Hybrid Inverted Index Is a Robust Accelerator for Dense Retrieval.
EMNLP 2023(2023)
摘要
Inverted file structure is a common technique for accelerating dense retrieval.
It clusters documents based on their embeddings; during searching, it probes nearby clusters w.r.t. an input query and only evaluates documents within them by subsequent codecs, thus avoiding the expensive cost from exhaustive traversal.
However, the clustering is always lossy, which results in the miss of relevant documents in the probed clusters and hence degrades retrieval quality.
In contrast, lexical matching, such as overlaps of salient terms, tend to be strong features for identifying relevant documents.
In this work, we present the Hybrid Inverted Index (HI$^2$), where the embedding clusters and salient terms work collaboratively to accelerate dense retrieval.
To make best of both effectiveness and efficiency, we devise a cluster selector and a term selector, to construct compact inverted lists and efficiently searching through them.
Moreover, we leverage simple unsupervised algorithms as well as end-to-end knowledge distillation to learn these two modules, with the latter further boosting the effectiveness.
Based on comprehensive experiments on popular retrieval benchmarks, we verify that clusters and terms indeed complement each other, enabling HI$^2$ to achieve lossless retrieval quality with competitive efficiency across a variety of index settings.
更多查看译文
关键词
retrieval,bi-phase,time-efficient,ad-hoc
AI 理解论文
溯源树
样例
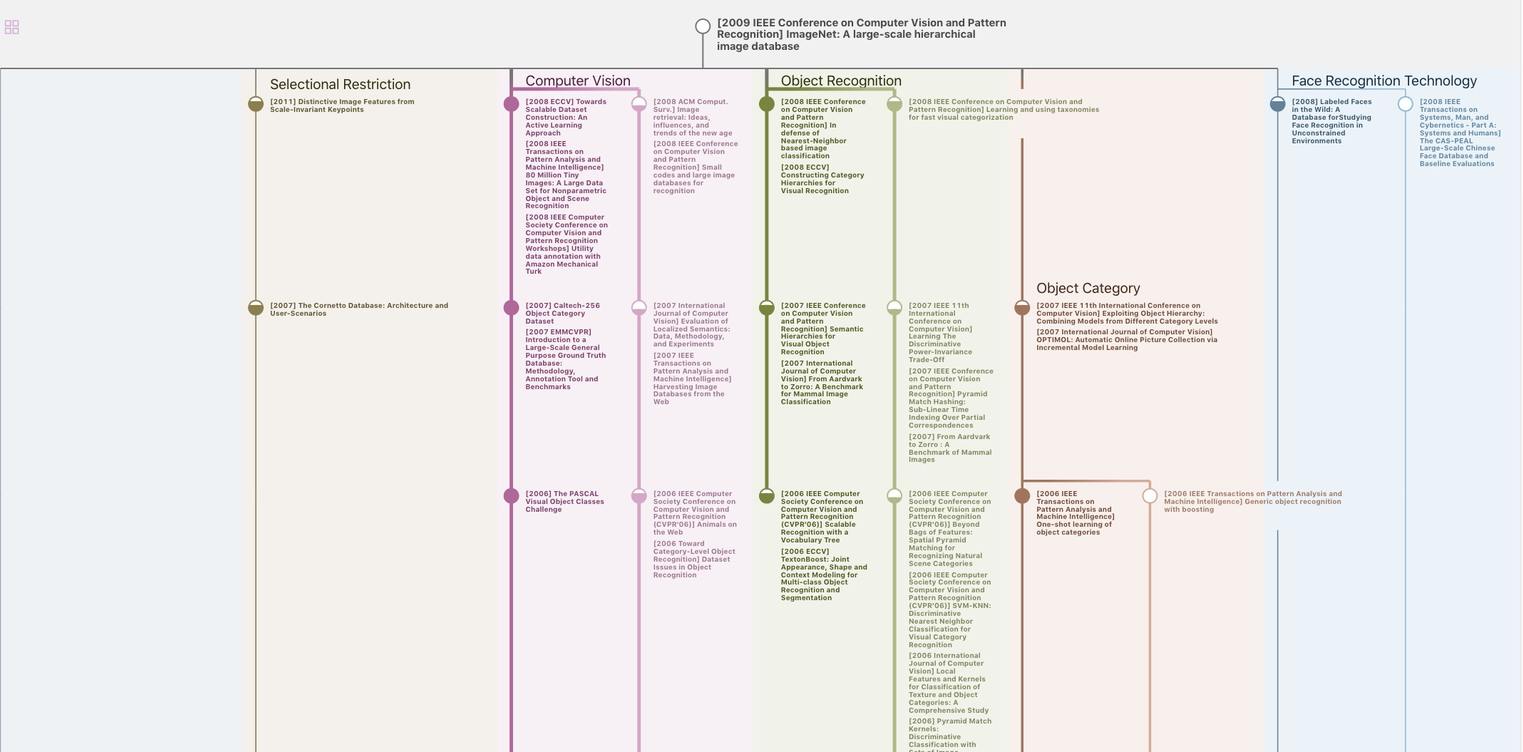
生成溯源树,研究论文发展脉络
Chat Paper
正在生成论文摘要