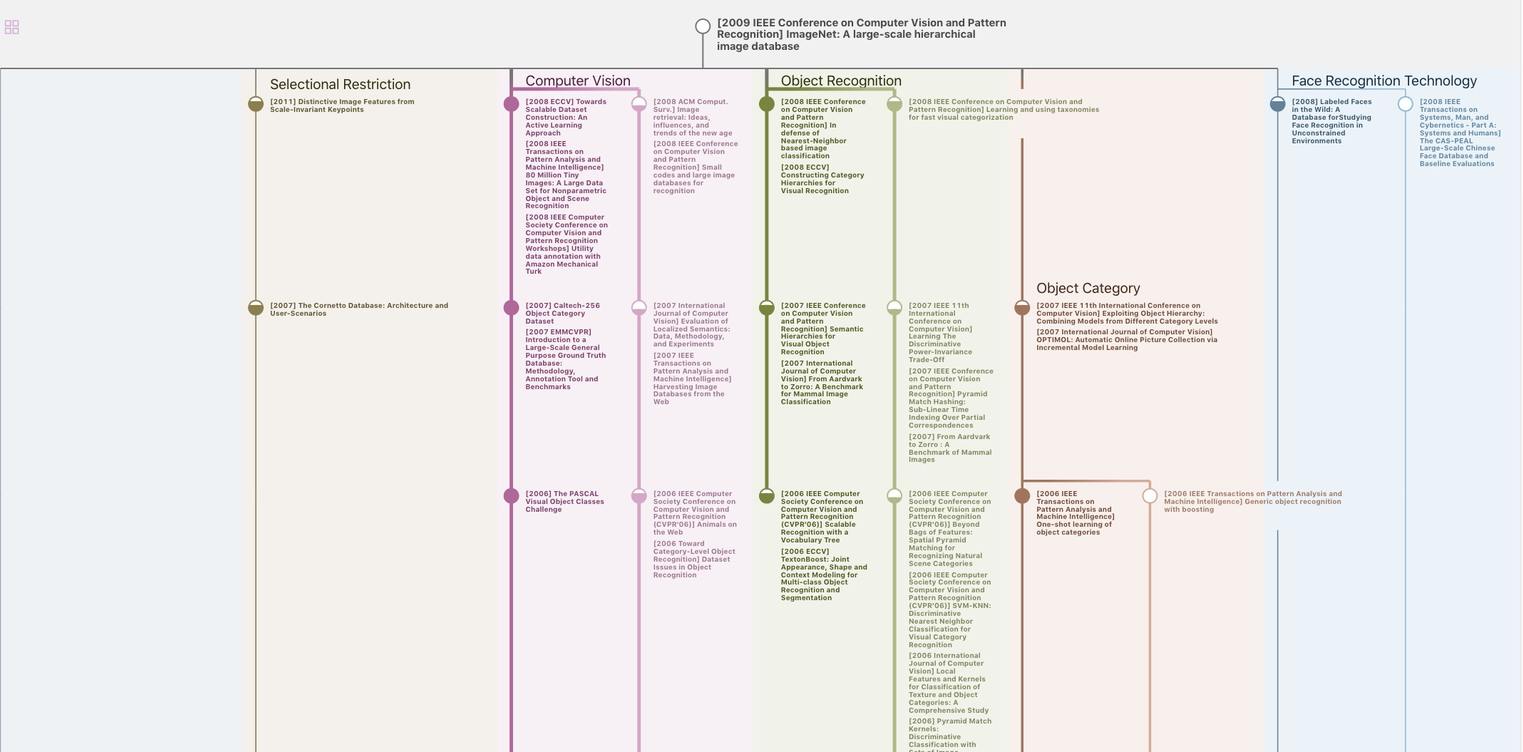
生成溯源树,研究论文发展脉络
Chat Paper
正在生成论文摘要