Novel Data-Driven Deep Learning Assisted CVA for Ironmaking System Prediction and Control
IEEE TRANSACTIONS ON CIRCUITS AND SYSTEMS II-EXPRESS BRIEFS(2023)
摘要
Effective control of blast furnace ironmaking system (IS) is crucial to maintain the metallurgical industry in smooth operation. Also, the quality of molten iron (MIQ) can be assessed using indices of phosphorus [P], sulfur [S], and silicon [Si] contents, which provide insight into the current state of the molten iron and internal operations of the blast furnace. In this brief, we propose a novel deep learning assisted canonical variate analysis (DLaCVA) method for modeling and predicting MIQ and optimizing IS control. Due to the highly complex physical and chemical reactions within IS, we build a nonlinear state-space model and estimate its state, utilizing DLaCVA. Moreover, we theoretically analyze of how deep learning can aid in state-space modeling, such as deriving the corresponding optimization objective and learning gradient, laying the basis for exploring deep learning in system identification further. Ultimately, we devise a predictive control strategy based on a quadratic performance index to attain optimal MIQ control performance. Experiments utilizing genuine IS data display that DLaCVA outperforms other methods concerning modeling accuracy and control effectiveness.
更多查看译文
关键词
Ironmaking system (IS),deep learning,nonlinear state-space model,model predictive control
AI 理解论文
溯源树
样例
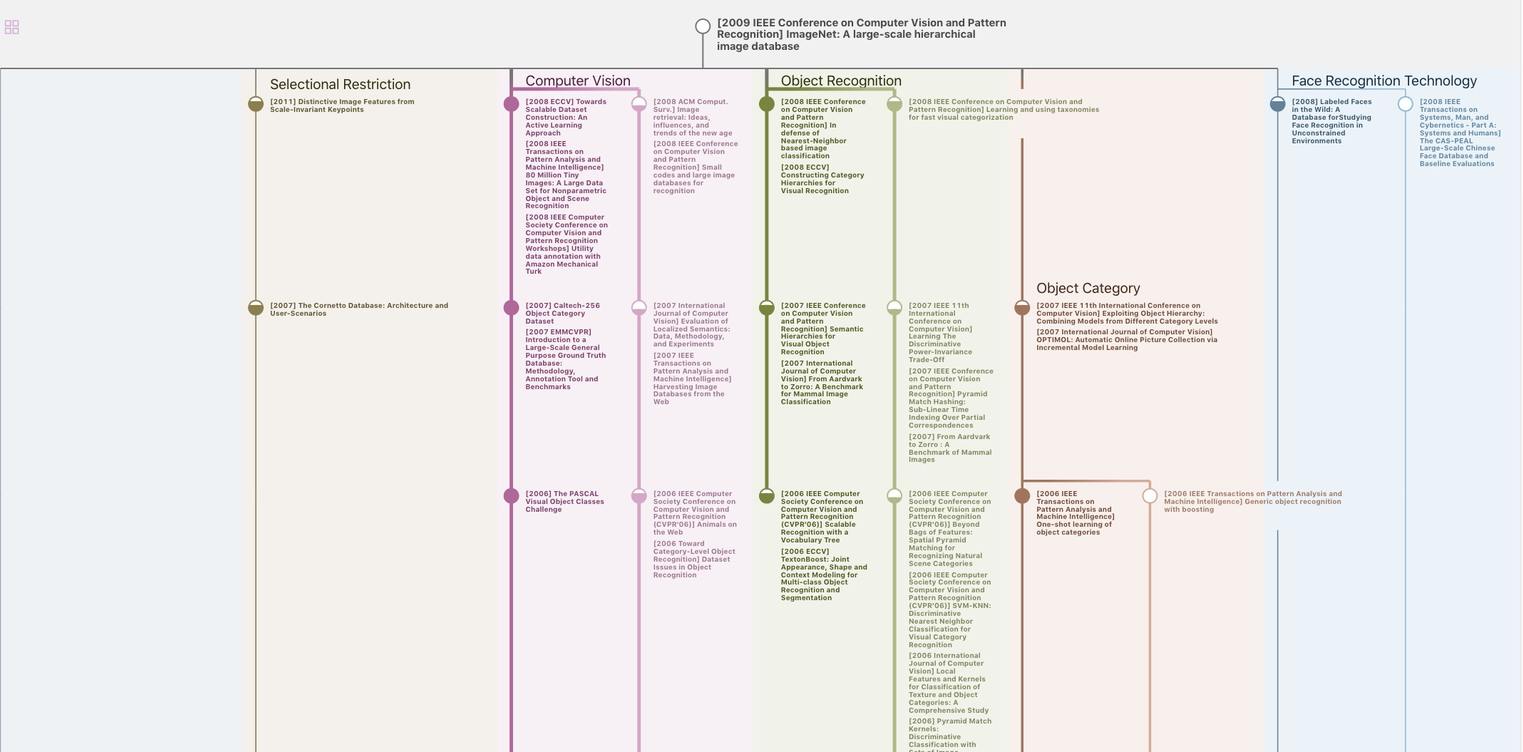
生成溯源树,研究论文发展脉络
Chat Paper
正在生成论文摘要