Efficient Sparse Bayesian Channel Estimation for Near-Field Ultra-Scale Massive MIMO Systems
IEEE WIRELESS COMMUNICATIONS LETTERS(2023)
Abstract
In this letter, we propose an efficient off-grid sparse Bayesian learning (SBL) algorithm to estimate the near-field (NF) channel in ultra-scale massive multiple-input multiple-output (MIMO) systems. We first exploit the sparsity of the NF channel in the polar domain and perform an off-grid sampling of angles and distances. Then, an effective SBL method is proposed for sparse channel recovery and off-grid refinement. The sampled grid points are set to adjustable parameters, which undergo iterative updates by resorting to an accurate block majorization-minimization (MM) algorithm and gradient calculation. The proposed method demonstrates efficient channel estimation capabilities even in the absence of prior knowledge of the noise variance, leveraging the sparse characteristics of the NF channel. Simulation results demonstrate that our approach significantly improves the sparsity and accuracy of the NF channel representation. Furthermore, it outperforms the existing state-of-the-art algorithms in terms of estimation performance while requiring less pilot overhead.
MoreTranslated text
Key words
Sparse Bayesian learning,near-field,ultra-scale,massive MIMO,channel estimation
AI Read Science
Must-Reading Tree
Example
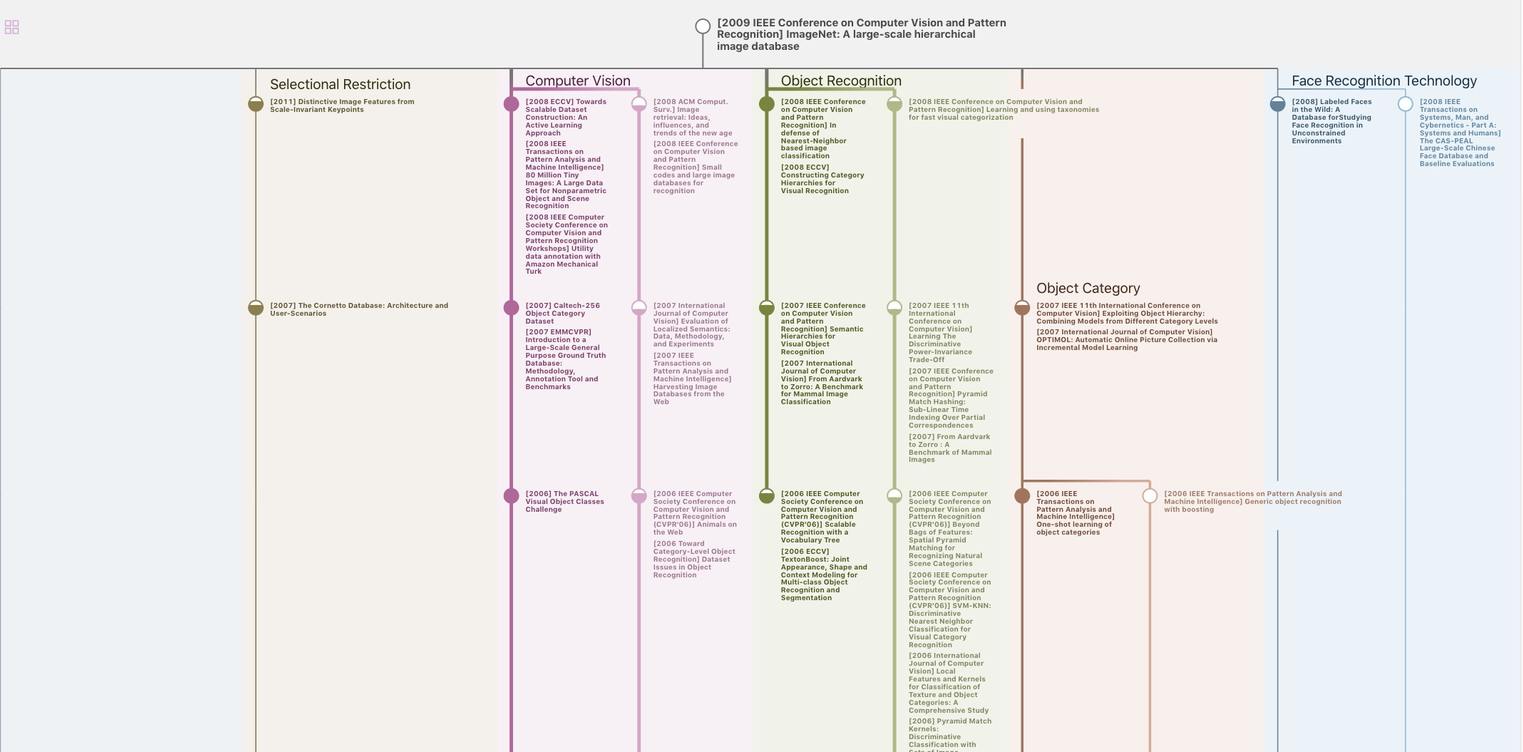
Generate MRT to find the research sequence of this paper
Chat Paper
Summary is being generated by the instructions you defined