Conceptual structure coheres in human cognition but not in large language models.
EMNLP 2023(2023)
摘要
Neural network models of language have long been used as a tool for developing hypotheses about conceptual representation in the mind and brain. For many years, such use involved extracting vector-space representations of words and using distances among these to predict or understand human behavior in various semantic tasks. In contemporary language models, however, it is possible to interrogate the latent structure of conceptual representations using methods nearly identical to those commonly used with human participants. The current work uses three common techniques borrowed from cognitive psychology to estimate and compare lexical-semantic structure in both humans and a well-known large language model, the DaVinci variant of GPT-3. In humans, we show that conceptual structure is robust to differences in culture, language, and method of estimation. Structures estimated from the LLM behavior, while individually fairly consistent with those estimated from human behavior, depend much more upon the particular task used to generate behavior responses–responses generated by the very same model in the three tasks yield estimates of conceptual structure that cohere less with one another than do human structure estimates. The results suggest one important way that knowledge inhering in contemporary LLMs can differ from human cognition.
更多查看译文
关键词
large language models,conceptual structure,behavioral estimates,tasks
AI 理解论文
溯源树
样例
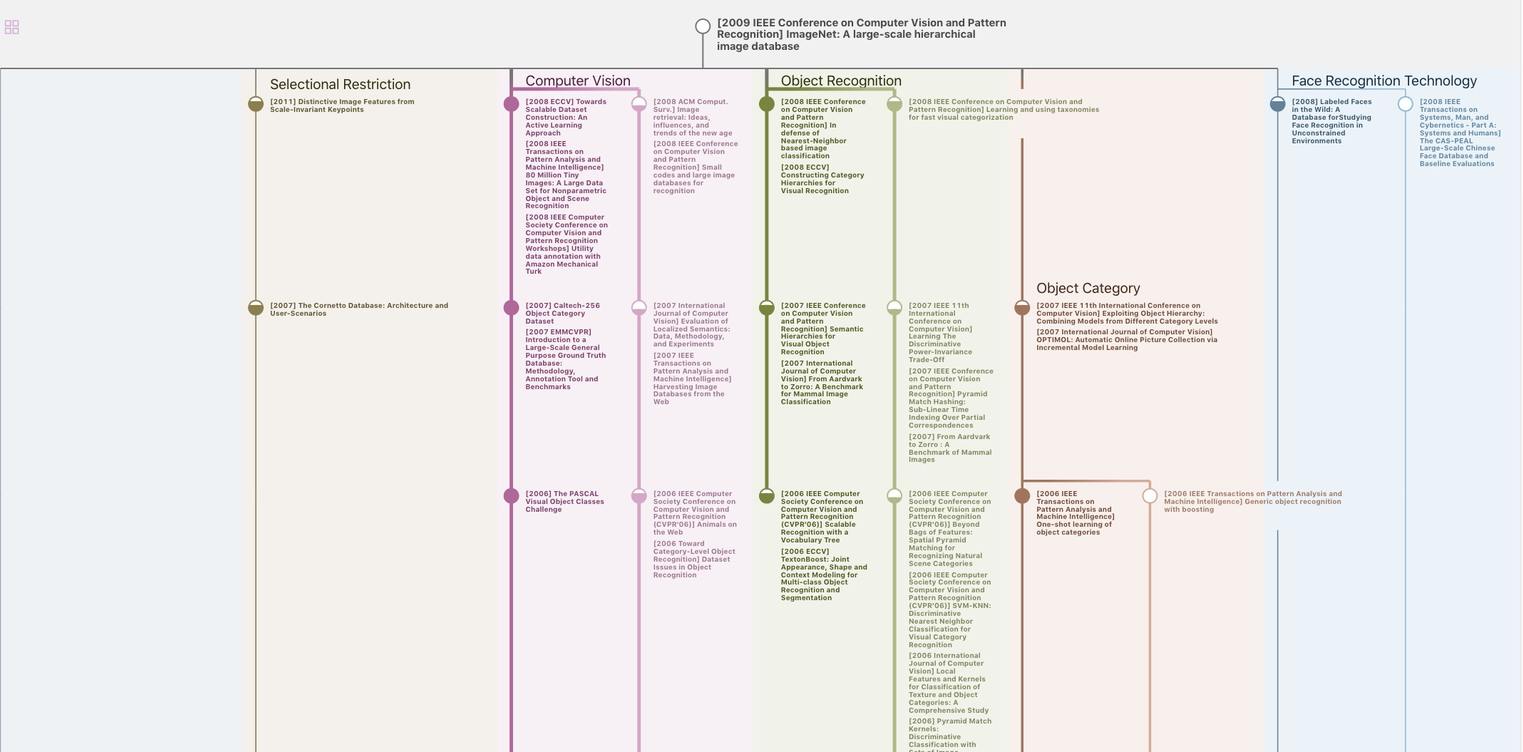
生成溯源树,研究论文发展脉络
Chat Paper
正在生成论文摘要