Measuring Underwater Image Quality with Depthwise-separable Convolutions and Global-sparse Attention
2023 IEEE 25TH INTERNATIONAL WORKSHOP ON MULTIMEDIA SIGNAL PROCESSING, MMSP(2023)
摘要
Underwater Image Quality Assessment (UIQA) poses specific challenges due to blur, dispersion of light, and color garbling caused by water turbulence. Images captured by Autonomous Underwater Vehicles (AVS) correlate poorly along their RGB channels, as blue and green channel components dominate. We propose a novel lightweight no-reference (NR) UIQA architecture based on depthwise-separable convolutions and global sparse attention that surpasses the existing deep learning architectures in terms of performance as well as parameter count and Multiply-Accumulate operations (MACs). Proposed model gives 3.64% increase in SRCC and 6.11% increase in PLCC in comparison to state-of-the-art for UEQAB dataset. Our work also illustrates the effectiveness of depthwise separable convolutions for underwater image quality assessment. Our empirical analysis confirms less redundancy among the channels of a feature map when depthwise separable convolutions are used compared to standard convolutions by increasing representational efficiency.
更多查看译文
关键词
Underwater Image Quality Assessment (UIQA),Global Sparse Attention,Depthwise-Separable Convolution,PLCC Loss,Rank Loss
AI 理解论文
溯源树
样例
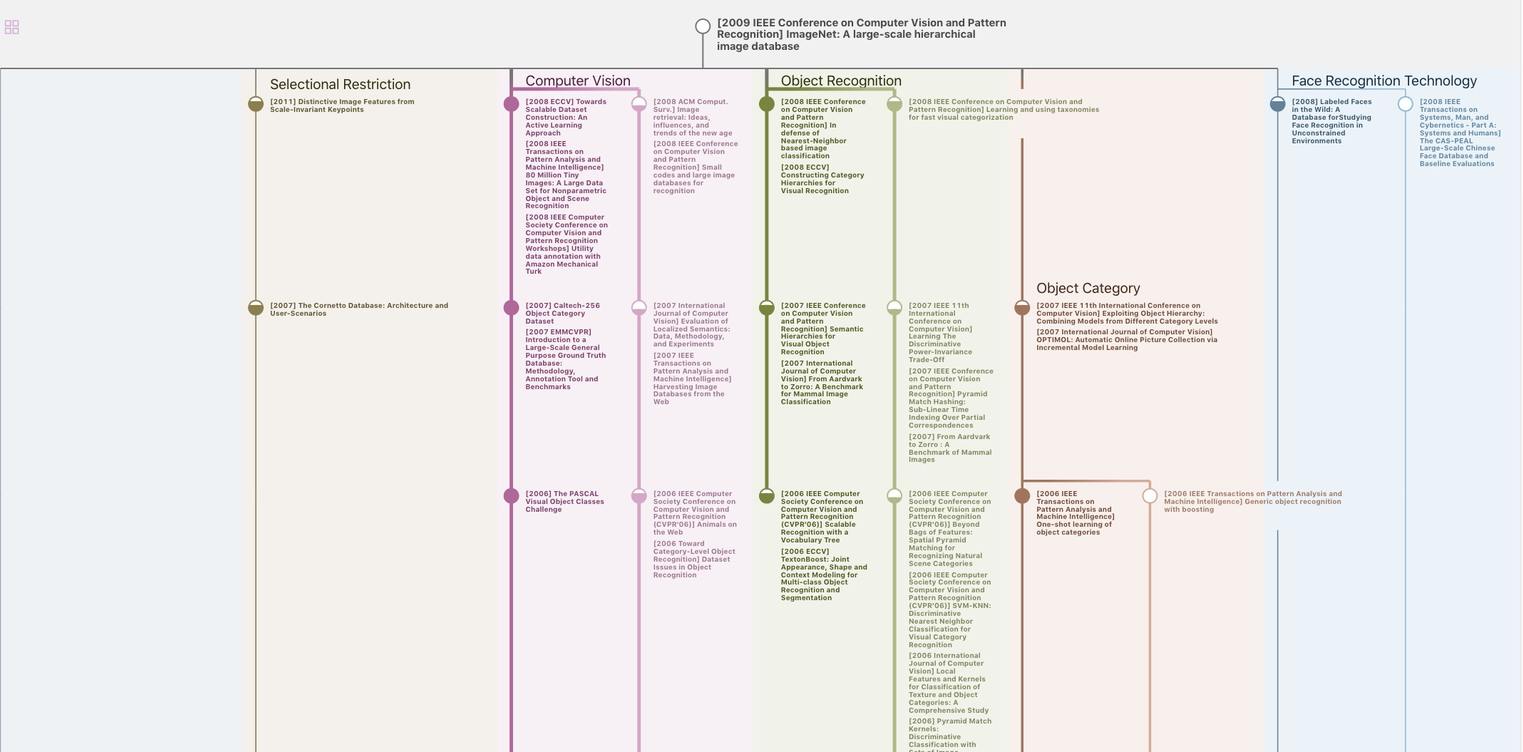
生成溯源树,研究论文发展脉络
Chat Paper
正在生成论文摘要