Comparing Prompt-Based and Standard Fine-Tuning for Urdu Text Classification.
EMNLP 2023(2023)
摘要
Recent advancements in natural language processing have demonstrated the efficacy of pre-trained language models for various downstream tasks through prompt-based fine-tuning. In contrast to standard fine-tuning, which relies solely on labeled examples, prompt-based fine-tuning combines a few labeled examples (few shot) with guidance through prompts tailored for the specific language and task. For low-resource languages, where labeled examples are limited, prompt-based fine-tuning appears to be a promising alternative. In this paper, we compare prompt-based and standard fine-tuning for the popular task of text classification in Urdu and Roman Urdu languages. We conduct experiments using five datasets, covering different domains, and pre-trained multilingual transformers. The results reveal that significant improvement of up to 13% in accuracy is achieved by prompt-based fine-tuning over standard fine-tuning approaches. This suggests the potential of prompt-based fine-tuning as a valuable approach for low-resource languages with limited labeled data.
更多查看译文
AI 理解论文
溯源树
样例
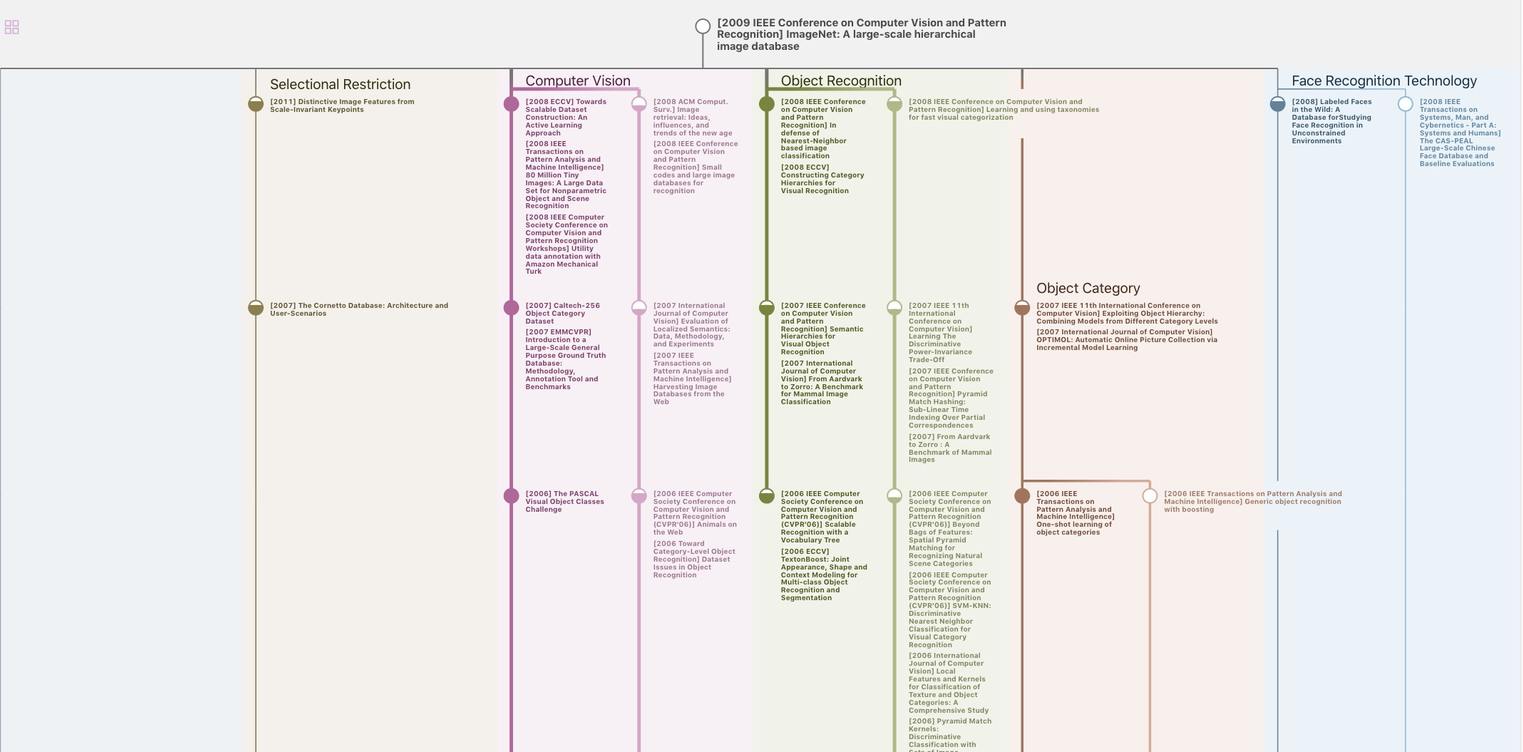
生成溯源树,研究论文发展脉络
Chat Paper
正在生成论文摘要