Machine Learning Identifies Complicated Sepsis Course and Subsequent Mortality Based on 20 Genes in Peripheral Blood Immune Cells at 24 Hours post ICU admission
bioRxiv (Cold Spring Harbor Laboratory)(2020)
摘要
Abstract A complicated clinical course for critically ill patients admitted to the ICU usually includes multiorgan dysfunction and subsequent death. Owning to the heterogeneity, complexity, and unpredictability of the disease progression, patient care is challenging. Identifying the predictors of complicated courses and subsequent mortality at the early stages of the disease and recognizing the trajectory of the disease from the vast array of longitudinal quantitative clinical data is difficult. Therefore, we attempted to identify novel early biomarkers and train the artificial intelligence systems to recognize the disease trajectories and subsequent clinical outcomes. Using the gene expression profile of peripheral blood cells obtained within 24 hours of PICU admission and numerous clinical data from 228 septic patients from pediatric ICU, we identified 20 differentially expressed genes that were predictive of complicated course outcomes and developed a new machine learning model. After 5-fold cross-validation with ten iterations, the overall mean area under the curve reached 0.82. Using the same set of genes, we further achieved an overall area under the curve of 0.72 when tested on an external validation set. This model was highly effective in identifying the clinical trajectories of the patients and mortality. Artificial intelligence systems identified eight out of twenty novel genetic markers SDC4, CLEC5A, TCN1, MS4A3, HCAR3, OLAH, PLCB1 and NLRP1 that help to predict sepsis severity or mortality. The discovery of eight novel genetic biomarkers related to the overactive innate immune system and neutrophils functions, and a new predictive machine learning method provides options to effectively recognize sepsis trajectories, modify real-time treatment options, improve prognosis, and patient survival. Research in Context Evidence before this study Transcriptomic biomarkers have long been explored as potential means of earlier disease endotyping. Much of the existing literature has however focused on mortality and discrete outcomes. Additionally, much of prior work in this area has been developed on statistical methods, while recent means of selecting features have not been sufficiently explored. Added value of this study In this study, we developed a robust machine learning based model for identifying novel biomarkers of complicated disease courses. We found 20 highly stable genes that predict disease complexity with an average derivation AUROC of 0.82 and validation AUROC of 0.72 within critically ill children, using peripheral blood collected within 24 hrs of ICU admission. Implications of all the available evidence Earlier identification of disease complexity can inform care management and targeted therapy. Therefore, the 20 gene candidates identified by our rigorous approach, can be used to identify, early in their ICU stay, patients who may ultimately develop significant organ dysfunction and complex care management.
更多查看译文
关键词
complicated sepsis course,peripheral blood immune cells,hours post icu admission
AI 理解论文
溯源树
样例
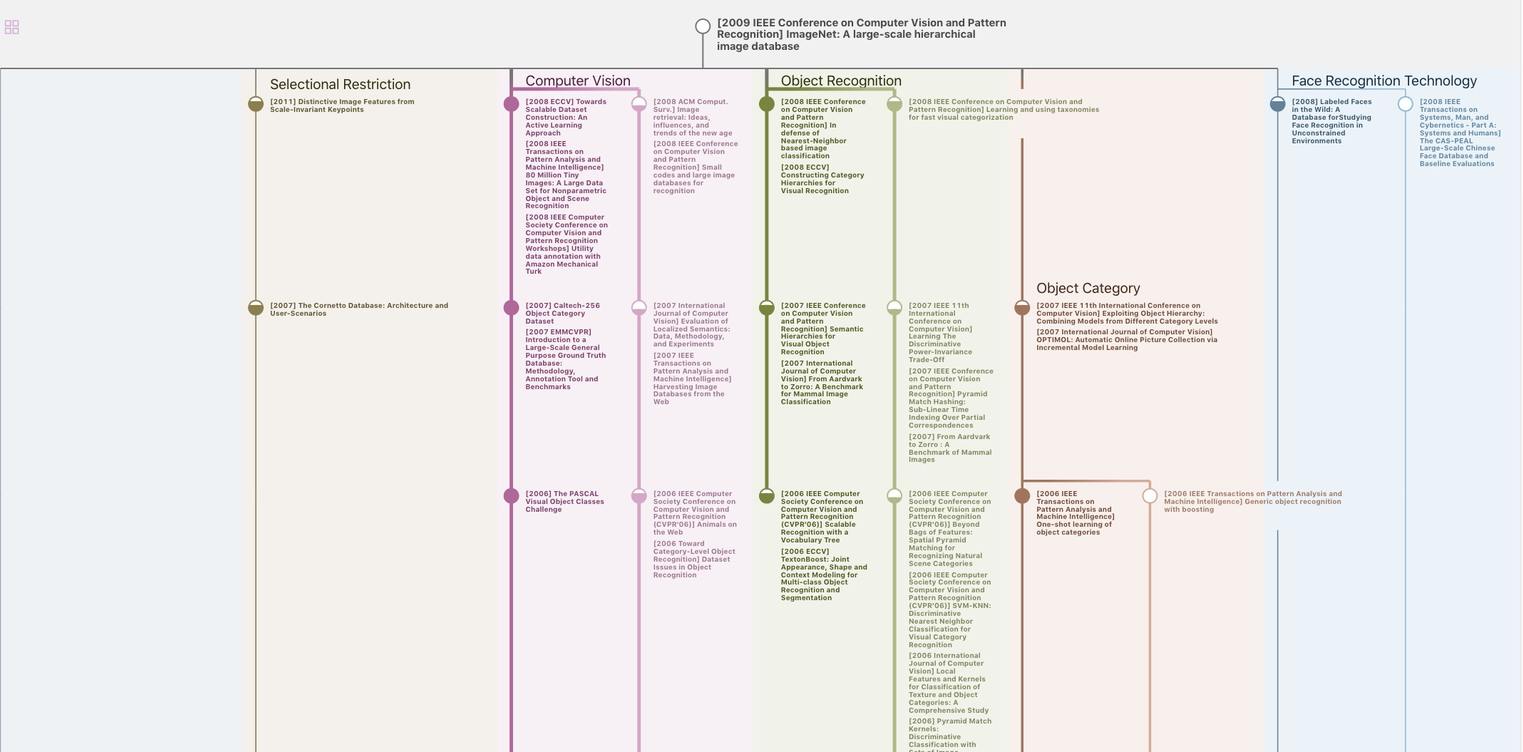
生成溯源树,研究论文发展脉络
Chat Paper
正在生成论文摘要