Machine learning by means of electrical impedance spectroscopy measurements for the classification of upper limbs.
Revista Argentina de Bioingeniería(2020)
摘要
Wearable devices have become very prevalent in everyday life. Some of these devices have functions of acquisition of physiological signals that allow monitoring the health status of a person throughout the day, while using the device, so they have attracted interest in recent years. Electrical Impedance Spectroscopy (EIS) has been used to identify the conditions of a tissue under test, being potentially useful for the development of diagnostic devices, monitoring or recognition of the patient. The use of machine learning classifiers through EIS has been studied for the development of diagnostic assistance devices, biometric patient recognition, among other applications. In this work, we evaluate the use of EIS measurements on the biceps to identify the right or left arm, from which the measurements were taken. The performance of four classification models was evaluated: Random Forest, Linear Discriminant Analysis, K-Means and Naive Bayes, in order to identify their potential use in wearable devices. The Random Forest model obtained the best performance, reaching 100% of correct classifications in one of ten repetitions. More studies are necessary to better understand the results obtained, while evaluating the optimal selection of features and parameters to improve the overall performance of the classification models.
更多查看译文
关键词
electrical impedance,upper limbs,classification,machine learning,spectroscopy measurements
AI 理解论文
溯源树
样例
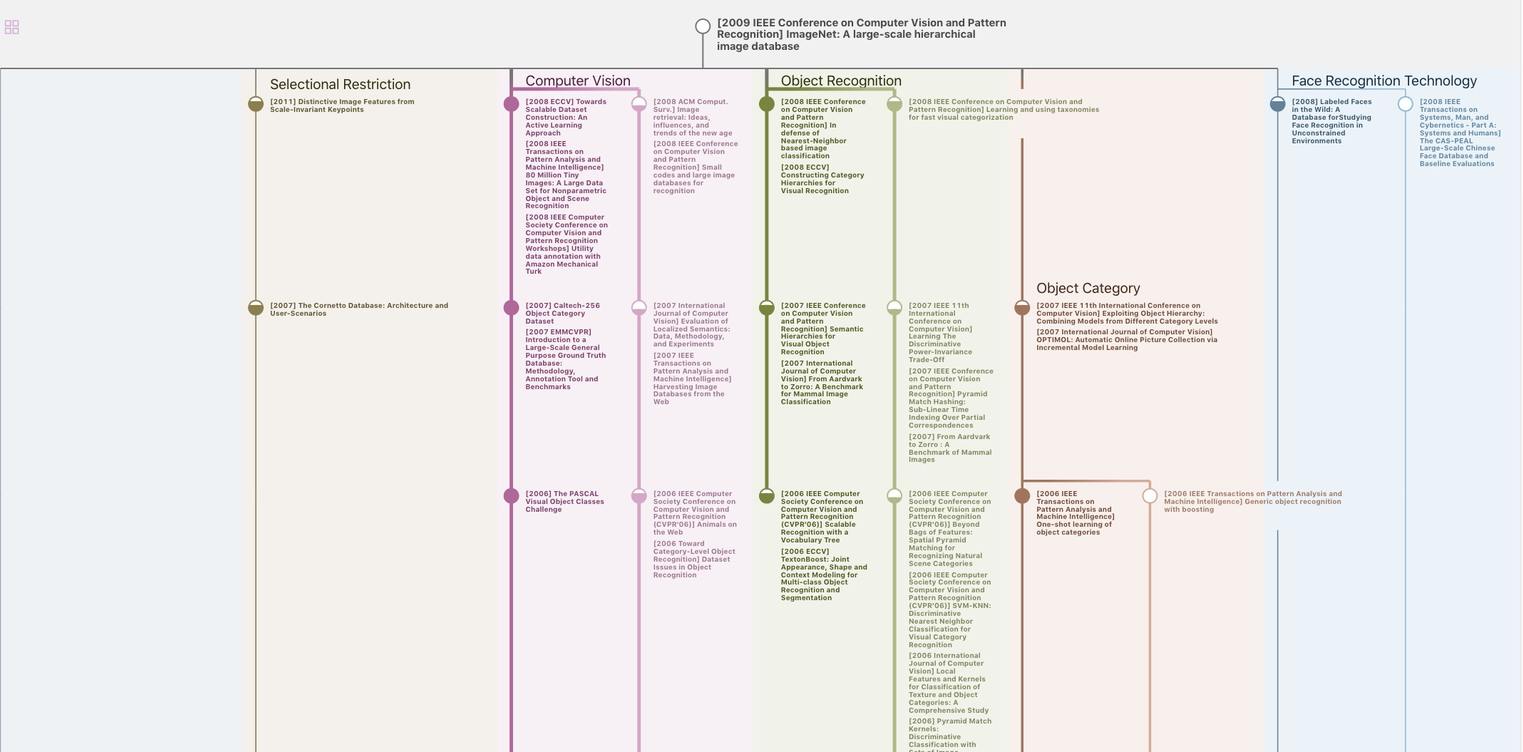
生成溯源树,研究论文发展脉络
Chat Paper
正在生成论文摘要