Interactive Reweighting for Mitigating Label Quality Issues
IEEE TRANSACTIONS ON VISUALIZATION AND COMPUTER GRAPHICS(2024)
摘要
Label quality issues, such as noisy labels and imbalanced class distributions, have negative effects on model performance. Automatic reweighting methods identify problematic samples with label quality issues by recognizing their negative effects on validation samples and assigning lower weights to them. However, these methods fail to achieve satisfactory performance when the validation samples are of low quality. To tackle this, we develop Reweighter, a visual analysis tool for sample reweighting. The reweighting relationships between validation samples and training samples are modeled as a bipartite graph. Based on this graph, a validation sample improvement method is developed to improve the quality of validation samples. Since the automatic improvement may not always be perfect, a co-cluster-based bipartite graph visualization is developed to illustrate the reweighting relationships and support the interactive adjustments to validation samples and reweighting results. The adjustments are converted into the constraints of the validation sample improvement method to further improve validation samples. We demonstrate the effectiveness of Reweighter in improving reweighting results through quantitative evaluation and two case studies.
更多查看译文
关键词
Training,Training data,Noise measurement,Annotations,Bipartite graph,Visualization,Data visualization,Bipartite graph visualization,sample reweighting,training data quality
AI 理解论文
溯源树
样例
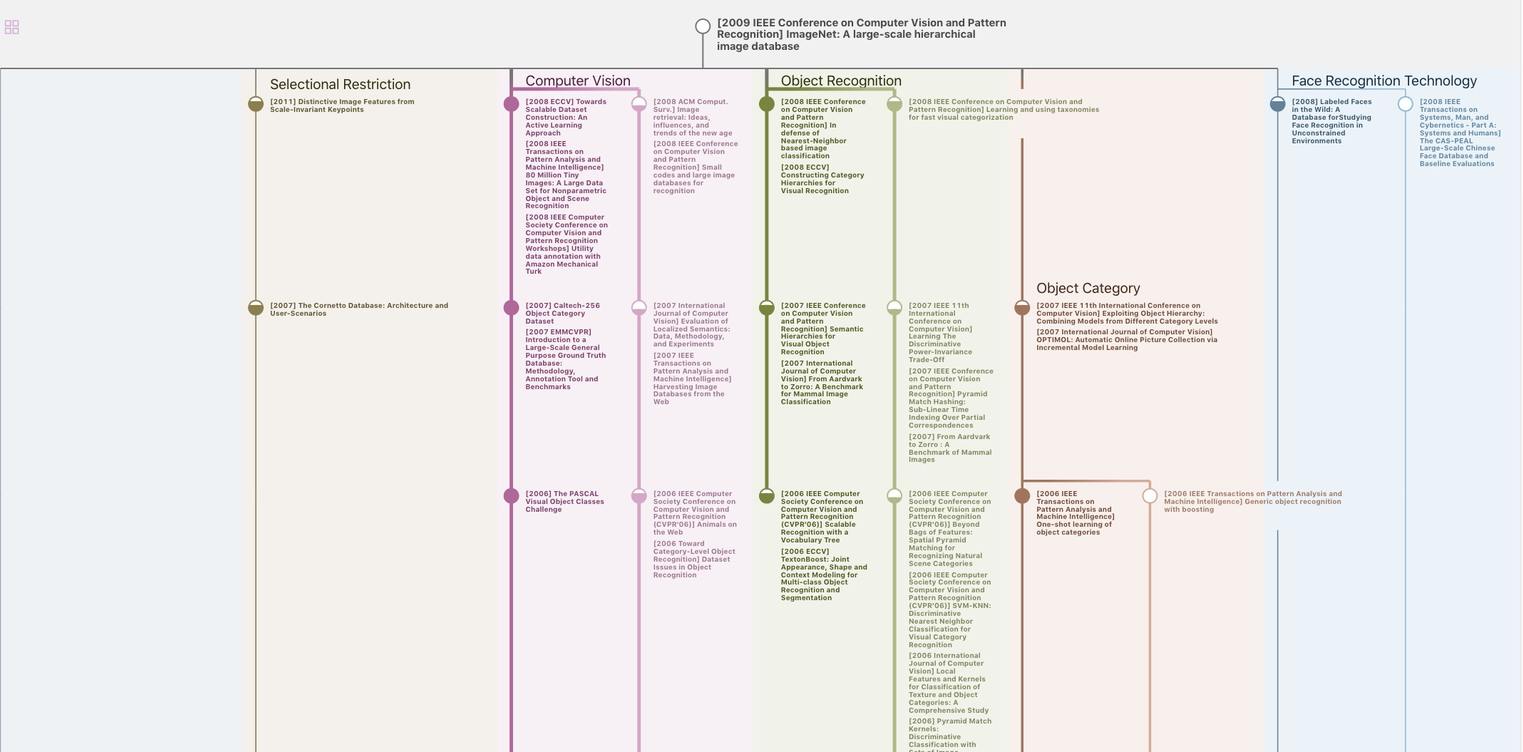
生成溯源树,研究论文发展脉络
Chat Paper
正在生成论文摘要