Prob2Vec: Mathematical Semantic Embedding for Problem Retrieval in Adaptive Tutoring
arXiv (Cornell University)(2020)
摘要
We propose a new application of embedding techniques for problem retrieval in adaptive tutoring. The objective is to retrieve problems whose mathematical concepts are similar. There are two challenges: First, like sentences, problems helpful to tutoring are never exactly the same in terms of the underlying concepts. Instead, good problems mix concepts in innovative ways, while still displaying continuity in their relationships. Second, it is difficult for humans to determine a similarity score that is consistent across a large enough training set. We propose a hierarchical problem embedding algorithm, called Prob2Vec, that consists of abstraction and embedding steps. Prob2Vec achieves 96.88\% accuracy on a problem similarity test, in contrast to 75\% from directly applying state-of-the-art sentence embedding methods. It is interesting that Prob2Vec is able to distinguish very fine-grained differences among problems, an ability humans need time and effort to acquire. In addition, the sub-problem of concept labeling with imbalanced training data set is interesting in its own right. It is a multi-label problem suffering from dimensionality explosion, which we propose ways to ameliorate. We propose the novel negative pre-training algorithm that dramatically reduces false negative and positive ratios for classification, using an imbalanced training data set.
更多查看译文
关键词
tutoring,problem retrieval,mathematical semantic
AI 理解论文
溯源树
样例
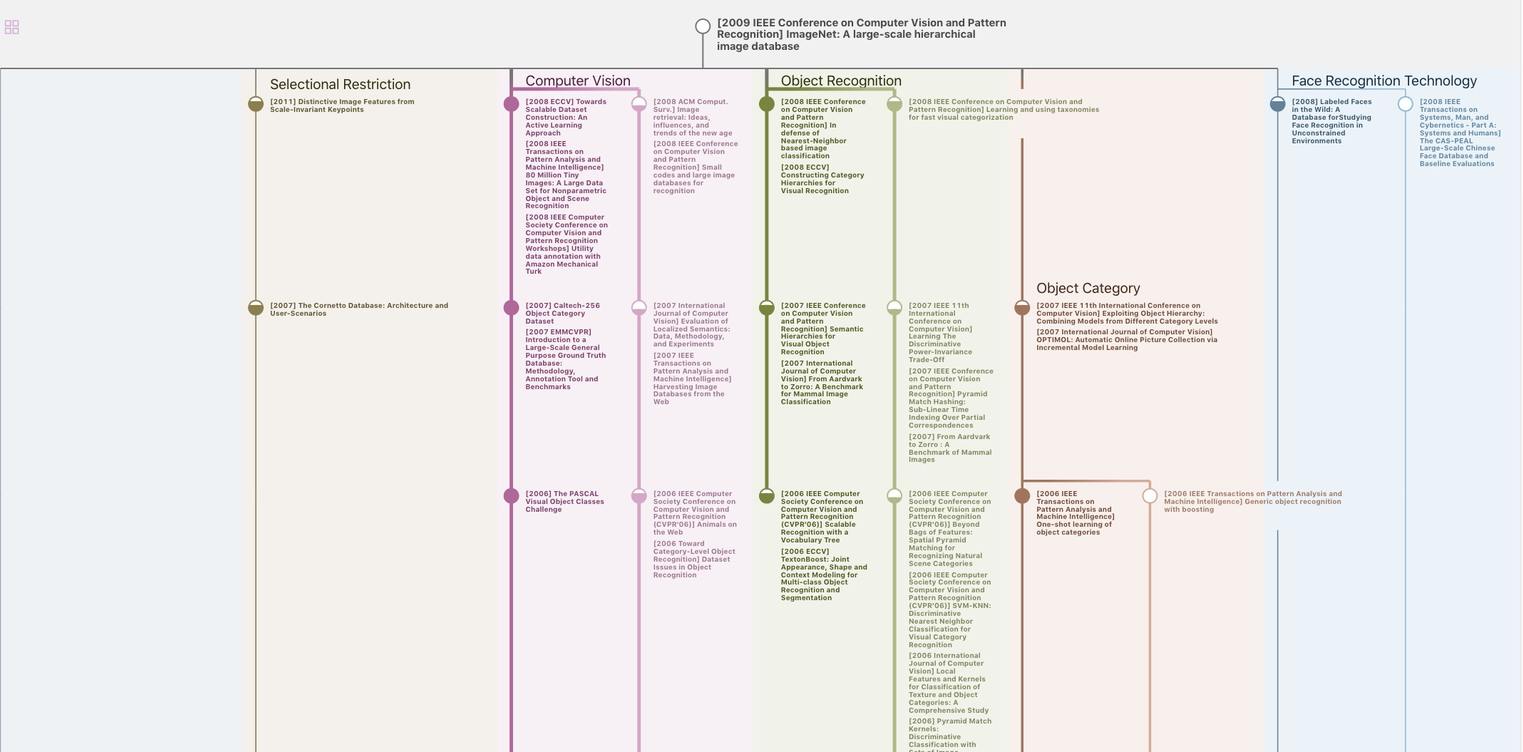
生成溯源树,研究论文发展脉络
Chat Paper
正在生成论文摘要