Time Series Classification for Locating Forced Oscillation Sources
arXiv (Cornell University)(2020)
Abstract
Forced oscillations are caused by sustained cyclic disturbances. This paper presents a machine learning (ML) based time-series classification method that uses the synchrophasor measurements to locate the sources of forced oscillations for fast disturbance removal. Sequential feature selection is used to identify the most informative measurements of each power plant so that multivariate time series (MTS) can be constructed. By training the Mahalanobis matrix, we measure and compare the distance between the MTSs. Templates for representing each class is constructed to reduce the size of training datasets and improve the online matching efficiency. Dynamic time warping (DTW) algorithm is used to align the out-of-sync MTSs to account for oscillation detection errors. The algorithm is validated on two test systems: the IEEE 39-bus system and the WECC 179-bus system. When a forced oscillation occurs, MTSs will be constructed by designated PMU measurements. Then, the MTSs will be classified by the trained classifiers, the class membership of which corresponds to the location of each oscillation source. Simulation results show that the proposed method can be used online to identify the forced oscillation sources with high accuracy. The robustness of the proposed algorithm in the presence of oscillation detection errors is also quantified.
MoreTranslated text
Key words
forced oscillation sources,classification
AI Read Science
Must-Reading Tree
Example
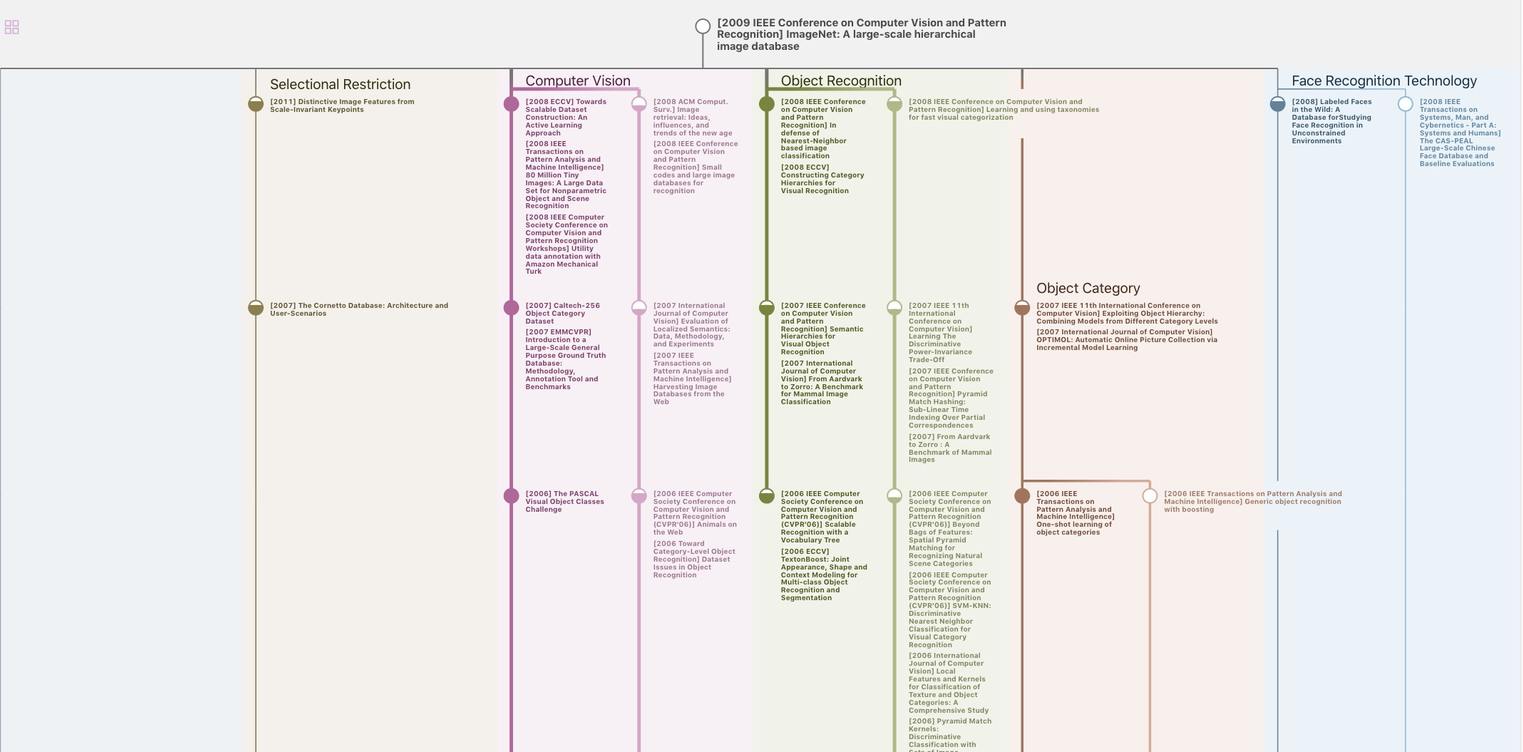
Generate MRT to find the research sequence of this paper
Chat Paper
Summary is being generated by the instructions you defined