Pre-training Graph Transformer with Multimodal Side Information for Recommendation
arXiv (Cornell University)(2020)
摘要
Side information of items, e.g., images and text description, has shown to be effective in contributing to accurate recommendations. Inspired by the recent success of pre-training models on natural language and images, we propose a pre-training strategy to learn item representations by considering both item side information and their relationships. We relate items by common user activities, e.g., co-purchase, and construct a homogeneous item graph. This graph provides a unified view of item relations and their associated side information in multimodality. We develop a novel sampling algorithm named MCNSampling to select contextual neighbors for each item. The proposed Pre-trained Multimodal Graph Transformer (PMGT) learns item representations with two objectives: 1) graph structure reconstruction, and 2) masked node feature reconstruction. Experimental results on real datasets demonstrate that the proposed PMGT model effectively exploits the multimodality side information to achieve better accuracies in downstream tasks including item recommendation, item classification, and click-through ratio prediction. We also report a case study of testing the proposed PMGT model in an online setting with 600 thousand users.
更多查看译文
关键词
multimodal side information,graph,pre-training
AI 理解论文
溯源树
样例
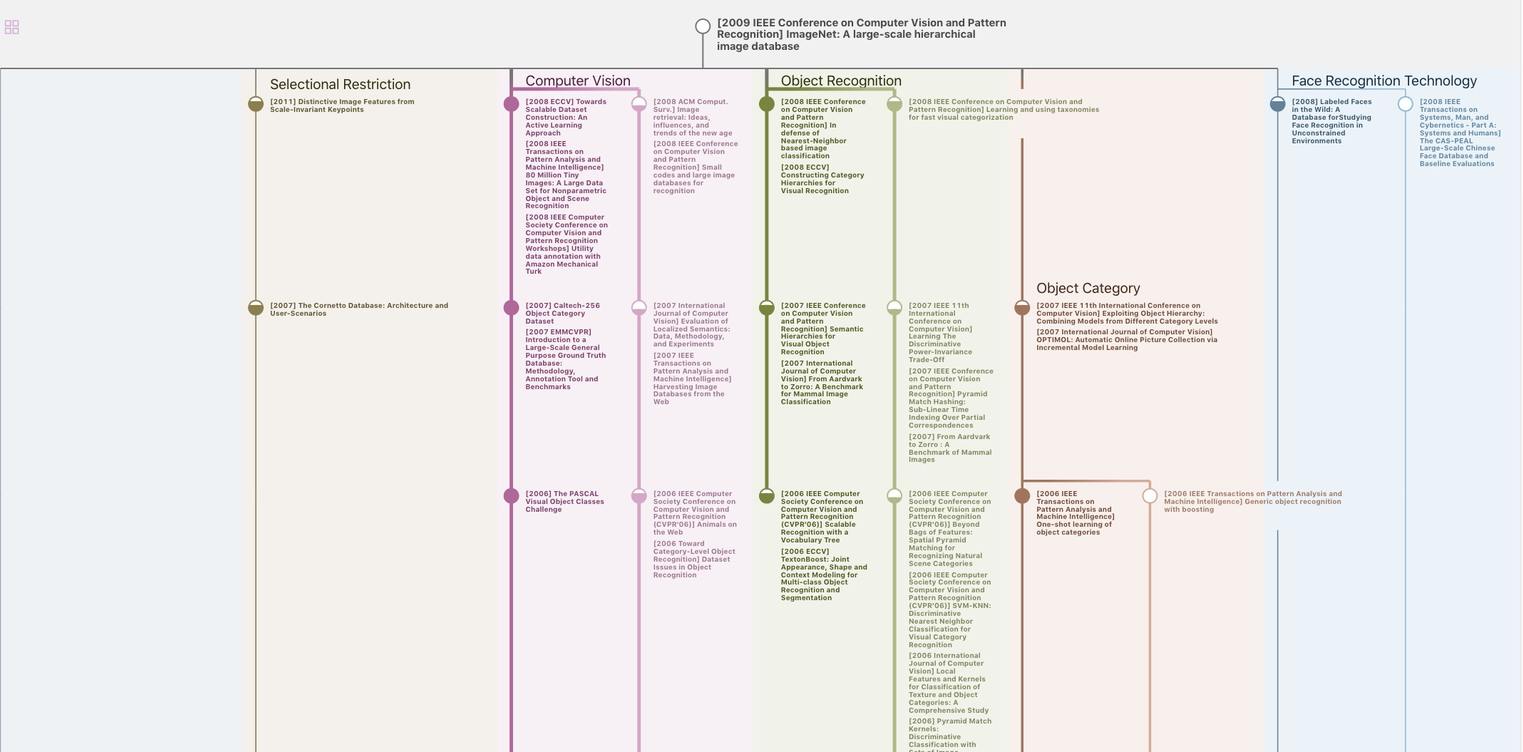
生成溯源树,研究论文发展脉络
Chat Paper
正在生成论文摘要