Data-Driven Assisted Chance-Constrained Energy and Reserve Scheduling with Wind Curtailment
arXiv (Cornell University)(2020)
摘要
Chance-constrained optimization (CCO) has been widely used for uncertainty management in power system operation. With the prevalence of wind energy, it becomes possible to consider the wind curtailment as a dispatch variable in CCO. However, the wind curtailment will cause impulse for the uncertainty distribution, yielding challenges for the chance constraints modeling. To deal with that, a data-driven framework is developed. By modeling the wind curtailment as a cap enforced on the wind power output, the proposed framework constructs a Gaussian process (GP) surrogate to describe the relationship between wind curtailment and the chance constraints. This allows us to reformulate the CCO with wind curtailment as a mixed-integer second-order cone programming (MI-SOCP) problem. An error correction strategy is developed by solving a convex linear programming (LP) to improve the modeling accuracy. Case studies performed on the PJM 5-bus and IEEE 118-bus systems demonstrate that the proposed method is capable of accurately accounting the influence of wind curtailment dispatch in CCO.
更多查看译文
关键词
reserve scheduling
AI 理解论文
溯源树
样例
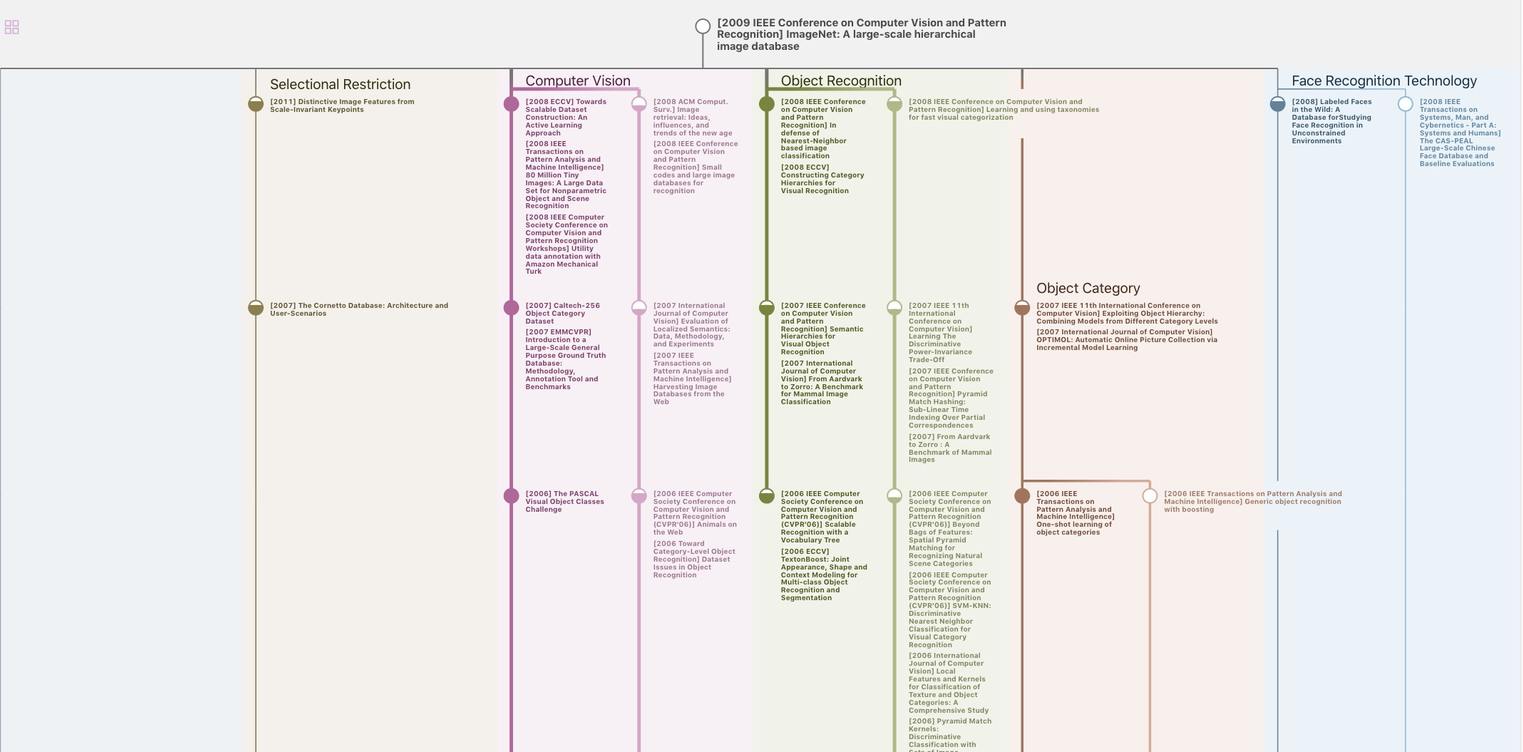
生成溯源树,研究论文发展脉络
Chat Paper
正在生成论文摘要