A Coupled Manifold Optimization Framework to Jointly Model the Functional Connectomics and Behavioral Data Spaces
arXiv (Cornell University)(2020)
摘要
The problem of linking functional connectomics to behavior is extremely challenging due to the complex interactions between the two distinct, but related, data domains. We propose a coupled manifold optimization framework which projects fMRI data onto a low dimensional matrix manifold common to the cohort. The patient specific loadings simultaneously map onto a behavioral measure of interest via a second, non-linear, manifold. By leveraging the kernel trick, we can optimize over a potentially infinite dimensional space without explicitly computing the embeddings. As opposed to conventional manifold learning, which assumes a fixed input representation, our framework directly optimizes for embedding directions that predict behavior. Our optimization algorithm combines proximal gradient descent with the trust region method, which has good convergence guarantees. We validate our framework on resting state fMRI from fifty-eight patients with Autism Spectrum Disorder using three distinct measures of clinical severity. Our method outperforms traditional representation learning techniques in a cross validated setting, thus demonstrating the predictive power of our coupled objective.
更多查看译文
关键词
functional connectomics,coupled manifold optimization framework,data
AI 理解论文
溯源树
样例
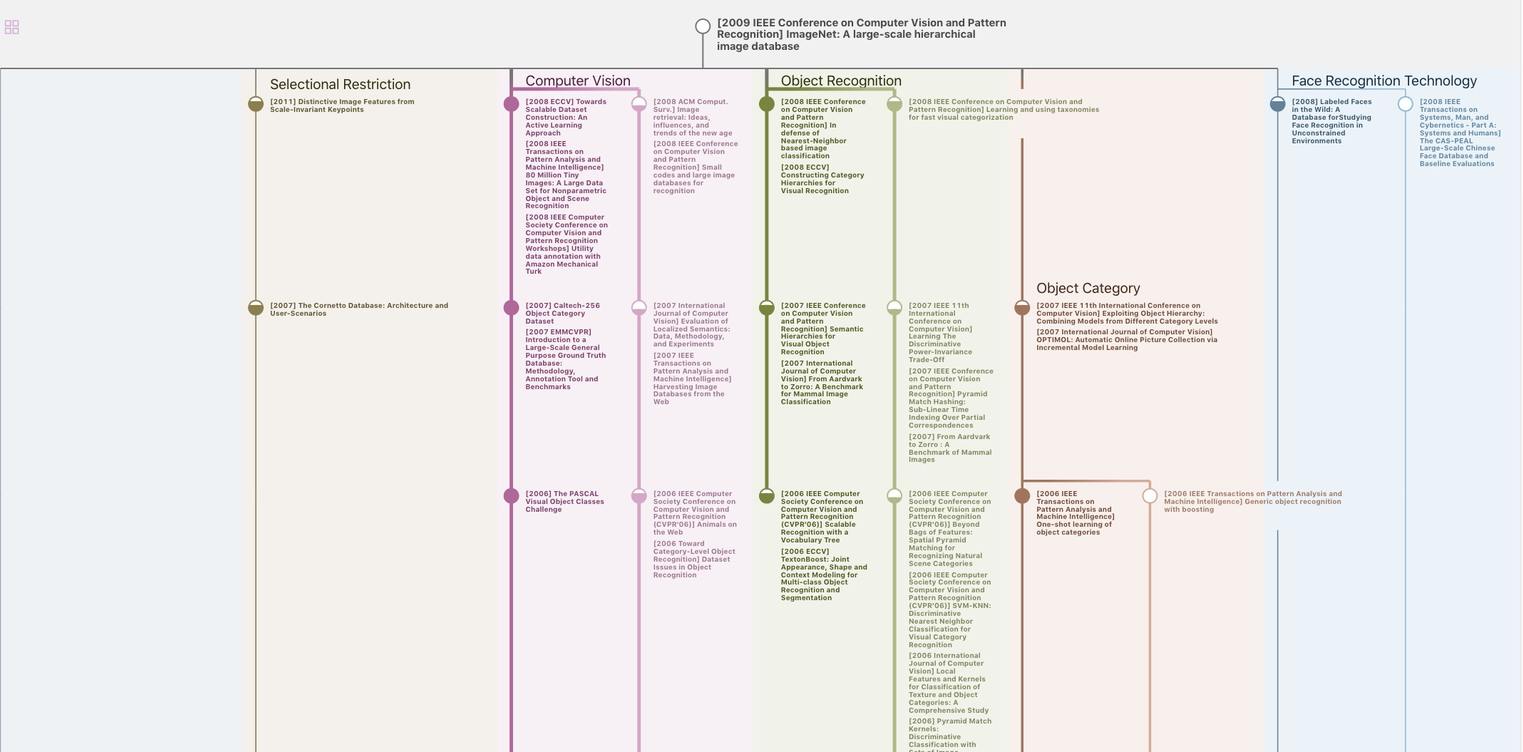
生成溯源树,研究论文发展脉络
Chat Paper
正在生成论文摘要