Contextualized Spoken Word Representations from Convolutional Autoencoders.
arXiv (Cornell University)(2020)
摘要
A lot of work has been done to build text-based language models for performing different NLP tasks, but not much research has been done in the case of audio-based language models. This paper proposes a Convolutional Autoencoder based neural architecture to model syntactically and semantically adequate contextualized representations of varying length spoken words. The use of such representations can not only lead to great advances in the audio-based NLP tasks but can also curtail the loss of information like tone, expression, accent, etc while converting speech to text to perform these tasks. The performance of the proposed model is validated by (1) examining the generated vector space, and (2) evaluating its performance on three benchmark datasets for measuring word similarities, against existing widely used text-based language models that are trained on the transcriptions. The proposed model was able to demonstrate its robustness when compared to the other two language-based models.
更多查看译文
关键词
contextualized spoken word representations,convolutional autoencoders
AI 理解论文
溯源树
样例
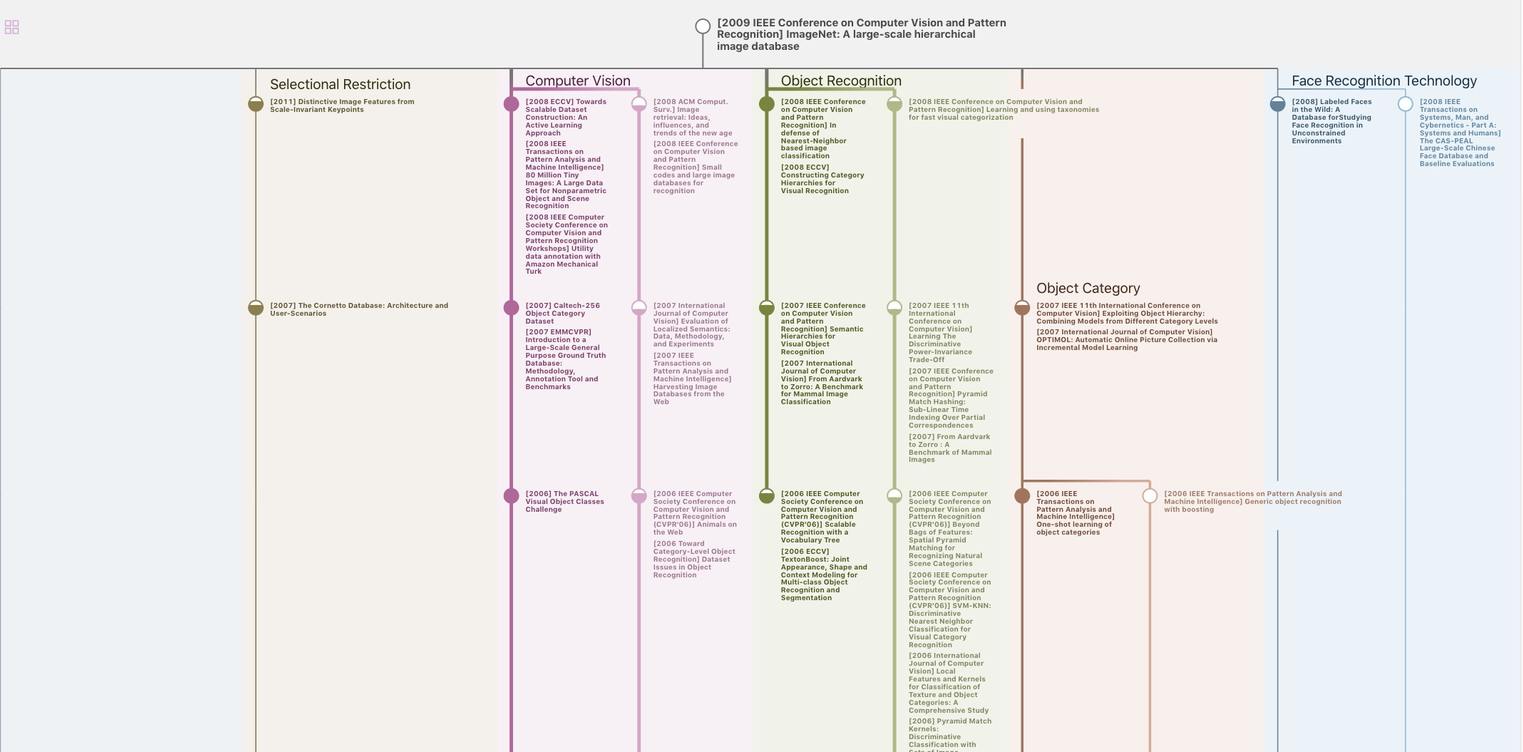
生成溯源树,研究论文发展脉络
Chat Paper
正在生成论文摘要