Direct loss minimization algorithms for Bayesian predictors
arXiv (Cornell University)(2020)
摘要
Recent work proposed direct loss minimization (DLM), which minimizes the predictive loss of a hypothetical posterior, as an alternative to variational inference, and showed that such approaches come with strong theoretical guarantees. However the use of DLM for Bayesian predictors has not been thoroughly evaluated to date. One difficulty with using DLM is the fact that, for log-loss, the objective function includes a complex term with a logarithm of an expectation (logE) that is not easily amenable to stochastic optimization with unbiased samples. The paper makes three contributions in this context. The first is a new method using product sampling for unbiased estimates of gradients (uPS) for objectives with LogE terms. The second is an analysis of biased Monte Carlo (bMC), a simple form of biased sampling of gradients for logE terms, showing that stochastic gradient descent converges despite the biased gradients. The third is an empirical evaluation of DLM in sparse Gaussian processes, including log loss for the conjugate and non-conjugate cases and square loss for regression. The results show that DLM is an effective approach which in some cases matches and in some cases significantly improves over the performance of variational inference. Results comparing the sampling methods show that uPS is potentially more sample-efficient but bMC provides a better tradeoff in terms of convergence time and computational efficiency.
更多查看译文
关键词
direct loss minimization algorithms,bayesian predictors
AI 理解论文
溯源树
样例
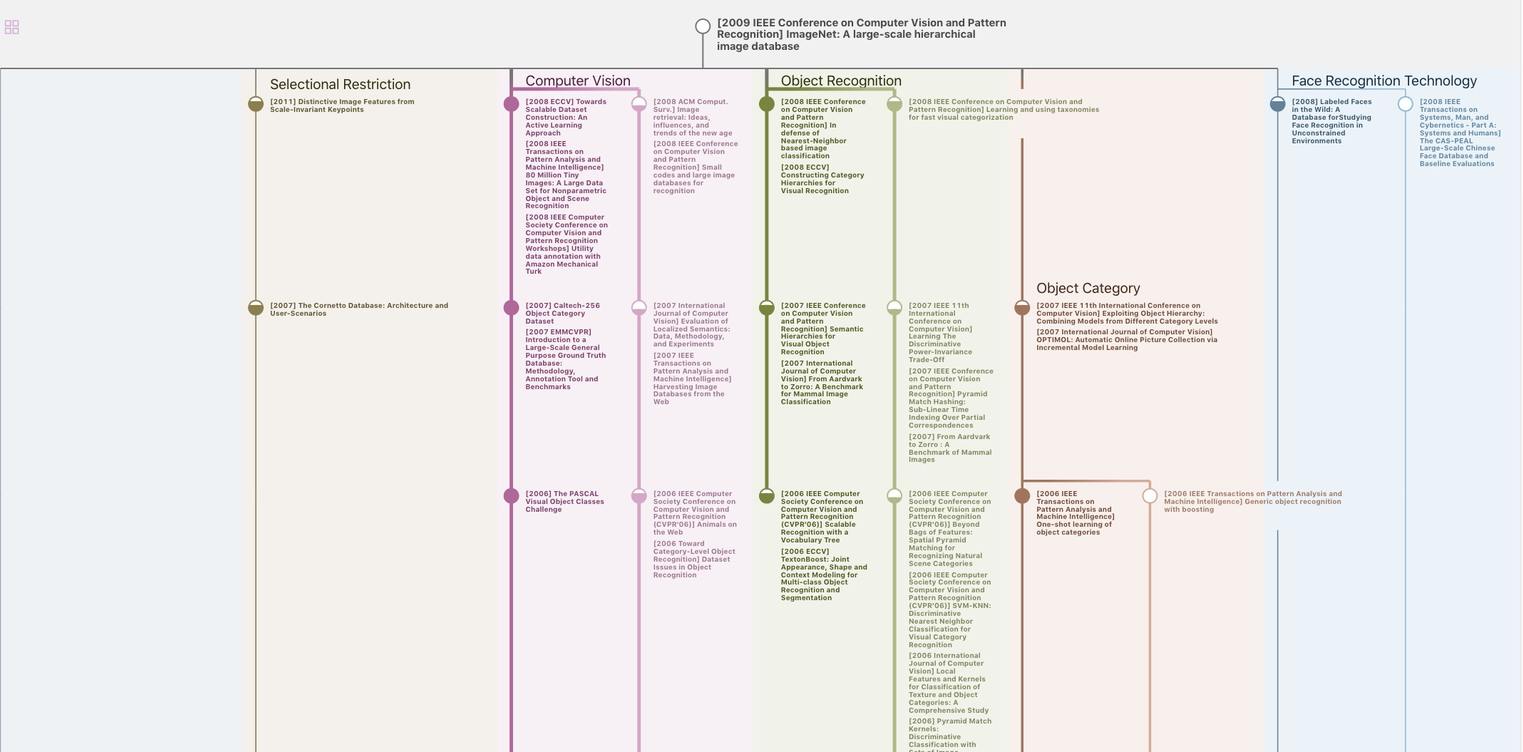
生成溯源树,研究论文发展脉络
Chat Paper
正在生成论文摘要