A Model Personalization-based Federated Learning Approach for Heterogeneous Participants with Variability in the Dataset
ACM TRANSACTIONS ON SENSOR NETWORKS(2024)
摘要
Federated learning is an emerging paradigm that provides privacy-preserving collaboration among multiple participants for model training without sharing private data. The participants with heterogeneous devices and networking resources decelerate the training and aggregation. The dataset of the participant also possesses a high level of variability, which means the characteristics of the dataset change over time. Moreover, it is a prerequisite to preserve the personalized characteristics of the local dataset on each participant device to achieve better performance. This article proposes a model personalization-based federated learning approach in the presence of variability in the local datasets. The approach involves participants with heterogeneous devices and networking resources. The central server initiates the approach and constructs a base model that executes on most participants. The approach simultaneously learns the personalized model and handles the variability in the datasets. We propose a knowledge distillation-based early-halting approach for devices where the base model does not fit directly. The early halting speeds up the training of the model. We also propose an aperiodic global update approach that helps participants to share their updated parameters aperiodically with server. Finally, we perform a real-world study to evaluate the performance of the approach and compare with state-of-the-art techniques.
更多查看译文
关键词
Dataset variability,early halting,federated learning,personalization
AI 理解论文
溯源树
样例
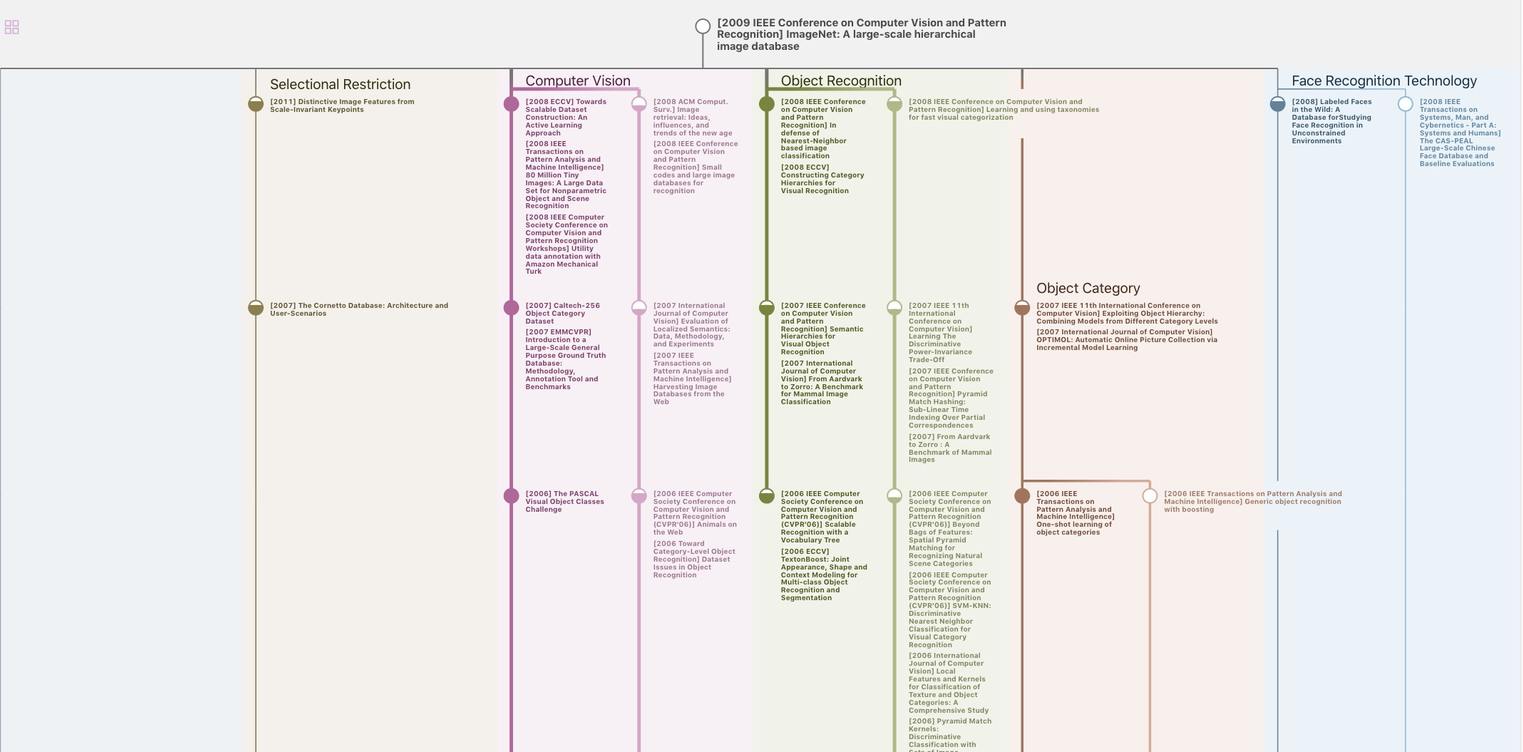
生成溯源树,研究论文发展脉络
Chat Paper
正在生成论文摘要