Modeling Both Intra- and Inter-Modality Uncertainty for Multimodal Fake News Detection
IEEE TRANSACTIONS ON MULTIMEDIA(2023)
摘要
Multimodal fake news detection has obtained increasing attention recently. Existing works generally encode multimodal contents into a deterministic point in semantic subspaces, and then fuse multimodal features by simple concatenation or attention mechanisms. However, most methods suffer from adapting to noisy multimodal contents since they neglect the robustness of modality-specific features. Besides, as different modalities usually have varying confidence levels, previous attention-based fusion models that learn modality-independent weights based on the input data feature, would limit the optimal integration of multimodal contents. To alleviate the above issues, we propose novel Multimodal Uncertainty Learning Network (MM-ULN) to enhance multimodal fake news detection by modeling both intra- and inter-modality uncertainty. Specifically, we incorporate a novel intra-modality uncertainty learning (EUL) module to better understand noisy multimodal contents. EULs provide feature regularization in a variational way, successfully alleviating the effects of data uncertainty within modalities. We design a new variational attention fusion (VAF) module to adaptively fuse multimodal contents with modality-dependent weights. The VAF module consider the relative confidence between modalities and enables to explore complementary properties for detection. Extensive experiments on two benchmark datasets demonstrate the effectiveness and superiority of MM-ULN on multimodal fake news detection.
更多查看译文
关键词
Fake news detection,uncertainty learning,multimodal fusion,vartional autoencoder,attention,social network analysis
AI 理解论文
溯源树
样例
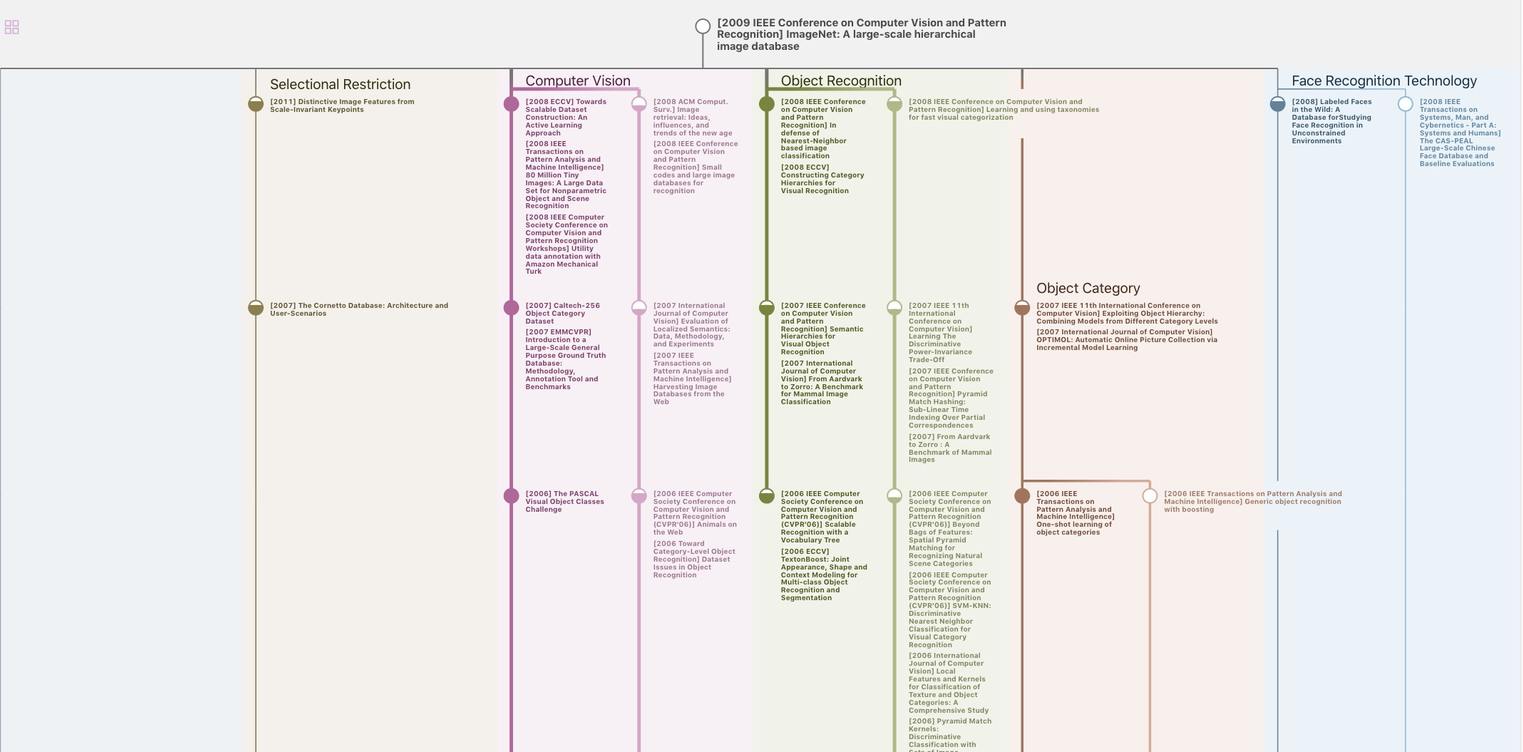
生成溯源树,研究论文发展脉络
Chat Paper
正在生成论文摘要