Deep learning analysis of intracranial EEG for recognizing drug effects and mechanisms of action
arXiv (Cornell University)(2020)
Abstract
Drug-target interaction (DTI) prediction has become a foundational task in drug repositioning, polypharmacology, drug discovery, as well as drug resistance and side-effect prediction. DTI identification using machine learning is gaining popularity in these research areas. Through the years, numerous deep learning methods have been proposed for DTI prediction. Nevertheless, prediction accuracy and efficiency remain key challenges. Pharmaco-electroencephalogram (pharmaco-EEG) is considered valuable in the development of central nervous system-active drugs. Quantitative EEG analysis demonstrates high reliability in studying the effects of drugs on the brain. Earlier preclinical pharmaco-EEG studies showed that different types of drugs can be classified according to their mechanism of action on neural activity. Here, we propose a convolutional neural network for EEG-mediated DTI prediction. This new approach can explain the mechanisms underlying complicated drug actions, as it allows the identification of similarities in the mechanisms of action and effects of psychotropic drugs.
MoreTranslated text
Key words
intracranial eeg,drug effects
AI Read Science
Must-Reading Tree
Example
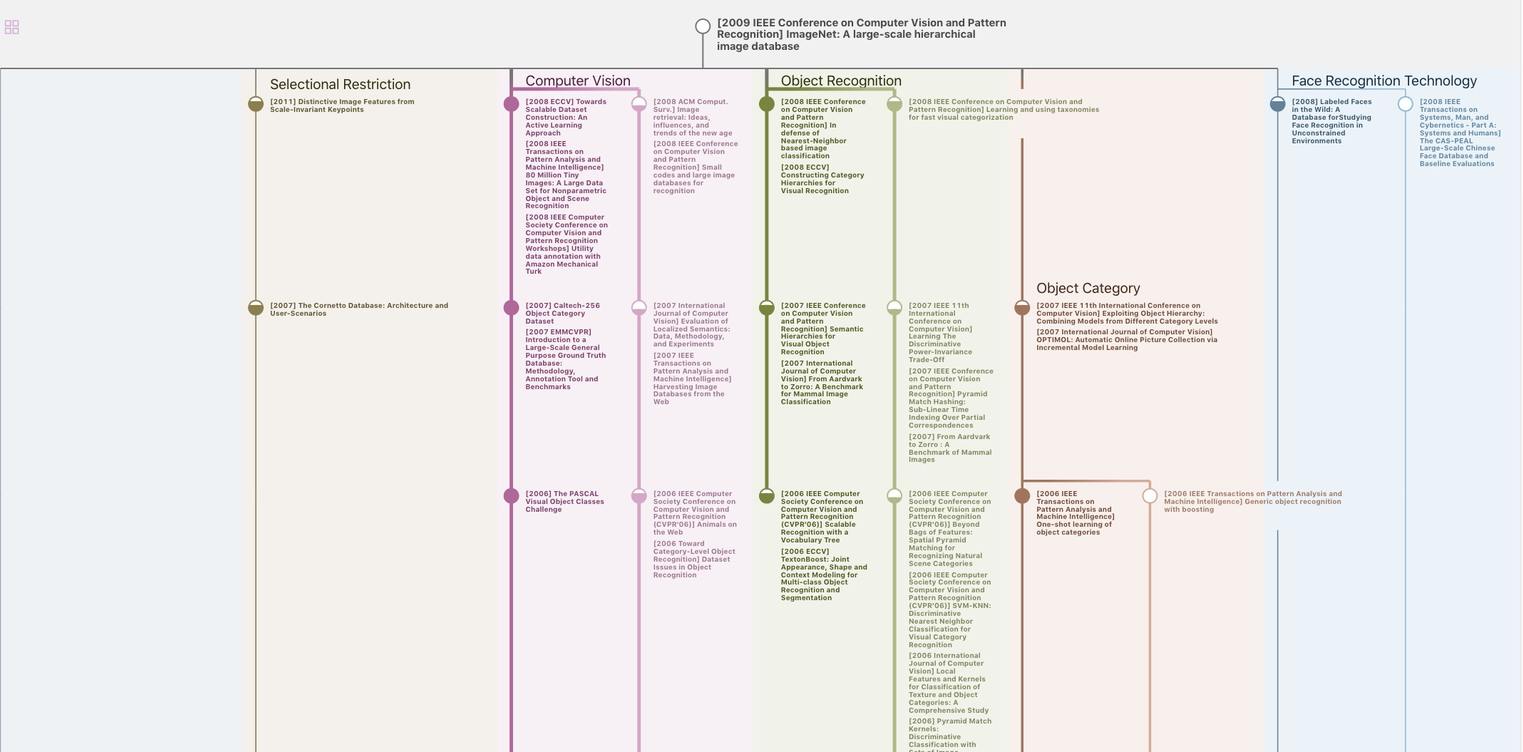
Generate MRT to find the research sequence of this paper
Chat Paper
Summary is being generated by the instructions you defined