A Framework for Autonomic Computing for In Situ Imageomics
2023 IEEE INTERNATIONAL CONFERENCE ON AUTONOMIC COMPUTING AND SELF-ORGANIZING SYSTEMS, ACSOS(2023)
摘要
In situ imageomics is a new approach to study ecological, biological and evolutionary systems wherein large image and video data sets are captured in the wild and machine learning methods are used to infer biological traits of individual organisms, animal social groups, species, and even whole ecosystems. Monitoring biological traits over large spaces and long periods of time could enable new, data-driven approaches to wildlife conservation, biodiversity, and sustainable ecosystem management. However, to accurately infer biological traits, machine learning methods for images require voluminous and high quality data. Adaptive, data-driven approaches are hamstrung by the speed at which data can be captured and processed. Camera traps and unmanned aerial vehicles (UAVs) produce voluminous data, but lose track of individuals over large areas, fail to capture social dynamics, and waste time and storage on images with poor lighting and view angles. In this vision paper, we make the case for a research agenda for in situ imageomics that depends on significant advances in autonomic and self-aware computing. Precisely, we seek autonomous data collection that manages camera angles, aircraft positioning, conflicting actions for multiple traits of interest, energy availability, and cost factors. Given the tools to detect object and identify individuals, we propose a research challenge: Which optimization model should the data collection system employ to accurately identify, characterize, and draw inferences from biological traits while respecting a budget? Using zebra and giraffe behavioral data collected over three weeks at the Mpala Research Centre in Laikipia County, Kenya, we quantify the volume and quality of data collected using existing approaches. Our proposed autonomic navigation policy for in situ imageomics collection has an F1 score of 82% compared to an expert pilot, and provides greater safety and consistency, suggesting great potential for state-of-the-art autonomic approaches if they can be scaled up to fully address the problem.
更多查看译文
关键词
autonomous flight,UAVs,ecology,machine learning,computer vision,imageomics
AI 理解论文
溯源树
样例
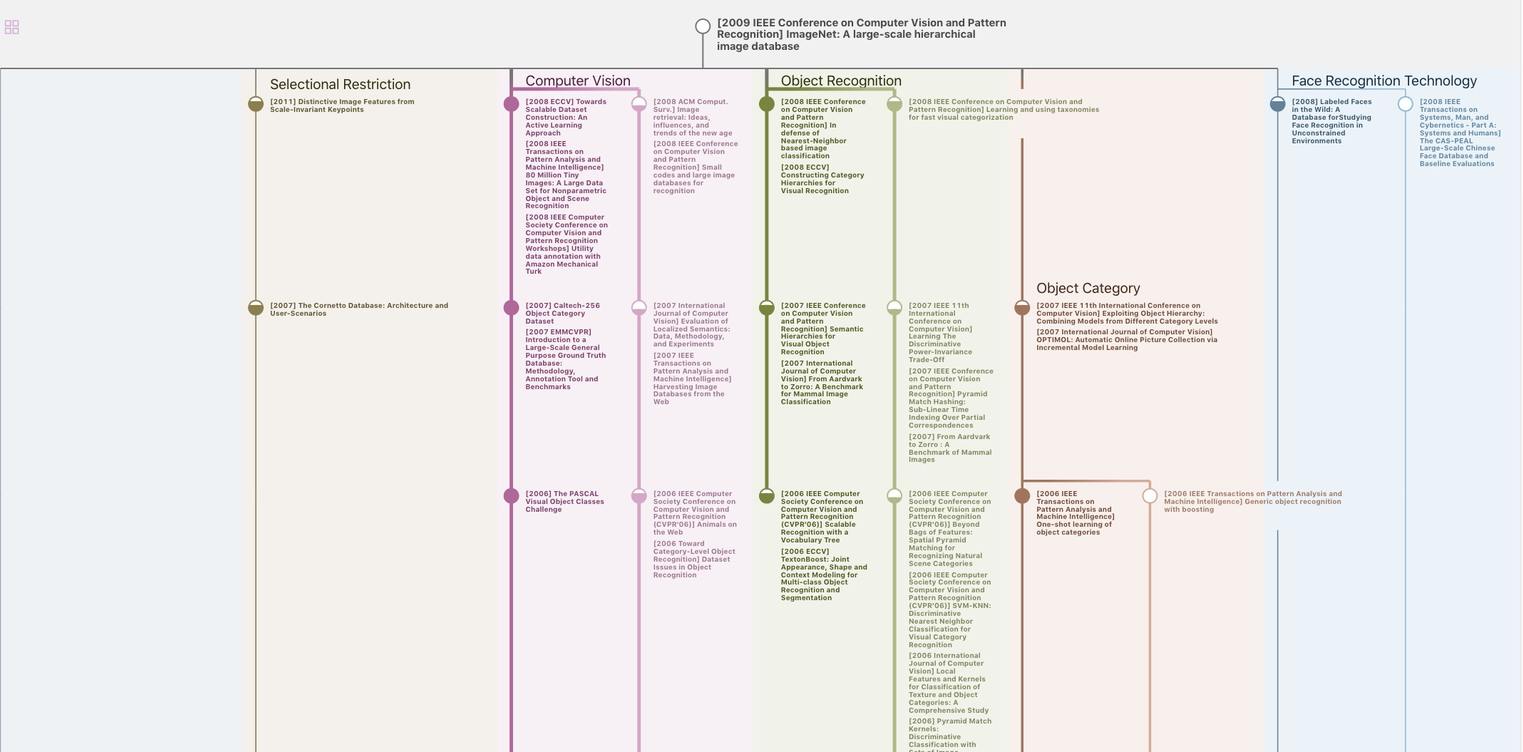
生成溯源树,研究论文发展脉络
Chat Paper
正在生成论文摘要