Electromyographic and Acceleration Signals-Based Gait Phase Analysis for Multiple Terrain Classification Using Deep Learning
Social Science Research Network(2020)
Abstract
The neuro-muscular activity emanated from the human leg muscles is of great importance for understanding the human locomotion. This information can be extracted by using surface EMG signals. This paper presents the concept of gait analysis by using a pair of hybrid- EMG and acceleration sensors attached to the Tibialis Anterior and Gastrocnemius muscles of both legs. The response of the EMG signal envelope is analyzed for five locomotion terrains, namely- level ground, ramp ascent, ramp descent, stair ascent, and stair descent. A heuristic algorithm using an acceleration signal is employed to identify heel-strike, toe-off, and mid-swing events. Results show that we identified all these events correctly for five healthy subjects walking on all five terrains. Also, deep learning-based classification is performed for the above five terrains by using both stance and swing phase data separately as a training data set. We obtained more than 99% accuracy for both stance and swing phase training datasets; and for unknown data set, classification accuracy is found to be 97.55% from stance phase data and 91.22% from swing phase data.
MoreTranslated text
Key words
gait phase analysis,multiple terrain classification,deep learning,signals-based
AI Read Science
Must-Reading Tree
Example
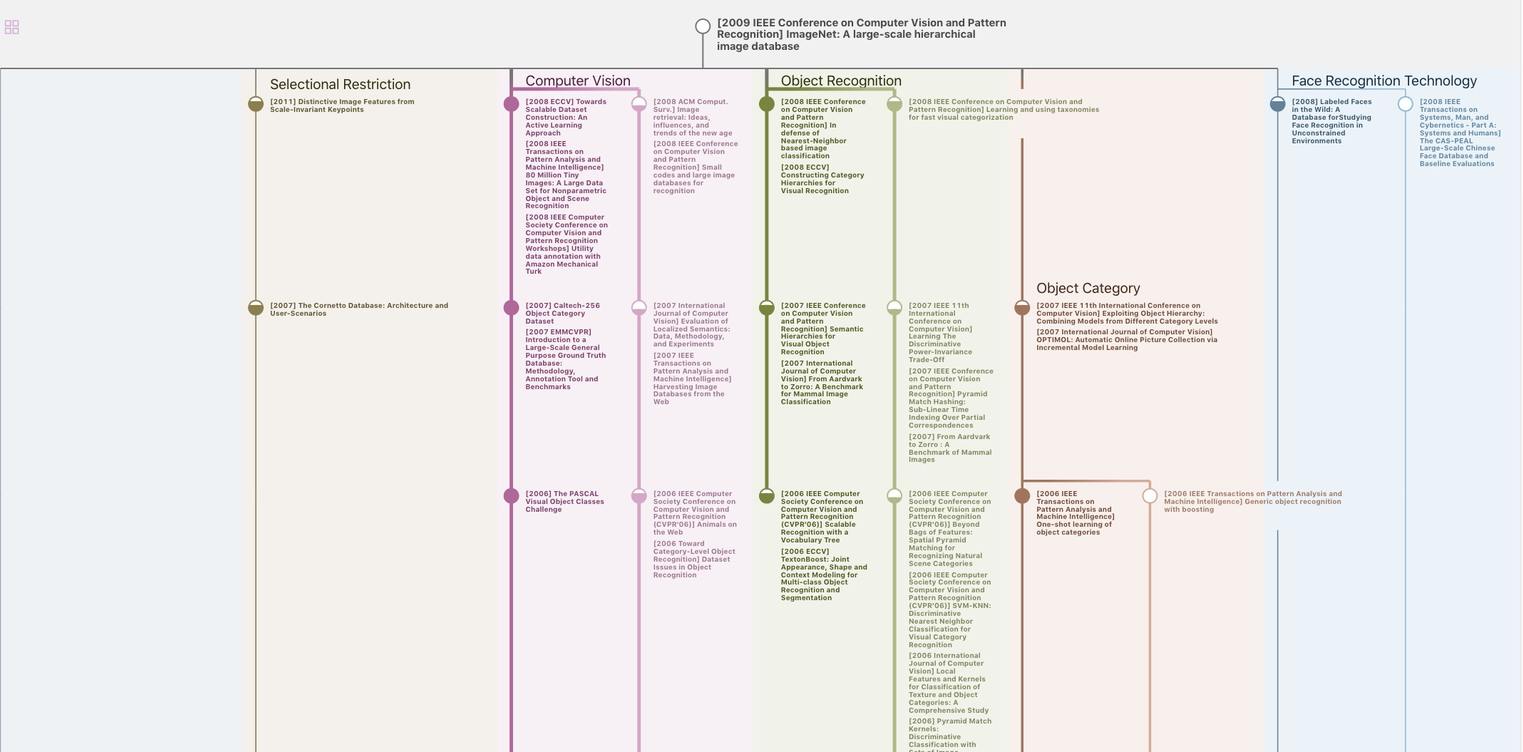
Generate MRT to find the research sequence of this paper
Chat Paper
Summary is being generated by the instructions you defined