Task-Adaptive Tokenization: Enhancing Long-Form Text Generation Efficacy in Mental Health and Beyond.
EMNLP 2023(2023)
Abstract
We propose task-adaptive tokenization\footnote{Our work will be publicly available upon acceptance.} as a way to adapt the generation pipeline to the specifics of a downstream task and enhance long-form generation in mental health. Inspired by insights from cognitive science, our task-adaptive tokenizer samples variable segmentations from multiple outcomes, with sampling probabilities optimized based on task-specific data. We introduce a strategy for building a specialized vocabulary and introduce a vocabulary merging protocol that allows for the integration of task-specific tokens into the pre-trained model's tokenization step.
Through extensive experiments on psychological question-answering tasks in both Chinese and English, we find that our task-adaptive tokenization approach brings a significant improvement in generation performance while using up to 60\% fewer tokens.
Preliminary experiments point to promising results when using our tokenization approach with very large language models.
MoreTranslated text
AI Read Science
Must-Reading Tree
Example
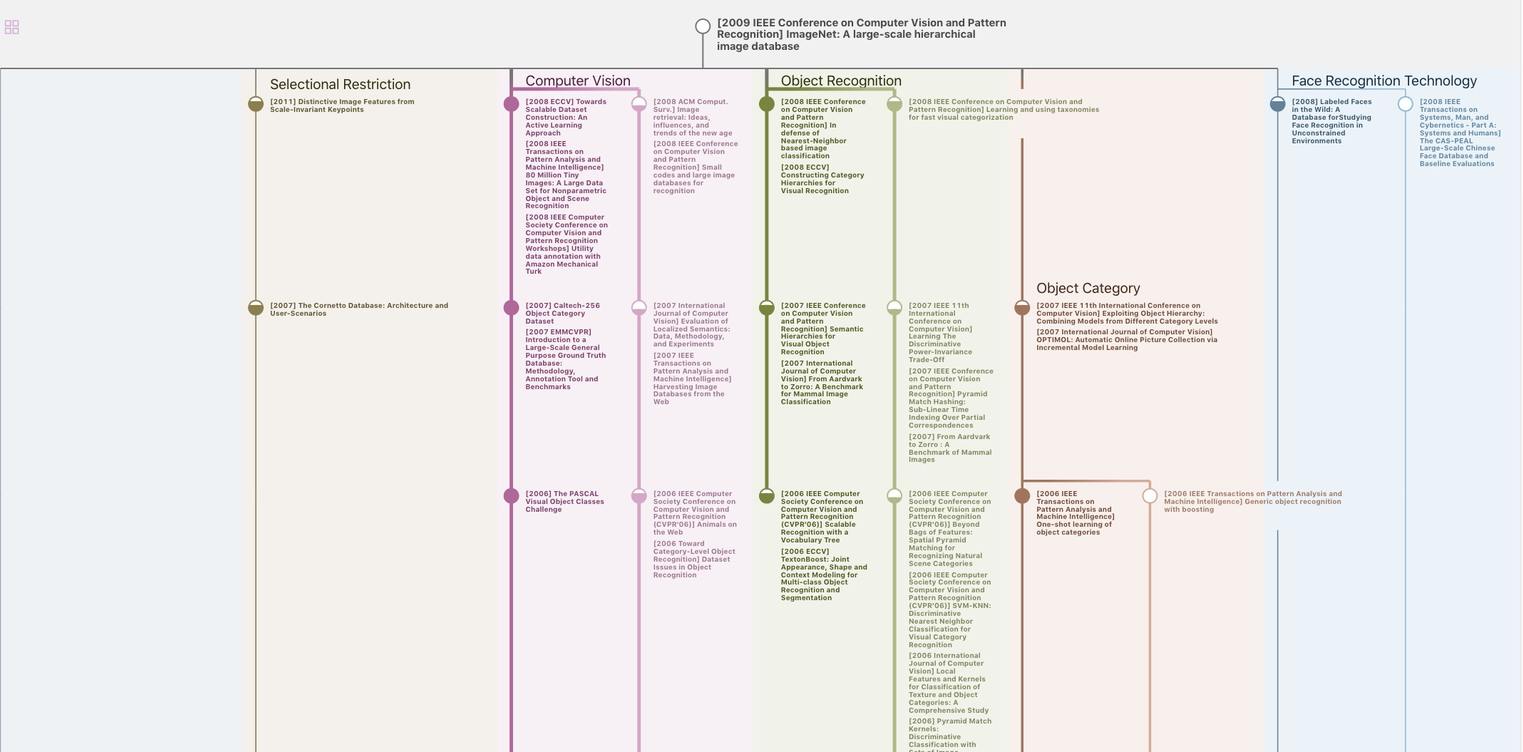
Generate MRT to find the research sequence of this paper
Chat Paper
Summary is being generated by the instructions you defined