Robot Inner Attention Modeling for Task-Adaptive Teaming of Heterogeneous Multi Robots
arXiv (Cornell University)(2020)
摘要
Attracted by team scale and function diversity, a heterogeneous multi-robot system (HMRS), where multiple robots with different functions and numbers are coordinated to perform tasks, has been widely used for complex and large-scale scenarios, including disaster search and rescue, site surveillance, and social security. However, due to the variety of the task requirements, it is challenging to accurately compose a robot team with appropriate sizes and functions to dynamically satisfy task needs while limiting the robot resource cost to a low level. To solve this problem, in this paper, a novel adaptive cooperation method, inner attention (innerATT), is developed to flexibly team heterogeneous robots to execute tasks as task types and environment change. innerATT is designed by integrating a novel attention mechanism into a multi-agent actor-critic reinforcement learning architecture. With an attention mechanism, robot capability will be analyzed to flexibly form teams to meet task requirements. Scenarios with different task variety ("Single Task", "Double Task", and "Mixed Task") were designed. The effectiveness of the innerATT was validated by its accuracy in flexible cooperation.
更多查看译文
关键词
heterogeneous multi robots,task-adaptive
AI 理解论文
溯源树
样例
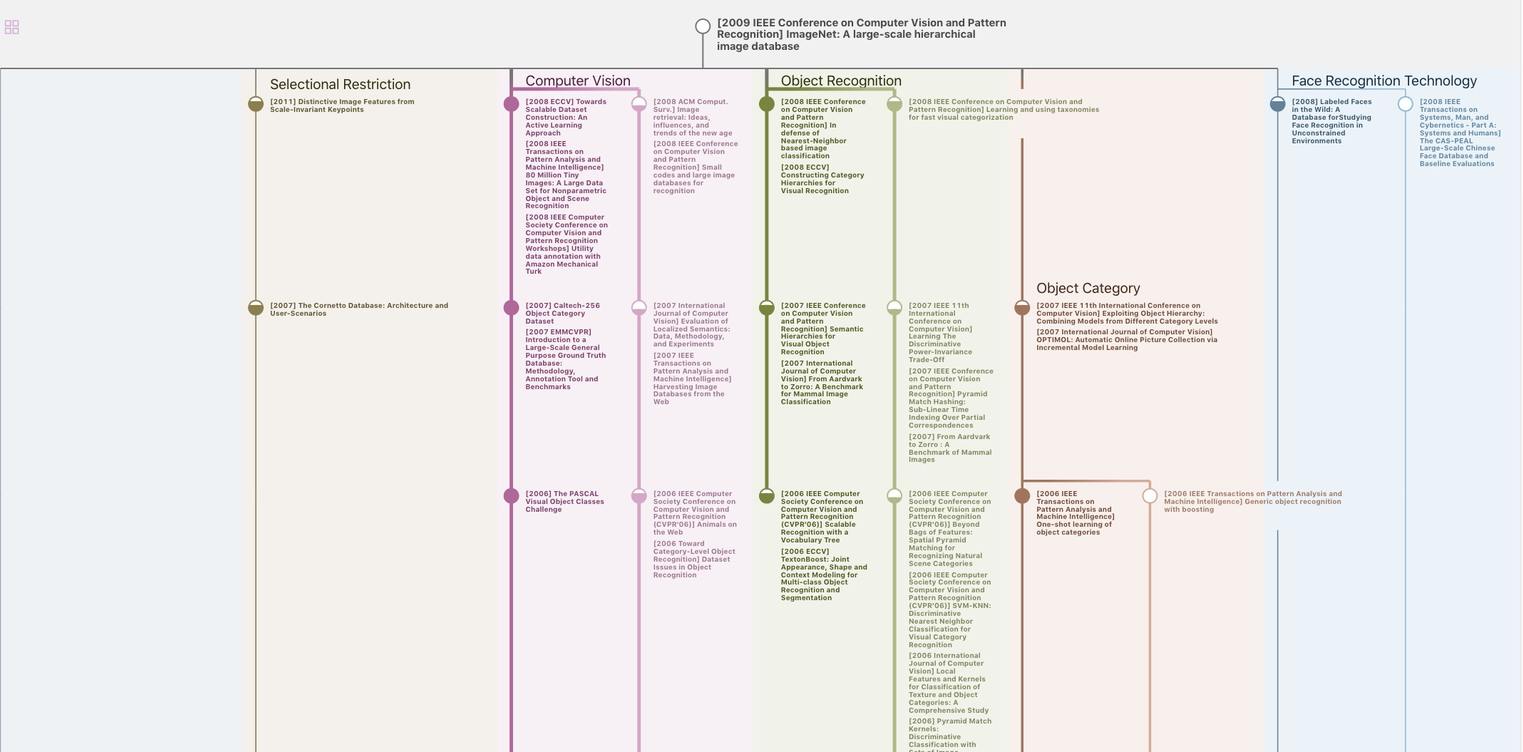
生成溯源树,研究论文发展脉络
Chat Paper
正在生成论文摘要